Abstract
Background: The banking sector plays an important role in economic activity: it mobilises savings and channels them to productive sectors thus encouraging the efficient allocation of resources. The competitive nature of the environment under which the banking sector operates is of paramount importance.
Aim: The main aim of this study was to investigate the relationship between competition, efficiency and soundness in the South African banking sector.
Setting: The setting for this study was the South African banking sector.
Methods: We used a data set of 17 local and international banks for the period 2004–2015 and stochastic frontier models to analyse efficiency.
Results: Results show that the impact of competition on efficiency depended on the measure of competition used. When using the Lerner index there was a negative effect of competition on efficiency while the opposite was true when using the theoretically robust Boone indicator.
Conclusion: In the case of bank soundness, competition using the Boone indicator is negatively related to the Z score, implying that competition enhances bank soundness and these results supported the prudent and efficient management hypothesis.
Introduction
Competition is the main driver of strong and effective markets, encourages firms to innovate, enhances productivity, and results in the efficient allocation of resources. A competitive environment ensures that companies compete fairly and puts businesses under constant pressure to offer the best possible range of goods at the best possible prices. This makes competition the essential drive of productivity growth in any economy. In addition to improving quality, competition creates a wider choice for consumers and, therefore, by removing distortions to competition, we will reduce opportunities for corruption and rent seeking, thus helping markets to work better and maximising economic benefits.
Industrial organisation theory argues that the level of concentration in a market determines the degree of competition among firms. The Structure Conduct Performance (SCP) paradigm, proposed by Bain (1951), argues that markets dominated by a few large firms are less competitive than markets that are lowly concentrated. This implies that the higher the level of concentration in a market, the lower the level of competition. However, the contestable market theory emphasises that a highly concentrated market can be highly competitive, even if a few firms dominate it (Baumol 1982). Thus, there is no strong theoretical support for the notion that in markets that are more concentrated, market power is higher, and competition is lower. One undisputable fact, however, is that competition is important in enhancing efficient allocation of resources. Competition pushes firms to produce goods at the lowest possible costs in a way that accounts for consumer preferences.
The theoretical literature is awash with a number of hypotheses that seek to explain the relationship between competition and efficiency (Demesetz 1973; Hicks 1935; Liebenstein 1966; Peltzman 1977). The quiet life hypothesis, developed by Hicks (1935), argues that in highly concentrated markets, there is less pressure to compete, which results in reduced efforts by managers to operate efficiently. Thus, increased market concentration weakens market competition, and this affects productive efficiency. This hypothesis is synonymous with the competition-efficiency hypothesis and argues that increases in competition precipitate increases in profit efficiency since banks are forced to engage in proper screening and monitoring of borrowers resulting in lower levels of non-performing loans (Schaek & Cihak 2008; Williams 2004). Thus, according to the quiet life hypothesis, increased competition improves efficiency, implying that the relationship between these two variables is positive and runs from competition to efficiency. The efficient structure hypothesis proposed by Demesetz (1973) takes the argument further and argues that efficient banks will increase in market share and size at the expense of the inefficient banks, leading to a higher market concentration. In this hypothesis, efficiency leads to higher concentration, suggesting low levels of competition. The alternative to the competition-efficiency hypothesis is the competition-inefficiency hypothesis which argues that competition leads to a decline in efficiency (Schaek & Cihak 2008). The argument is that higher competition is likely to be associated with unstable and shorter bank client relationships since clients tend to have a high propensity to switch to other service providers. This creates information asymmetry and requires banks to spend more on the screening and monitoring of borrowers. Banks in turn will likely reduce relationship-building programmes and this affects the reusability and value of information. In this case, banks will incur greater expenses in keeping old and attracting new clients through investments into ATMs, new information systems and aggressive marketing (Schaek & Cihak 2008). In this case, competition reduces bank efficiency.
According to Simbanegavi, Greenberg and Gwatidzo (2015), a well-functioning banking sector contributes to economic growth via the more efficient allocation of resources and risk diversification. The competitiveness and efficiency of the banking sector is critical to the well-being of the economy because it helps facilitate the efficient movement of funds from surplus to deficit units, thereby encouraging savings and the optimal allocation of resources. Maredza et al.’s (2013) study also identified that lack of competition in banking is one of the aspects that is related to low levels of efficiency of commercial banks in Africa. The study noted that interest rate spreads, profits and overhead costs are high in African banking compared to other regions of the world. This competition-efficiency nexus is of importance to South Africa given that the country’s banking sector is dominated by four major banks that account for over 80% of total banking assets. This high level of concentration suggests low level of competition and hence inefficiency as per the quiet life hypothesis or the high levels of concentration do not necessarily suggest lower levels of competition as per the contestable market theory. Given these conflicting theoretical positions, the objective of this study is to investigate empirically the nature of the relationship between competition and efficiency in the South African banking sector and look at how these variables ultimately affect bank soundness.1
Although there are so many studies that have examined these relationships in the banking sector, there is none on South Africa. Most of the studies on South Africa have looked at the competitive conditions in the banking sector, all finding that the sector is monopolistically competitive (Mlambo & Ncube 2011; Simatele 2015; Simbanegavi et al. 2015). If the competitive conditions are good, we expect to see an improvement in the level of efficiency and financial stability.2 The competitiveness of the South African banking sector was under the spotlight recently, in 2017, after the Competition Commission investigated allegations of price fixing and market allocation (in the trading of foreign currency) by 17 banks including three South African banks (National Treasury 2017). These allegations are not entirely surprising given that the country’s banking sector is highly concentrated with five banks holding close to 99% market share (see Table 1). In addition, the recent (March 2018) placement under curatorship of VBS Mutual Bank by the South African Reserve Bank (SARB) because of imprudent banking practices also compromises the stability of the banking sector. It is, therefore, of interest to understand how the concentrated nature of the sector has affected efficiency and bank stability. The promotion of efficiency in the pricing and delivery of financial products is very important, especially given the devastating effects of the 2008–2009 global financial crisis. This study will also partly serve as an indirect test of the contestable market theory and its applicability to the South African banking industry. Our results show that the impact of competition on efficiency depends on the measure of competition used. When using the Lerner index, there is a negative effect of competition on efficiency, while the opposite is true when using the Boone indicator. In the case of bank soundness, the Boone indicator is negatively related to the Z score, implying that competition enhances bank soundness and these results support the prudent and efficient management hypothesis.
This article is organised as follows: the next section discusses some basic stylised facts about the South African banking sector, while section two covers literature review, followed by section three on methodology. Section four covers results analysis and the last section then concludes the article.
Some stylised facts about South African banking
The South African banking system is well developed and effectively regulated, comprising a central bank, the SARB, a few large banks and investment institutions, and a number of smaller banks, as well as lending and savings organisations (South African Reserve Bank [SARB] 2017). The banking sector is well developed and compares favourably with those of the developed world, and ranked 11th out of 138 countries in terms of financial market development in the Global Competitiveness Report. In terms of bank soundness, the country is ranked at number 2 out of 138 countries but ranked number 27 in terms of affordability of financial services. The financial sector together with real estate and business services for the past 10 years until 2016 has been contributing on average about 19% to gross domestic product (GDP), growing at 2.2% per year (Stats SA 2017).
The banking sector is comprised of 64 institutions and close to half of these are foreign bank representatives with locally controlled banks constituting 16% of the total (SARB 2017). The high number of players in the sector suggests an improved level of competitiveness in the market and this could be good for consumers. The total banking assets have been increasing over the years, moving from ZAR2.5 trillion in July 2008 to ZAR5 trillion in the same month in 2017. Other banking depth indicators also show an improvement in financial deepening since the year 2000. Bank deposits to GDP ratio increased from 50.1% in 2000 to 59.7% in 2015 and domestic credit to the private sector as a percentage of GDP also increased to 147% from 130% for the same period. The same pattern is replicated when looking at broad money to GDP, which increased from 53% in 2000 to 74% in 2015 (see Table 2).
Banking structure indicators support some of the findings on competitiveness found by many researchers in the sector. The five banks concentration ratio calculated by the World Bank increased from 94.8% in 2000 to 98.99% in 2015 suggesting a heavily concentrated industry (see Table 2). However, the intensity of competition is encouraging as the Panzar Rose H statistic increased from 0.79 in 2010 to 0.86 in 2015, still supporting the generally held view that the sector is monopolistically competitive. The Lerner index moved from 0.22 in 2000 to 0.17 in 2010, while the Bonne indicator changed from –0.09 in 2000 to +0.03 in 2015 (Global Financial Development 2017). The Lerner index suggests that there is some level of competitiveness in the banking sector but the Boone indicator suggests that the level of competitiveness is falling, although the changes are marginal.
However, looking at bank access and efficiency indicators calculated by the World Bank, bank lending deposit spreads have been falling since 2000 and the same is true for bank non-interest income to total income. This could suggest that competitive conditions are intensifying or efficiency is improving, resulting in banks reducing their prices. This trend is repeated even when looking at bank return on assets which has been falling since 2000. However, the bank Z score values have been increasing, supporting bank soundness and low risk profile of the sector. Finally, in terms of access, the number of bank branches to 100 000 adults has been increasing gradually from 7.3 in 2005 to 10.5 in 2015, and ATMs per 100 000 adults also increased from 25.5 in 2005 to 69.3 in 2015 (see Table 1).
Given that the banking structure and efficiency indicators in Table 1 point to improved levels of competition and efficiency, does this, therefore, suggest that there is a positive relationship between these two variables? Thus, given that Z score values (standard indirect measure of bank soundness) are increasing, does this mean competition or efficiency positively influence bank stability? These questions form the core objectives of this study.
Literature review
A handful of studies have been done on the South African banking sector. These studies are of two strands: one strand looked at the competitive settings in the sector while another group analysed efficiency conditions. Ncube (2009) used stochastic frontier analysis (SFA) to calculate the cost and profit efficiency of four large and four small banks in South Africa for the period 2000–2005. His results show that banks are generally 85% cost efficient with Investec being the most efficient bank while Standard Bank was the least. Profit efficiency levels were relatively lower at 55% for the banking sector with the most profit efficient banks being Capitec and Standard Bank with Nedbank and Absa being the least (Ncube 2009). Using the Krusral-Wallis analysis of variance tests, he found that there has been a significant change in cost efficiency over this period but no change in profit efficiency. A study by Erasmus and Makina (2014) analysed technical efficiency in the South African banking sector using the five largest banks. They used standard and alternative approaches to data envelope analysis (DEA) for the period before and after the financial crisis, that is 2006–2012. Their results show that Barclays Bank and Nedbank were the most technically efficient banks using the two approaches and they conclude that the global financial crisis did not have a significant impact on the technical efficiency of the major banks in South Africa. The results found by Erasmus and Makina were contrary to those obtained by Maredza and Ikhide (2013). Using data for the four largest commercial banks for the period 2000–2010 and calculating total factor productivity or efficiency using DEA’s Hicks-Moorsteen index, Maredza and Ikhide found technical efficiency scores to have been affected by the financial crisis. Results from their stage two Tobit model showed that efficiency was 17% lower during the crisis compared to the pre-crisis period. Mlambo and Ncube (2011) carried out another study on South African banks where they analysed the evolution of competition and efficiency of 26 banks between the years 1999 and 2008. Using DEA for measuring technical, allocative and cost efficiency and that the Panzar Rose model for estimating competitive conditions, they found that even though the number of efficient banks was falling, average efficiency was increasing and that the banking industry was monopolistic in nature. This study is an extension of what Mlambo and Ncube did and extends the analysis further by looking at the impact of competition and efficiency on bank soundness. Simbanegavi et al. (2015) also tested for competition in the South African banking sector employing the Panzar Rose and the Bresnahan models and using a data set of 14 banks over the period 1998–2008. They found the banking sector to be monopolistic in nature using the Panzar Rose model but could not reject the null hypothesis for perfect competition using the alternative Bresnahan approach. They conclude that these findings suggest that even though the banking sector is highly concentrated, this has not affected competition in the sector. It appears that studies that have analysed the competitiveness of the South African banking sector using the Panzar Rose methodology arrive at the same conclusion. Simatele (2015) also used a time varying Panzar Rose methodology to examine the relationship between bank structure and competition in South Africa for the period 1997–2014. Using a data set of 35 banks, she also found the sector to be monopolistically competitive, confirming the results found by Mlambo and Ncube, as well as Simbanegavi et al.
In Africa, a number of studies have also investigated the relationship between competition and efficiency using various competition and efficiency measurement techniques and finding mixed results. A study that employed DEA done in Ghana by Alhassan and Ohene-Asare (2016) found competition to improve cost efficiency, supporting the quiet life hypothesis; similar results were found for the Middle East North Africa (MENA) region by Apergis and Polemis (2016), concluding that increases in competition do not precede increases in cost efficiency. However, Saka, Aboagye and Gemegah (2012), also using DEA, found that in Ghana competition improves technical efficiency, supporting the efficient structure hypothesis. Their results were partly supported by Buchs and Mathisen (2005) who found that banks in Ghana behaved in a non-competitive manner and this may not be good for financial intermediation efficiency. Sarpong-Kumankoma, Abor and Aboagye (2017) also looked at competition and bank efficiency in sub-Saharan Africa, employing SFA and finding results inconsistent with the quiet life hypothesis. They found that increase in market power leads to greater bank cost efficiency, but the effect is weaker with higher levels of financial freedom. In the case of stability, Amidu and Wolfe (2013) analysed competition and stability in 55 emerging market countries, of which 22 were from Africa including South Africa. Their core finding was that competition increases stability, as diversification increases across and within both interest and non-interest income-generating activities of banks. Their results show a positive and significant relationship between competition and stability, supporting the competition stability view. Another study was done by Hope, Gwatidzo and Ntuli (2013) using 10 African countries and they found that there is a robust positive relationship between market power and financial stability. This result suggests that there is a trade-off between bank competition and financial sector stability in these African countries, as per the competition-fragility view.
Studies on the relationship between competition and efficiency in the banking sector in non-African countries also abound. Most of these studies use the Granger causality tests to analyse the relationship between competition and efficiency. They only differ in the way they measure efficiency: one group of this literature uses DEA while the other employs SFA. They all measure competition using non-structural measures like Panzar Rose H statistics, the Lerner index and the Boone indicator. Regardless of the efficiency technique used, there is no consensus on the nature of the relationship between efficiency and competition. Rahim (2016), using the Malaysian commercial banking sector, found the same relationship as Schaek and Cihak (2008) who used banks in Europe and the United States. Rahim found a positive effect of competition on technical efficiency while Schaeck and Cihak found competition to be positively related to both profit and cost efficiency. Schaek and Cihak also found that increased competition increases bank soundness via the efficiency channel. Casu and Girardone (2009), using banks from selected European Union (EU) countries for the period 2000–2005, found a negative relationship between market power and efficiency and the same results were obtained by Fernandez de Guevara, Maudos and Perez (2005), also using EU data for the period 1993–2002. The latter rejected the quiet life hypothesis in the same manner that Podpiera, Weill and Schobert (2008) rejected it using Czech Republic data for the period 1994–2005.
Using SFA, Fungacova, Pessarossi and Weill (2013) studied whether bank competition is detrimental to efficiency in China using data for the period 2002–2011. Their finding is inconsistent with the quiet life hypothesis that market power has a negative impact on cost efficiency. Maudos and Solis (2009) performed a similar analysis for Mexican banks by considering separately the Lerner index for deposits and loans. While they observe a negative link between competition and efficiency on the deposit market, they find an opposite result for the loan market. All these studies show that the relationship between competition and efficiency is not clear cut and thus varies from one country to another.
Methodology
The approach followed in this article to analyse the relationship between competition, efficiency and soundness is divided into three parts. We first measure the level of competition using the Lerner index and the Boone indicator, and after that we compute various efficiency scores (technical, cost and profit). Lastly, we then compute bank soundness using the Z scores and non-performing loans.
Measuring bank competition
There are a number of techniques developed to measure competition in any industry. These measures are grouped into structural and non-structural and the former are based on the SCP paradigm developed by Mason (1939) and Bain (1951). The SCP model explains the aspects of conduct and performance of firms in terms of the structural characteristics of the markets in which they operate and argues that the more concentrated an industry is, the easier it is for firms to operate in an uncompetitive manner (Leon 2015). Structural measures include the number of firms, the concentration ratios and the Herfindahl Hirschman index (HHI).3 The first generation of non-structural measures include the Lerner index developed in 1934 and the conjectural variation models like the Panzar Rose H statistic developed in 1987, the Bresnahan-Lau test in 1982 and the Boone indicator in 2008.
In this study, since we use bank level data, we use a measure of market power that is calculated at bank level like the Lerner index. This index captures the divergence between product prices and marginal cost of production. The price and marginal cost are equal in perfect competition but diverge in less competitive markets.
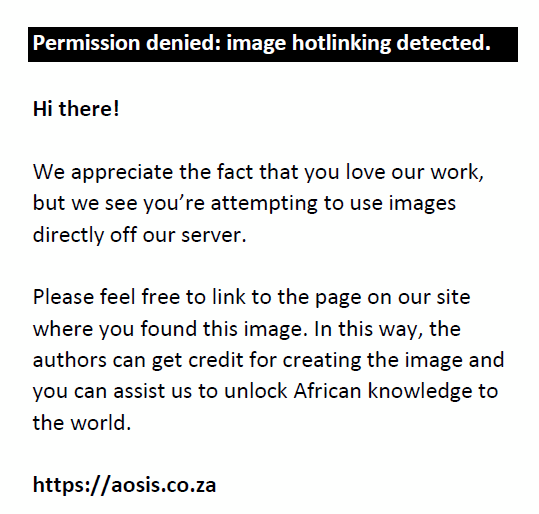
In Equation 1, pit is the output price of bank i at time t and is defined as total revenue4 divided by total assets. Marginal cost is calculated by differentiating the translog cost function with one output (total assets) by output. This index ranges between 0 and 1, and a bigger wedge between price and marginal cost suggests greater market power. We can alternatively present this as follows so that it becomes clear how this index is calculated: .
qi is the quantity produced by firm i, Q is total quantity and P(Q) is the market price. C(qiwi) is the total costs of firm i and wi is the vector of the prices of inputs used. The differential of total costs with respect to qi gives us marginal cost.
We follow the approach adopted by Fungacova et al. (2013) by formulating a translog cost function where output is measured using total assets or loans and three input prices, namely price of labour, price of borrowed funds and price of capital.5 We also estimate one cost function for all the periods and symmetry and linear homogeneity restrictions in input prices are imposed. The translog cost function that will be estimated is specified as follows:
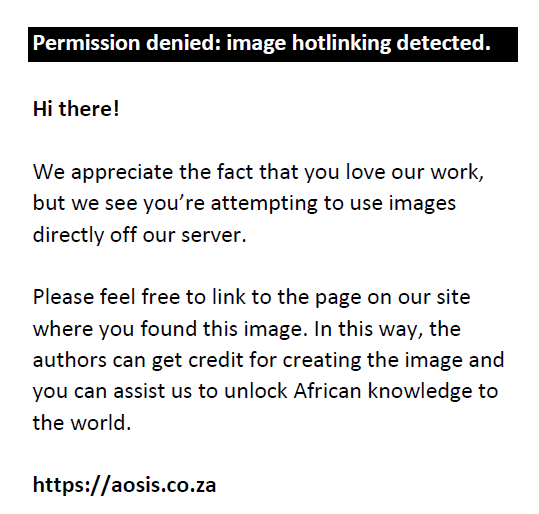
In Equation 2, q is a measure of output and equal to total assets, w is the price of inputs, with price of labour measured using the ratio of personnel expenses to total assets; the price of capital is the ratio of other non-interest expenses to fixed assets and the price of borrowed funds is the ratio of interest paid to total funding. Total cost is the sum of personnel expenses, other non-interest expenses and interest paid (Fungacova et al. 2013). The coefficients of this cost function are used to compute marginal costs values as follows:
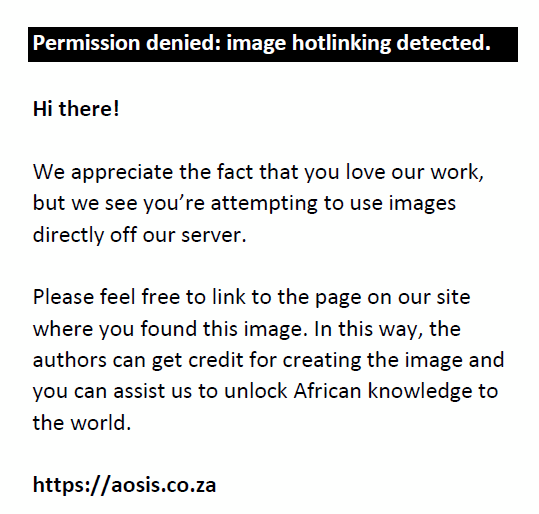
Using marginal costs and price, we are able to calculate the Lerner index for each bank and for each year and thus obtain a direct bank level measure of competition. We also go further and use the adjusted Lerner index6, calculated as follows: .
Q is total output.
Although the Lerner index has been widely used in the empirical literature, the theoretical foundations of the index as a competition measure are not robust (Boone 2008). Amir (2000), Bulow and Klemperer (1999), Rosenthal (1980) and Stiglitz (1989), for example, present models where more intense competition leads to higher instead of lower Lerner index values. Corts (1999) shows that the estimates of the Lerner index will typically underestimate the price-cost margin and the level of market conduct itself. Boone (2008) argues that their competition measure is theoretically more robust and does not pose more data that are stringent requirements than the Lerner index. Even though they found the Lerner index and the Boone indicator highly correlated on average, the Lerner index tends to misrepresent the development of competition over time in markets with a few firms and a high concentration (markets most relevant for competition policy and regulation) and is thus less reliable (Boone, Van Ours & Van der Wiel 2007). However, the empirical applicability and robustness of the Boone indicator is still unknown.7
We use the Boone indicator to measure market power and this measure is argued to capture directly the relationship between competition and efficiency. The premise on which it is built is that banks that are more efficient attain better performance or higher profits and that this outcome increases the degree of competition (Schaek & Cihak 2008). It is modelled as a relationship between profits and marginal costs because an increase in costs reduces profits, but in competitive markets the impact of changes in costs is relatively high since in this market inefficiency is heavily punished (Cummins et al. 2017). We construct this indicator from a regression model as follows:
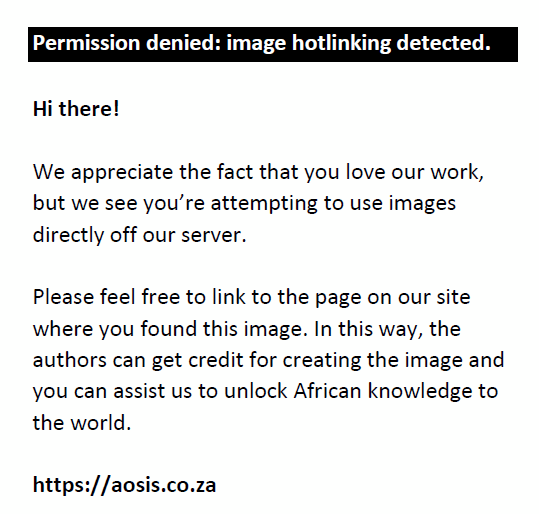
In Equation 4, p is profit and MC is marginal costs. The parameter bt is the Boone indicator measured for each year and is expected to be negative, showing that increases in competition raise the profits of more efficient banks. We run this model for each year across all banks to estimate the Boone indicator parameter.
Measuring efficiency in banking
Efficiency measures used in banking analysis are varied. We use allocative efficiency, technical efficiency, cost efficiency and profit efficiency. Allocative efficiency is the extent to which resources are being allocated to the use with the highest expected value, while a firm is technically efficient if it produces a given set of outputs using the smallest possible amount of inputs (Ncube 2009). A firm is also said to be cost efficient if it is both allocatively and technically efficient (Berger & Mester 1997). Cost efficiency measures how close a bank is to its optimal cost when producing the same bundle of outputs (Fungacova et al. 2013). Profit efficiency measures how close a bank gets to the efficiency frontier, which denotes the maximum achievable profit, given a particular level of input and output prices (Berger & Mester 1997). This profit measure takes into account performance from both the cost and revenue side of bank business, and the argument is that profit efficiency is superior as it embraces cost efficiency (Schaek & Cihak 2008).
In this study, we employ SFA to generate different efficiency scores (technical, cost and profit) for each bank in the sample during the period under analysis. We use the Battese and Coelli (1995) model which provides estimates of efficiency in a single step in which bank effects are directly influenced by a number of variables and is assumed to be superior to a two-step procedure, in which the estimated efficiency scores obtained from the stochastic frontier are then regressed during a second step on a set of explanatory variables. The SFA has the advantage of separating random noise from efficiency, while DEA assumes that everything not accounted for by inputs (random noise) is efficiency. The banking sector is affected by different economic and industry-specific regulations and these can affect performance and be difficult to capture when estimating productivity models. In the case of SFA these kind of factors will be captured in the error term but will be ignored by the DEA approach.
The Battese and Coelli (1995) model is expressed as follows:
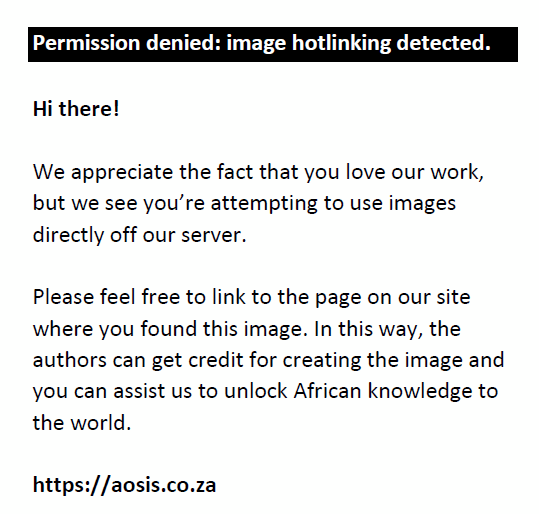
In Equation 5, Yi is output for firm i, X is a set of inputs, b is a set of parameters and vi is a random error term assumed to be iid is a non-negative random variable representing inefficiency, independently distributed and truncated at . The mean of this distribution is assumed to be a function of a number of explanatory variables and given as ui = diZi. This gives the following inefficiency term:
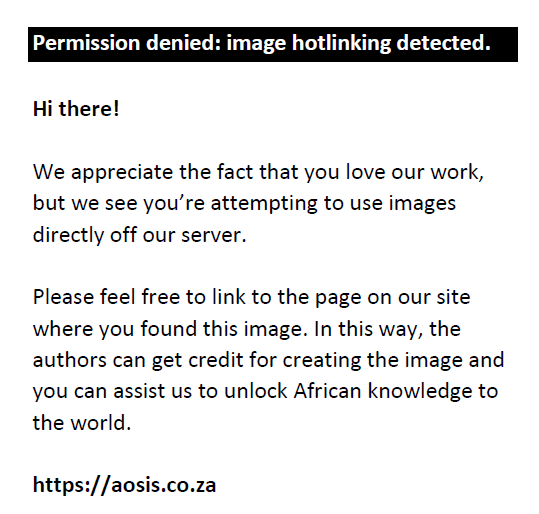
In Equation 6, Zi is a vector of variables that may affect firm efficiency, d is also a vector of parameters to be estimated and Wi is a random variable defined by the truncation of the normal distribution with zero mean and constant variance (s2). In this case the point of truncation –Zid is where Wi > – Zid. These assumptions are consistent with ui being a non-negative truncation of the N(Zids2) distribution (Battese & Coelli 1995).
The production function parameters b and the inefficiency coefficients dj are estimated using maximum likelihood techniques together with the following variance parameters.
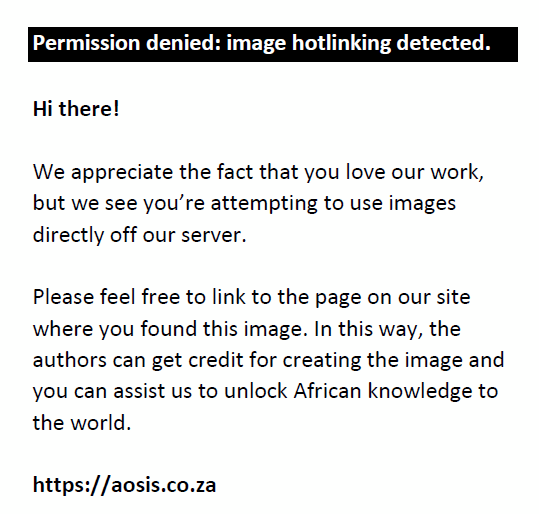
Since technical efficiency is the ratio of observed production over the maximum possible technical output (a case of zero inefficiency), the efficiency measure TE of firm i in any period could be expressed as follows:
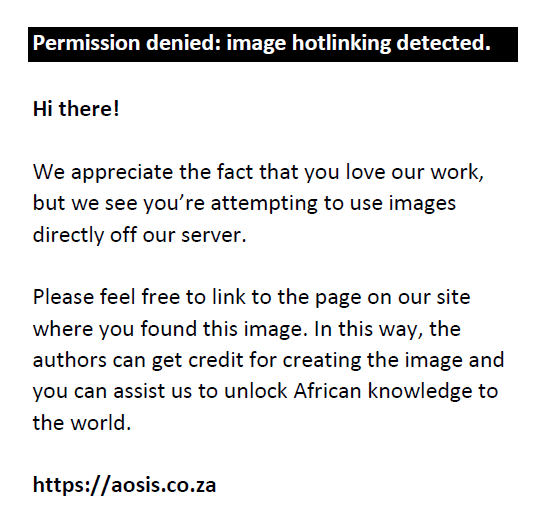
The above efficiency scores (Equation 8) will assume the value of 1 when the firm is fully efficient and less than 1 otherwise.
To estimate technical inefficiency scores we will use a translog production function because of its flexible nature. The stochastic frontier translog production function to be estimated is specified as follows:
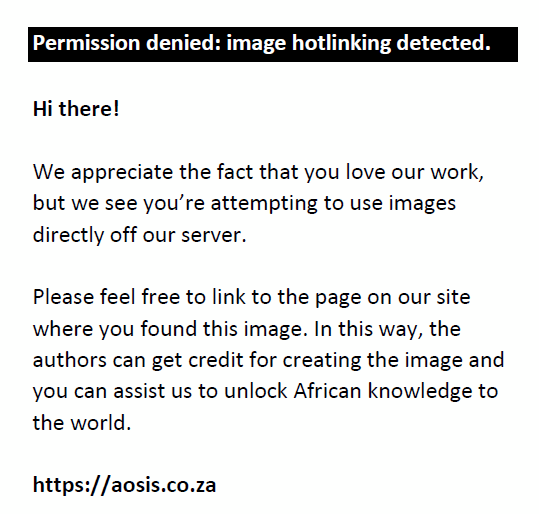
In Equation 9, K represents capital, L is labour and BF equals borrowed funds used in production. We assume a half-normal distribution for the inefficiency term.8 In addition to technical efficiency, we also measure cost and profit efficiency. In the case of banks, cost efficiency is the minimum cost needed to produce the bank’s output vector if the bank was as efficient as the best- practice bank in the sample that is facing the same exogenous variables. Profit efficiency on the other hand measures how close a bank gets to the efficiency frontier, which denotes the maximum achievable profit, given a particular level of input and output prices (Ncube 2009; Schaek & Cihak 2008). Profit efficiency9 has the benefit of taking into account not only the cost side but also the revenue side of bank business and this distinction is important because banks compete not only through cutting costs but also through adjustments in revenues (Schaek & Cihak 2008). The efficiency channel is sometimes the conduit through which competition affects stability. Therefore, in this study we want to identify the type of efficiency important for encouraging stability. Cost and profit efficiency levels will be estimated using Equations 10 and 11:
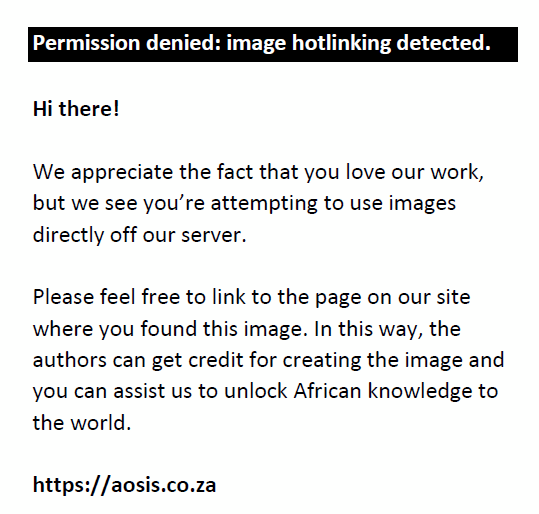
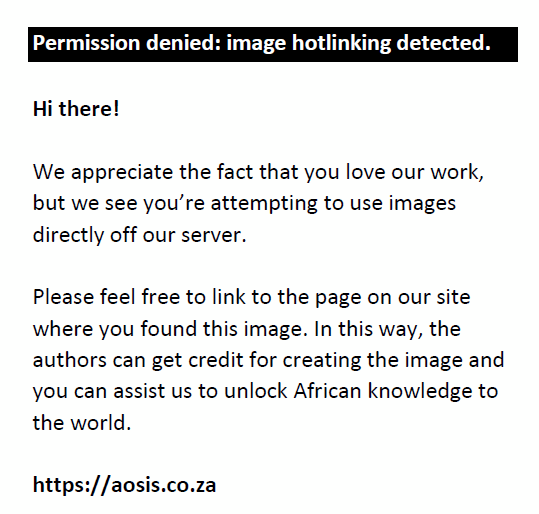
In these equations, C is total cost and w and z are prices of inputs and output quantity. p is profit and q is a constant added to avoid taking the log of a negative number (in the case where profits are negative). vi and ui represent the white noise and the efficiency term. We impose standard homogeneity conditions by scaling profits and cost functions with one of the input prices (borrowed funds).
The resulting efficiency effects are specified as follows:
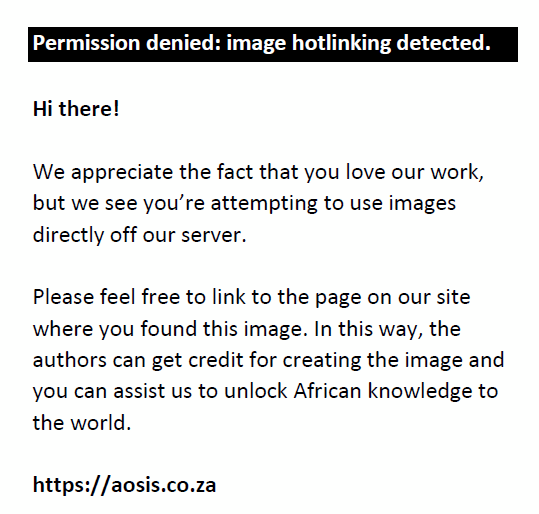
Equation 12 is an expanded version of Equation 6. Equations 5 and 6 will be estimated simultaneously using Frontier 4.1 model. The data used in this article are sourced from BankScope and SARB.
Measuring bank stability or soundness
Bank stability is the absence of the macro-economic costs of disturbances in the system of financial exchange between households, businesses and financial service firms. A sound banking system ensures the optimal allocation of capital resources to prevent costly banking system crises and their associated adverse feedback effects on the real economy (SARB 2017).
The standard approach used in the literature to measure soundness is to use the Z score, calculated as follows: this method has the advantage of being easy to compute as it only uses accounting information, combining bank buffers (capital and profits) with the risks they face (standard deviation of returns) in a way that is grounded in theory (Schaek & Cihak 2008).
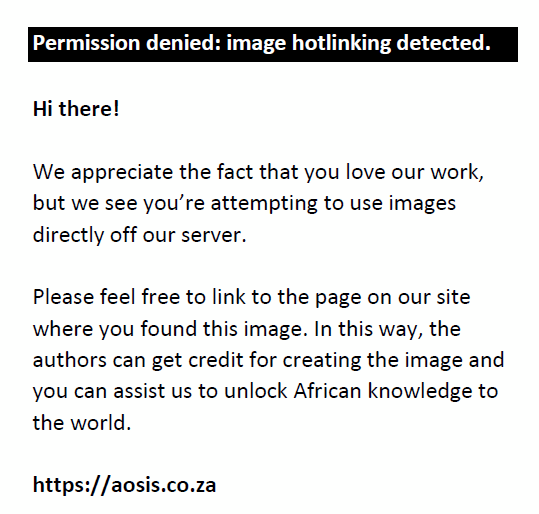
In Equation 13, ROA is the bank’s return on assets, EAR is the equity to assets ratio while sROA is standard deviation of return on assets calculated over the sampling horizon. A higher Z score implies a lower probability of insolvency (Schaeck & Cihak 2008). We also alternatively measured soundness using ratio of non-performing loans as a percentage of total loans. To investigate bank soundness, we estimate the following model:
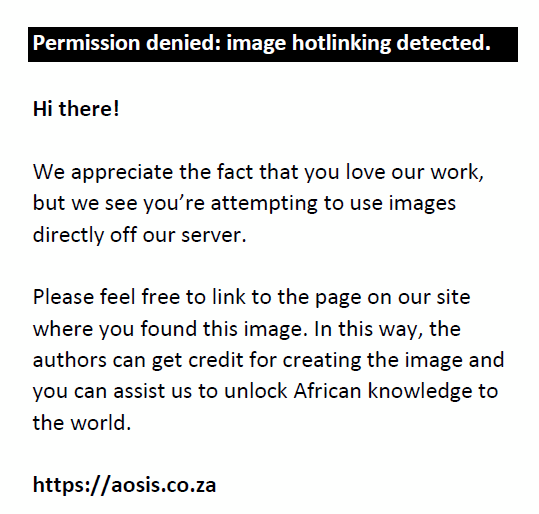
In Equation 14, BSFu captures bank-specific features like age, size and foreign ownership while Comp is bank competition measured using the Lerner and Boone indicators. The last variable, Eff, is bank efficiency. We also control for macro-economic stability and economic performance using inflation and GDP.
Data
The bank level data used in this study is from BankScope and covers 17 local and international banks and spans the period 2004–2015. What influenced the sample size is the availability of comparable data on the variables of interest as well as the timeline. Some banks did not have data spanning 2004–2015 and were therefore excluded. The Bureau Van Dijk, which compiles Bankscope, now publishes financial statements covering the past five years and this again affected the inclusion of a number of banks in the study. The sample included commercial banks, a mutual bank and an infrastructure bank. Our sample size is similar to the one used by Simbanegavi et al. (2015) of 14 banks to investigate the level of competition.
Results and analysis
Descriptive or stylised facts about the sampled banks
In the first part of this section we computed competition and efficiency scores and a summary of these statistics is presented in Tables 3, 4 and 5. What is clear from these statistics is that efficiency levels are generally high, above 80%, and that these banks are more profit efficient than they are cost and technically efficient (see Table 5). However, all these different measures of efficiency appear to have decreased over time, though the decline is marginal. There is also very little difference between the average efficiency levels of the big four banks and those of the 17 banks used in this study. This suggests that the computed efficiency scores are driven largely by the big four banks rather than the other 13 small banks included in the sample. These results are similar to those found by Maredza and Ikhide (2013) using the four largest South African banks and technical efficiency scores of around 98%. Ncube (2009) using eight banks and Okeahalam (2006) using bank branches in all the nine South African provinces, as well as Obeholzer and Van der Westhuizen (2004) all found efficiency scores of 84%. Mlambo and Ncube (2011) using 25 banks found technical efficiency scores of around 67% and cost efficiency of 42%. The size of the sample and the sample period could be one of the reasons driving these differences in efficiency scores in these South African studies. In the case of competitiveness, the Lerner index shows that competitiveness deteriorated between 2004 and 2007 and improved during the periods 2008–2010 and 2014–2015. In the case of the Boone indicator, the more negative the value, the higher the degree of competition, because the effect of reallocation is stronger. This indicator has consistently been more negative from 2011 until 2015, suggesting that competitive conditions improved during this period. In the case of competition, most studies on South Africa used country level indicators like the Panzar Rose (Mlambo & Ncube 2011; Simatele 2015; Simbanegavi et al. 2015) and the Bresnahan (Simbanegavi et al. 2015). However, statistics from Global Financial Development (2017) show that using the Lerner index (period 2000–2010) suggests that there is some level of competitiveness in the banking sector but the Boone indicator (period 2000–2015) suggests that the level of competitiveness is falling.
TABLE 3: Summary of statistics for all banks. |
TABLE 4: Summary of statistics for big four banks (Standard Bank, Nedbank, Amalgamated Banks of South Africa [ABSA]/Barclays and First National Bank). |
TABLE 5: Firm characteristics, efficiency and competition. |
The change in competitive conditions between 2004 and 2015 juxtaposed with the changes in efficiency levels over the same period show that the relationship between these two variables is not clear and is something that should be investigated using econometrics. We also went further and used descriptive statistics to explain how bank characteristics like age and size relate to bank efficiency and competitiveness. Table 5 shows that older and bigger banks (in terms of total assets) are less efficient than younger and smaller banks. Foreign-owned banks are slightly more efficient than local banks. In terms of competition, banks whose total assets size is greater than R173 100 million have more market power than smaller banks and this is the case even when looking at locally owned banks. Banks older than the average age of 52 years appear to have less market power than younger banks.10
The correlation matrix (see Table 2-A1) also adds some insights into how these variables move together. The size and age variables appear to move negatively with all efficiency variables and this is the case with the competition indicators like the Lerner index. The Z score, which is our measure of bank soundness, is positively correlated with efficiency variables suggesting prima facie that efficiency may be good for bank soundness.
Analysis of regression results
The first model looked at the relationship between technical efficiency and different indicators of competitiveness (see Table 6). We also included bank-specific variables like age, size measured using total assets and a foreign ownership dummy. These results partly confirm what is presented under descriptive statistics (Table 5): that larger banks are less efficient than smaller banks. Bank size has a consistently negative but significant relationship with technical efficiency. This could be explained by the fact that small banks have to be very innovative in order to survive and be able to attract clients by offering products or financial services at prices below what big banks are charging. This probably explains why even though the South African banking sector is dominated by a few large banks, this has never stopped new entrants into the sector and bank turnover has also been low. These results are contrary to what was found by Hauner and Peiris (2005) in Uganda, Ataullah, Cockerill and Le (2004) and Chen, Skully and Brown (2005), but in line with what Isik and Hassan (2003), Girardone, Molyneux and Gardener (2004) and Weill (2004) found. Bank age also appears to have a negative and significant relationship with technical efficiency. Thus, older banks are less technically efficient than younger banks. In a highly concentrated banking environment like the one in South Africa, new banks can only enter and survive longer if they innovate or offer products that are not offered by the existing banks and if they offer the same products at lower prices. Albert (2012), however, found that age has a positive effect on efficiency using Egyptian banks, while Karim, Chan and Hassan (2010) found age to be positive and insignificant when related to efficiency and stability in Malaysia and Singapore. The correlation matrix (Table 2-A1) shows a relatively high level of association between age and size, something that could indicate multicollinearity and affect the impact of these variables. To control for this, we ran the efficiency models again, dropping either the age or size variable. In all the models, the sign of the age and size variable as well as competition indicators did not change.11 Being foreign owned does not appear to be important in enhancing technical efficiency in South Africa. This could be explained by the fact that foreign technologies have to be adapted to local conditions before they can be successfully implemented. Thus, you need to fully understand the local consumer market first before you can introduce new financial products or technologies. Foreign financial innovations or technologies may not be successful locally unless adapted to local conditions. This result, however, is not consistent with what Hauner and Peiris (2005) found in Uganda where efficiency was found to increase with foreign ownership. Karim et al. found foreign ownership to be negative and insignificant when related to efficiency in Malaysia and Singapore.
To analyse the relationship between competition and efficiency, we used the Lerner index and the Boone indicator. Using the Lerner index, the results show a negative and significant relationship between these variables supporting the efficient structure hypothesis. This result is similar to that found by Casu and Girardone (2009) using EU banks. The Boone indicator and adjusted Lerner results, however, support the quiet life hypothesis and show a positive relationship between technical efficiency and competition. We also used the Generalised Methods of Moments (GMM) model and the instrumental variable technique to take care of possible endogeneity problems in the model. This is because the quiet life model hypothesises that competition increases efficiency while the competition-inefficient model assumes that competition reduces efficiency. Results do not change much and still show a negative relationship between competition and efficiency and the same pattern with other bank characteristics.12 The shortcoming of using the GMM in this case is that it assumes that efficiency in the previous year affects efficiency in the current period. This assumption may render the competition indicator impotent. Thus, if competition affects efficiency, its lagged value already contains this information and this could be the reason why it is insignificant.
We followed the same approach as the one used in analysing technical efficiency in Table 6, in analysing the determinants of cost and profit efficiency in the South African banking sector. The results (see Table 7) are generally similar to what we found under technical efficiency. Foreign ownership, bank size and bank age are all negatively related to cost and profit efficiency except that age is no longer statistically significant. The Lerner and adjusted Lerner indices are all significant and negatively related to these two efficiency variables while the Boone indicator still show a positive relationship. Thus, using the Lerner indices, these results support the competition inefficiency hypothesis while the Boone indicator is in support of the quiet life hypothesis or the competition-efficiency hypothesis. The relationship between competition and profit efficiency using the Boone indicator also support what Schaek and Cihak (2008) refer to as the competition-efficiency hypothesis, which is adapted from the efficient structure hypothesis proposed by Demesetz (1973). Under the competition-efficiency hypothesis, increases in competition precipitates increases in profit efficiency. On the contrary, the results using the Lerner index support the alternative, which they called the competition inefficiency hypothesis. In this case, competition leads to a decline in bank efficiency. According to Boone (2008), the theoretical foundations of the Lerner index are not robust and some theoretical papers have found more models where intense competition leads to higher Lerner index values. The fact that the Boone indicator also performs better in a highly concentrated market like the one in South Africa reduces the attractiveness of the Lerner index results.
TABLE 7: Cost and profit efficiency determinants. |
In Table 1-A1 (in the appendix), we also estimated a model using competition indicators as dependent variables. The results generally confirm what we found above: that there is a negative relationship between competition and efficiency using the Lerner indices but a positive relationship using the Boone indicator. Thus, the Lerner index results support the efficient structure hypothesis that efficient banks reduce bank competition.
Competition, efficiency and bank soundness
The final section of this article looks at the impact of competition and efficiency on bank soundness. We measure bank soundness here using Z scores. The objective is to find out which bank level characteristics affect bank stability. Schaek and Cihak (2008) argue that the popularity of the Z score as a measure of bank soundness stems from that fact that it combines bank’s capital and profits with the risk they face in a way that is grounded in theory. The Z score is inversely related to the probability of a financial institution’s insolvency and the higher this value, the lower the probability of insolvency. The other advantage of this measure is that it is easy to compute because it only requires each bank’s accounting information compared to market-based measures such as distance to default. The bank soundness results are presented in Table 8 and the impact of competition indicators appear mixed. The Lerner indices show a positive and significant relationship with bank soundness, while the impact using the Boone indicator is negative though insignificant. What is, however, clear in these results is that efficiency indicators have a positive and significant effect on bank soundness. This result supports what is referred to in the literature as the ‘prudent and efficient management hypothesis’ (Koetter & Porath 2007; Petersen & Rajan 1997; Schaek & Cihak 2008). The argument is that more efficient banks have lower risks and are sounder than their less efficient counterparts. The negative value of the Boone indicator and the positive value of the Lerner index support this hypothesis in this South African banking sample. Schaek and Cihak, using data from Europe and the United States, as well as Cummins et al. (2017), using European life insurance markets found similar results. Thus, an increase in the Lerner index signals a fall in the level of competition and since we found a negative relationship between competition and efficiency, this means an increase in efficiency which increases bank soundness. The negative and significant effect of the adjusted Lerner index supports the presence of what Schaeck and Cihak refer to as the ‘poor and inefficient management hypothesis’. In this hypothesis, competition adversely influences bank efficiency, resulting in a negative effect on bank soundness. Thus if bank efficiency declines, these banks will do whatever it takes to retain old clients and attract new customers and by so doing may end up not employing sophisticated credit scoring systems and may also lack skills in assessing the value of collateral. This may result in a high proportion of non-performing loans and this negatively affects bank soundness (Schaeck & Cihak 2008). Using non-performing loans (see Table 3-A1 in the appendix) all competition and efficiency indicators show a negative effect on stability, partly supporting some of the results found using the Z score values.
Results in Table 8 also show that bank age and foreign ownership variables are negatively related to the Z score but the effect is insignificant. However, in the case of bank size, there is a positive relationship and this is significant. This suggests that bigger banks have a lower probability of insolvency than smaller banks. Karim et al. (2010), however, found age to be positive and foreign ownership to be negatively related to financial stability in Malaysia and Singapore. We also introduce two macro-economic variables and they appear to carry expected signs. Inflation has a negative effect on the Z score while GDP per capita carries a positive sign.
Conclusion
The main aim of this study was to investigate the relationship between competition and efficiency in the South African banking sector, then go further, and see how these variables affect bank soundness. Results show that the impact of competition on efficiency depends on the measure of competition used. When using the Lerner index there is a negative effect of competition on efficiency while the opposite is true when using the Boone indicator. Results also show that bank size measured using total assets is significantly negatively related to efficiency. In the case of bank soundness, our results are partly consistent with what other researchers (Cummins et al. 2017; Schaeck & Cihak 2008) have found. Thus, competition using the Boone indicator is negatively related to the Z score, implying that competition enhances bank soundness and these results support the prudent and efficient management hypothesis. This is also the case when using the Lerner index. Although Boone (2008) argued that their new theory-based measure of competition performs better than the traditional Lerner index, especially in markets with a few firms and a high concentration, a feature characteristic of the South African banking landscape, the empirical robustness of this indicator remains questionable. However, given that our five-bank concentration ratio estimated for year 2015 was around 99% (see Table 1), the South African banking sector is undoubtedly highly concentrated and a measure that takes this into account, as is the case with the Boone indicator, is ideal. Our conclusions are therefore in line with the Boone indicator results, suggesting that the competition-efficiency relationship in South Africa supports the quiet life hypothesis in which competition enhances efficiency. The improvement in the level of competition since 2011 partly supports the contestable market theory.
Other macro-economic variables used in the study also show consistent results. These results mean that the relationship between competition and efficiency in the South African banking sector supports the efficient structure hypothesis and the competition inefficiency hypothesis when using the Lerner index, but when using the Boone indicator results confirm the competition-efficiency hypothesis or the quiet life hypothesis. Since the relationship between competition and bank soundness is generally unambiguous, there is therefore need for the regulatory authorities to weed out anti-competitive practices or barriers to entry into the banking sector. They should also ensure that the big four banks do not abuse their market dominance but that contestable market conditions are promoted. The enactment of the Financial Sector Regulation Bill, which intends to put in place an intensive, intrusive, and effective regulatory framework that will help in the implementation of the Twin Peaks model, is a welcome move. The Prudential Authority at SARB will enhance safety and soundness while the Financial Sector Conduct Authority (current FSB) will protect financial customers and ensure they are treated fairly. For future research, it would be informative to examine non-linearities between competition and soundness to ascertain whether there is an inflection point as found by Fernandez and Garza-Garcia (2015), Berger, Klapper and Turk-Ariss (2009), Tabak, Fazio and Cajueiro (2012) and Fu, Lin and Molyneux (2014). There is need for more studies to investigate empirically the robustness of the Boone indicator as a better measure of competition.
Acknowledgements
Besides thanks to the National Research Foundation (NRF) for funding and the anonymous reviewers, there is no other person to acknowledge who assisted with this study.
Competing interests
The author declares that he has no financial or personal relationships that may have inappropriately influenced him in writing this article.
References
Albert, N., 2012, ‘Determinants of banking efficiency: Evidence from Egypt’, International Business Research 8, 2–9.
Alhassan, A.L. & Ohene-Asare, K., 2016, ‘Competition and bank efficiency in emerging markets. Empirical evidence from Ghana’, African Journal of Economic and Management Studies 7, 268–288. https://doi.org/10.1108/AJEMS-01-2014-0007
Amir, R., 2000, ‘Market structure, scale economies and industry performance’, Discussion Papers 00-08, Department of Economics, University of Copenhagen, Denmark.
Ataullah, A., Cockerill, T. & Le, H., 2004, ‘Financial liberalization and bank efficiency: A comparative analysis of India and Pakistan’, Applied Economics 36(17), 1915–1924.
Amidu, M. & Wolfe, S., 2013, ‘Does bank competition and diversification lead to greater stability? Evidence from emerging markets’, Review of Development Finance 3, 152–166. https://doi.org/10.1016/j.rdf.2013.08.002
Apergis, N. & Polemis, M.L., 2016, ‘Competition and efficiency in the MENA banking region: A non-structural DEA approach’, Applied Economics 10, 1–16. https://doi.org/10.1080/00036846.2016.1176112
Bain, J., 1951, ‘Relation of profit rate to industry concentration’, Quarterly Journal of Economics 65, 293–324. https://doi.org/10.2307/1882217
Baumol, W.J., 1982, ‘Contestable markets: An uprising of the theory of industrial structure’, American Economic Review 72(1), 1–15.
Battese, G.E. & Coelli, T.J., 1995, ‘A model of technical inefficiency effects in a stochastic frontier production for panel data’, Empirical Economics 20, 325–332. https://doi.org/10.1007/BF01205442
Boone, J., Van Ours, J. & Van der Wiel, H., 2007, ‘How (not) to measure competition’ CPB Discussion Paper, No 91, Bureau for Economic Policy Analysis, The Hague, The Netherlands.
Boone, J., 2008, ‘A new way to measure competition’, The Economic Journal 118, 1245–1261. https://doi.org/10.1111/j.1468-0297.2008.02168.x
Buchs, T., & Mathisen, J., 2005, ‘Competition and efficiency in banking: Behavioural evidence from Ghana’, IMF Working papers WP/05/17, IMF, Washington, DC.
Bulow, J. & Klemperer, P., 1999, ‘Prices and the winner’s curse’, RAND Journal of Economics 33(1), 1–21. https://doi.org/10.2307/2696372
Berger, A.N. & Humphrey, D.B., 1997, ‘Efficiency of financial institutions: International survey and directions for future research’, European Journal of Operational Research 98, 175–212. https://doi.org/10.1016/S0377-2217(96)00342-6
Berger, A.N. & Mester, L., 1997, ‘Inside the black box. What explains differences in the efficiency of financial institutions?’, Journal of Banking and Finance 21, 895–947. https://doi.org/10.1016/S0378-4266(97)00010-1
Berger, A.N., Klapper, L.F. & Turk-Ariss, R., 2009, ‘Bank competition and financial stability’, Journal of Financial Services Research 35(2), 99–118. https://doi.org/10.1007/s10693-008-0050-7
Casu, B., & Girardone, C., 2009, ‘Testing the relationship between competition and efficiency in banking. A panel data analysis’, Economics Letters 105, 134–137. https://doi.org/10.1016/j.econlet.2009.06.018
Chen, X., Skully, M. & Brown, K., 2005, ‘Banking efficiency in China: Application of DEA to pre- and post-deregulation eras: 1993–2000. China Economic Review 16(3), 229–245. https://doi.org/10.1016/j.chieco.2005.02.001
Coelli, T.J., Rao, D.S.P. & Battese, G.E., 1998. ‘An introduction to efficiency and productivity analysis’, Kluwer Academic Publishers, Norwell, MA.
Corts, K., 1999, ‘Conduct parameters and the measurement of market power’, Journal of Econometrics 88, 227–250. https://doi.org/10.1016/S0304-4076(98)00028-1
Cummins, J.D., Rubio Misas, M. & Vencappa, D., 2017, ‘Competition, efficiency and soundness in European Life Insurance markets’, Journal of Financial Stability 28, 66–78. https://doi.org/10.1016/j.jfs.2016.11.007
Demesetz, H., 1973, ‘Industry structure, market rivalry and public policy’, Journal of Law and Economics 16, 1–9. https://doi.org/10.1086/466752
Erasmus, C. & Makina, D., 2014, ‘An empirical study of bank efficiency in South Africa using the standard and alternative approaches to Data Envelopment Analysis (DEA)’, Journal of Economics and Behavioural Studies 6(4), 310–317.
Fernandez de Guevara, J., Maudos, J. & Perez, F., 2005, ‘Market power in European banking sectors’, Journal of Financial Services Research 27(2), 109–137. https://doi.org/10.1007/s10693-005-6665-z
Fernandez, R.O. & Garza-Garcia, J.G., 2015, ‘The relationship between bank competition and financial stability. A case study of the Mexican banking industry’, Ensayos Revista de Economía 34, 103–120.
Fu, X., Lin, Y. & Molyneux, P., 2014, ‘Bank competition and financial stability in Asia Pacific’, Journal of Banking & Finance 38, 64–77. https://doi.org/10.1016/j.jbankfin.2013.09.012
Fungacova, Z., Pessarossi, P. & Weill, L., 2012, ‘Is bank competition detrimental to efficiency? Evidence from China’, China Economic Review 27, 121–134. https://doi.org/10.1016/j.chieco.2013.09.004
Global Financial Development Report, 2017, viewed October 2017, from http://www.worldbank.org/en/publication/gfdr/report.
Girardone, C., Molyneux, P. & Gardener, E.P.M., 2004, ‘Analysing the determinants of bank efficiency: The case of Italian banks’, Applied Economics 36(3), 215–227. https://doi.org/10.1080/0003684042000175334
Hauner, D. & Peiris, S.J., 2005, ‘Banking efficiency and competition in low-income countries: The case of Uganda’, Applied Economics 40, 2703–2720. https://doi.org/10.1080/00036840600972456
Hope, C.J., Gwatidzo, T. & Ntuli, M., 2013, ‘Investigating the effect of bank competition on financial stability in ten African countries’, International Business and Economic Research Journal 12, 755–769. https://doi.org/10.19030/iber.v12i7.7968
Hicks, J., 1935, ‘The theory of monopoly’, Econometrica 3, 1–20. https://doi.org/10.2307/1907343
Isik, I. & Hassan, K.M., 2003, ‘Financial deregulation and total factor productivity change: An empirical study of Turkish commercial banks’, Journal of Banking and Finance 27(8), 1455–1485. https://doi.org/10.1016/S0378-4266(02)00288-1
Kaparakis, E., Miller, S. & Noulas, P., 1994, ‘Short-run cost inefficiency of commercial banks: A flexible stochastic frontier approach’, Journal of Money, Credit and Banking 26, 875–893. https://doi.org/10.2307/2077953
Karim, M.Z.A., Chan, G.S. & Hassan, S., 2010, ‘Bank efficiency and non-performing loans. Evidence from Malaysia and Singapore’, Prague Economic Papers 2, University of Economics, Prague.
Koetter, M. & Porath, D., 2007, ‘Efficient profitable and safe banking, an oxymoron. Evidence from a Panel VAR Approach’, Deutsche Bundesbank Discussion Paper Series 2, Banking and Financial Studies No 02/2007, Deutsche Bundesbank, Frankfurt.
Koetter, M., Kolari, J.W. & Spierdijk, L., 2012, ‘Enjoying the quiet life under deregulation. Evidence from adjusted Lerner indices for U.S. banks’, The Review of Economics and Statistics 94(2), 462–480. https://doi.org/10.1162/REST_a_00155
Leibenstein, H., 1966, ‘Allocative efficiency versus X-efficiency’, American Economic Review 56, 392–415.
Leon, F., 2015, ‘What do we know about the role of bank competition in Africa?’, Serie etudes et documents number 16, Ministère des Solidarités et de la Santé, Paris.
Maudos, J. & Solis, L., 2009, ‘The determinants of net interest income in the Mexican banking system: An integrated model’, Journal of Banking & Finance 33, 1920–1931. https://doi.org/10.1016/j.jbankfin.2009.04.012
Mason, E., 1939, ‘Price and production policies of large-scale enterprise’, The American Economic Review 29(1), 61–74.
Maredza, A. & Ikhide, S., 2013, ‘The impact of the global financial crisis on efficiency and productivity of the banking system in South Africa’, ERSA Working Paper Series 328 ERSA, Cape Town.
Maredza, A., Kapingura, F. & Mishi, S., 2013, ‘Exploring the nexus between bank competition and productivity in the South African banking sector’, Economic Research Southern Africa, viewed from https://econrsa.org/workshops/papers/2013/exploring-nexus-between-bank-competition-and-productivity-south-african
Mlambo, K. & Ncube, M., 2011, ‘Competition and efficiency in the banking sector in South Africa’, African Development Review 23, 4–15. https://doi.org/10.1111/j.1467-8268.2010.00268.x
National Treasury, 2017, Media release-competition commission findings on the banks…, viewed April 2018, from www.treasury.gov.za/…/2017021701%20-%20Media%20Statement%20Competition.
Ncube, M., 2009, Efficiency of the banking sector in South Africa, University of the Witwatersrand, South Africa.
Oberholzer, M. & Van der Westhuizen, G., 2004, ‘An empirical study on measuring efficiency and profitability of bank regions’, Meditari Accountancy Research 12(1), 165–178. https://doi.org/10.1108/10222529200400009
Okeahalam, C.C., 2006, ‘Production efficiency in the South African banking sector: A stochastic analysis’, International Review of Applied Economics 20(1), 103–123. https://doi.org/10.1080/02692170500362819
Peltzman, S., 1977, ‘The gains and losses from industrial concentration’, Journal of Law and Economics 20, 229–263. https://doi.org/10.1086/466902
Petersen, M. & Rajan, R., 1997, ‘The effect of credit market competition and lending relationships’, The Quarterly Journal of Economics 110, 407–443. https://doi.org/10.2307/2118445
Podpiera, A.P., Weill, L. & Schobert, F., 2008, ‘Banking competition and efficiency: A micro-data analysis on the Czech banking industry’, Comparative Economic Studies 50, 253–273. https://doi.org/10.1057/palgrave.ces.8100248
Rahim, A.B.R., 2016, ‘Does competition foster efficiency? Empirical evidence from Malaysian commercial banks’, Asian academy of management Journal 12(1), 1–23.
Rosenthal, R., 1980, ‘A model in which an increase in the number of sellers leads to a higher price’, Econometrica 48(6), 1575–1579. https://doi.org/10.2307/1912828
Saka, A.N.A., Aboagye, A.Q.Q. & Gemegah, A., 2012., ‘Technical efficiency of the Ghanaian banking industry and the effects of the entry of foreign banks’, Journal of African Business 13(3), 232–243.
Sarpong-Kumankoma, E., Abor, J. & Aboagye, A.Q.Q., 2017, ‘Freedom, competition and bank efficiency in sub-Saharan Africa’, International Journal of Law and Management 59, 1359–1380. https://doi.org/10.1108/IJLMA-11-2016-0142
Schaek, K. & Cihak, M., 2008, ‘How does competition effect efficiency and soundness in banking: New empirical evidence’, European Central Bank Working Paper Series Number 932, European Central Bank, Frankfurt.
Simatele, M., 2015, ‘Market structure and competition in the South African Banking sector’, Procedia Economics and Finance 30, 825–835. https://doi.org/10.1016/S2212-5671(15)01332-5
Simbanegavi, W., Greenberg, J.B. & Gwatidzo, T., 2015, ‘Testing for competition in the South African Banking Sector’, Journal of African Economics 24, 303–324. https://doi.org/10.1093/jae/eju022
South African Reserve Bank, 2017, viewed October 2017, from https://www.resbank.co.za/RegulationAndSupervision/BankSupervision/Pages/SouthAfricanRegisteredBanksAndRepresentativeOffices.aspx.
Statistics South Africa, 2017, viewed October 2017, from http://www.statssa.gov.za/.
Stiglitz, J., 1989, ‘Imperfect information in the product market’, in R. Schmalensee & R. Willig (eds.), Handbook of industrial organization, vol. I, Elsevier Science Publishers, Amsterdam.
South African Reserve Bank, 2018, Financial stability review, viewed April 2018, from https://www.resbank.co.za/Publications/Reviews/Pages/FinancialStabilityReview.aspx.
Tabak, B.M., Fazio, D.M. & Cajueiro, D.O., 2012, ‘The relationship between banking market competition and risk-taking: Do size and capitalization matter?’, Journal of Banking & Finance 36(12), 3366–3381. https://doi.org/10.1016/j.jbankfin.2012.07.022
Van Leuvensteijn, M., 2008, ‘The Boone indicator: Identifying different regimes of competition for the American Sugar Refining Company 1890–1914’, Tjalling C. Koopmans Research Institute, Discussion Paper Series nr 08-37, University of Utrecht, The Netherlands.
Vivas, A.L., 1997, ‘Profit efficiency for Spanish savings banks: Accounting for heterogeneity is important’, European Journal of Operational Research 98, 381–394. https://doi.org/10.1016/S0377-2217(97)00354-8
Weill, L., 2004, ‘Measuring cost efficiency in European banking: A comparison of frontier techniques’, Journal of Productivity Analysis 21(2), 133–152. https://doi.org/10.1023/B:PROD.0000016869.09423.0c
Williams, J., 2004, ‘Determining management behaviour in European banking’, Journal of Banking and Finance 28, 2427–2460. https://doi.org/10.1016/j.jbankfin.2003.09.010 Appendix starts on the next page →
Appendix 1
TABLE 3-A1: Bank stability results using non-performing loans. |
Footnotes
1. The South African Reserve Bank defines bank stability as the absence of the macro-economic costs of disturbances in the system of financial exchange between households, businesses and financial service firms. A sound banking system ensures the optimal allocation of capital resources, and efficient management of risks to prevent costly banking system crises and their associated adverse feedback effects on the real economy.
2. This, however, also depends on which efficiency hypothesis, as discussed in the first part of the introduction, characterises what is happening in the South African banking sector.
3. For more information on these structural and non-structural measures and their shortcomings, see Leon (2015).
4. Total revenue is equal to total interest and non-interest income
5. This approach of determining inputs and outputs variables is based on the intermediation approach. In this approach banks are treated as collectors of funds, which are then intermediated to loans and other assets. The total balance of deposits and loans is used as a measure for outputs, while operating and interest costs are used to measure total costs. In the production approach a bank is viewed as a producer of deposits and loans using labour, capital and materials. According to Kaparakis, Miller and Noulas (1994), this approach seems more appropriate when the sample contains large banks, who fund a larger share of their assets from non-deposit sources. Berger and Humphrey (1997) suggest that the intermediation approach is best suited for analysing firm level efficiency, while the production approach is suited for measuring branch level efficiency, as at this level employees have little influence over funding and investment decisions (Ncube 2009).
6. The criticisms of the standard Lerner index is that it implicitly assumes banks are fully efficient. Koetter, Kolari and Spierdijk (2012) show that by ignoring both cost and profit inefficiencies, the Lerner index would lead to an even larger bias in price-cost margin, as well as in consumer and producer welfare losses. They propose a correction in the form of an efficiency adjusted Lerner index.
7. Van Leuvensteijn (2008) found empirical support for the Boone indicator using American sugar industry data, while Schiersch and Schmidt-Ehmcke (2010) used German manufacturing data and showed that the Indicator fails to correctly indicate competition compared to the traditional Lerner index.
8. There are no a priori reasons for choosing one distributional form over another. All have advantages and disadvantages but the exponential and half-normal have a mode of zero ideal when many firms are perfectly efficient and are easy to compute compared to the truncated normal and gamma distributions (Coelli, Rao & Battese 1998).
9. Berger & Mester (1997) consider the profit efficiency concept to be superior to the cost efficiency concept for evaluating the overall performance of a firm. Firstly, profit efficiency is based on profit maximisation, which requires that the same amount of focus is placed on maximising marginal revenue so as to reduce marginal costs. Secondly, the profit function deals with both input and outputs inefficiencies while the cost function accounts for only inefficiencies in inputs (Vivas 1997). Finally, a bank can be inefficient if it produces too few, or a nonoptimal mix of, outputs given the inputs it uses and the prices it faces. As highlighted by Isik and Hassan (2003), ‘cost efficiency models ignore this possibility and thus can misrepresent the nature and extent of efficiency of banks’.
10. We used the bank size mean and bank age mean to divide the banks into small and large, as well as older and younger respectively. The mean size using total assets was R173 100 million and the mean age was 52 years. There are no studies in South Africa that looked at these bank level characteristics to compare with.
11. These results are not included here but are available on request.
12. We also ran another set of GMM models using lags of different efficiency variables and this did not change the general sign of the two competition indicators. The Lerner index was however significant only when using cost efficiency but insignificant with profit efficiency.
|