Abstract
Background: Exporting poses a challenge to the achievement of inclusive growth because there is a discernible wage inequality between exporting and non-exporting firms. The literature shows that exporting firms pay a wage premium relative to non-exporting firms, with the resultant wage gaps having widened over the years in line with expanding global trade.
Aim: Limited research has been done on the distribution of wages within manufacturing exporting firms relative to non-exporting firms in South Africa and how wage differentials might contribute to wage inequality. This article disentangles these wage differentials using administrative firm-level panel data.
Setting: Exporting and non-exporting firms in the South African manufacturing sector.
Methods: By determining the wage differential in a firm at various percentiles, it is found that all employees (across the wage distribution) in an exporting firm earned a wage premium. This premium seemed to increase in magnitude towards the upper tail of the distribution, indicating that the wage differential did contribute to wage inequality.
Results: Much of the wage inequality could be explained by the size and labour productivity of a firm. This implies that larger, more productive firms are more likely to be exporters, whereas there was little evidence that wage inequality is driven by either the type of destination country or the quality of export products.
Conclusion: The findings suggest that the resultant wage inequality is related to the process of exporting or simply a firm being in the export market. Alternatively, wage inequality could be attributable to a specific type of firm (employing a specific type of person with sought-after skills) that had this (unequal) wage distribution before it started to export.
Keywords: Exporters; firm-level administrative data; wage premium; wage inequality; wage distribution; South Africa; inclusive growth.
Introduction
Although exports have been identified as an engine for inclusive growth, they also pose challenges as far as such growth is concerned, since they create a type of wage inequality that can be discerned between exporting and non-exporting firms. This is because the most productive firms (where wage inequality is most acute) are more likely to be exporters. Exporting firms have been shown in the literature to pay a wage premium relative to non-exporting firms. Moreover, this wage differential between exporting and non-exporting firms has contributed to an increase in wage inequality in developed and developing countries alike (Krugman 2008). Moreover, this wage gap (between the wages of exporters and non-exporters) has been widening as global trade has expanded over the last few decades (Klein, Moser & Urban 2013), which calls into question the ability of exports to foster inclusive economic growth, especially in developing countries like South Africa.
South Africa’s reintegration into the world economy and trade liberalisation process since the 1990s (Edwards 2001) constitute an interesting case study, as they have taken place during a period in which income inequality has been steadily increasing – so much so that South Africa is one of the world’s most consistently unequal countries, with an income Gini coefficient ranging between 0.66 and 0.7 (World Bank 2017). Van der Berg (2011) decomposed South Africa’s income inequality by income source and found wage income to be the primary component in income inequality. Therefore, a large part of the unequal Gini coefficient relates to an uneven distribution of wages, indicating wage inequality.
In the light of the above, this article investigates how South Africa’s participation in the global market (more specifically, the country’s export participation from 2010 to 2014) affects the wage distribution within the manufacturing sector. South Africa experienced an average overall export growth of 14% for the period 2010–2014 (Trademap 2020). During this period South African manufacturing exporters’ behaviour is similar to the stylised findings in the international literature (i.e. exporting firms employ more people and pay higher wages than non-exporters). However, only limited work has been done on how these higher wages are distributed within manufacturing exporting firms, relative to non-exporting firms (e.g. do all employees earn a wage premium or only some of them?) and how this wage differential contributes to wage inequality.
The international trade literature reveals that wage inequality is usually studied in terms of the skills premium (which relates to the wages of skilled and unskilled labour being higher in an exporting firm than in a non-exporting firm) (Bernard & Jensen 1997; Brambilla, Lederman & Porto 2012; Klein et al. 2013). The firm-level data used in this study do not contain variables on education or skills level. However, the data allowed for the calculation of the average wage within a firm at the 5th, 25th, 50th, 75th and 95th percentiles (i.e. the within-firm wage distribution). Therefore, instead of comparing the average or median wages of exporting versus non-exporting firms, one can compare how wages are distributed within an exporting firm, relative to a non-exporting firm. Wage inequality can therefore be illustrated through this within-firm wage distribution, where a more unequal distribution of wages thoughout the percentiles shows higher wage inequality.
This article contributes to the limited literature by firstly disentangling the wage differentials between non-exporters and exporters, as well as within exporters (which includes a consideration of exporter dynamics and destinations served). In short, it studies within-firm wage distributions and wage inequality. There are only a limited number of international studies that have considered the within-firm wage distribution phenomenon (see, for example, Bernini, Guillou & Treibich 2015). Secondly, the article examines possible sources of wage inequality by analysing firm characteristics, the type of product exported and the type of destination served. The article uses detailed longitudinal tax administrative data (made available by the South African Revenue Service [SARS]) to do a precise evaluation of the link between a firm’s export status, its within-firm wage distribution and wage inequality. A panel data set from 2010 to 2014 was created by linking three sets of administrative data, namely the company’s income tax (CIT) data, employee data (IRP5) and customs data.1
Literature overview
Empirical literature overview
The wage differential between exporting and non-exporting firms is one of the sources of the increase in wage inequality within countries (Krugman 2008). Moreover, this gap (between the wages of exporters and non-exporters) widens as global trade expands (Klein et al. 2013). This implies that the wage inequality between exporters and non-exporters grows as international trade increases. The link between (the rising) wage dispersion and trade has been examined in numerous countries, for example Germany (Klein et al. 2013), the United States (US; Bernard & Jensen 1997), Mexico (Verhoogen 2008) China (Fu & Wu 2013) and France (Bernini et al. 2015).
A study by Bernard and Jensen (1997) investigated the increase in wage inequality among exporting and non-exporting firms. They found that a large part of the rise in wage inequality in the US manufacturing sector (in the 1980s) was due to exporting firms’ increased demand for skilled labour. Therefore, relative to non-exporting firms, exporting firms needed to pay these ‘skilled workers’ higher wages (which is also known as a ‘skills premium’). Bacchetta et al. (2017) also discussed the skills premium and its link to wage inequality. In a paper on investing in skills for inclusive trade, they explained that the demand for and supply of skills in the context of trade can affect the wage distribution (and thus wage inequality). If the skills supply is inadequate for the demand (i.e. there is a skills mismatch), then the price of skilled jobs increases and there is an increase in inequality. There is risk of further polarisation of the wage distribution if the demand for middle-paid jobs decreases in relation to high-paid, as well as low-paid jobs. On the other hand, if the skills supply meets the increased demand, then there is a less discernible link between trade and wage inequality, and overall labour market outcomes improve.
Similar to Bernard and Jensen (1997) and Bacchetta et al. (2017), Klein et al. (2013) also considered the skills premium, but used German manufacturing employer-employee level data to compare the wages of different skills groups employed by exporters. They found that significant export wage dispersion existed among highly skilled workers (earning a ‘wage premium’) and low-skilled workers (earning a ‘wage discount’). Moreover, 30% of wage inequality (within and between skills groups) could be ascribed to exporting firms. In a similar study in Mexico, Verhoogen (2008) found that the devaluation of the peso (during the peso crisis of 1994) led to within-industry wage dispersion. Moreover, the more productive exporting firms in Mexico were able to withstand the crisis and increased their exports (both in terms of quality and wages), while the less productive firms were unable to do so, which resulted in within-industry wage dispersion.
It is important to consider not only the difference in the average wages of firms (using ordinary least squares [OLS] regressions) but also the wage differences at different quantiles or percentiles of the wage distribution (using either quantile regressions or OLS regressions at different percentiles). As stated by Fu and Wu (2013):
… if more talented and high-ability workers would tend to be hired by exporting firms, the average wage of exporting firms would be driven up and the export wage premia would be overestimated. (p. 185)
Recently, the availability of more detailed data has allowed for the consideration of the wage differential (across the wage distribution) on both a firm level (i.e. the firm’s distribution of wages – see Bernini et al. 2015) and a job level (i.e. the overall wage distribution in a sector – see Fu &Wu 2013).
A noteworthy study by Bernini et al. (2015) focused on the within-firm wage distribution (firm level) of exporters versus non-exporters. Their results showed a wage premium right across the distribution (from the 10th to the 90th percentiles). This premium seemed to increase towards the top end of the distribution. Furthermore, they showed that this premium increased with the export intensity of the firm.
Bernini et al. (2015) considered not only the firm-level distribution of wages (to compare firms) but also the job level (employee level) distribution of wages in order to study the distribution of wages for all employees. They found that, across the wage distribution, the wages of those working in exporting firms were predominantly higher than those working in non-exporting firms. Fu and Wu (2013) also considered the overall wage distribution (at the job level) of the Chinese manufacturing sector in 2004. They found an export wage premium (specifically among firms defined as state-owned enterprises originating from countries in the Organisation for Economic Cooperation and Development [OECD]) across the entire wage distribution. This wage premium seemed to decrease as one moved from the lower tail to the upper tail of the distribution (while controlling for firm characteristics, e.g. labour productivity, size, age and capital intensity, female share of total employees, workers with graduate degrees, college degrees or highschool certificates, industry and province).
Even though workers in exporting firms earn higher wages (across the distribution), this is not to say that all exporting firms are the same (and pay the same premium). Exporters themselves are heterogeneous in nature. This can be seen in terms of the location of the firm (Fu & Wu 2013), productivity levels (Bas 2012; Bernard, Jensen & Schott 2009), number of products exported, number of destinations served (Bernard et al. 2009), type of destination served and product quality (Brambilla et al. 2012; Brambilla & Porto 2016; Verhoogen 2008).
Exporter heterogeneity in terms of firms’ location influences the wages they pay. In their study on Chinese manufacturing exporters, Fu and Wu (2013) showed that the wages of Chinese exporters differed depending on the firm’s location. Firms that were situated along the coast paid higher wages than those that were located inland. Exporters are also heterogeneous in terms of their level of productivity. A plant level study on the productivity of Chilean manufacturing exporters revealed a difference between the upper range exporters and lower range exporters within the productivity distribution (Bas 2012). The exporters in the upper range used high technology and were more skill-intensive, and therefore paid higher wages than the lower range exporters. Another study considering the productivity levels of exporters (specifically US manufacturing exporters) showed that the more productive firms (that paid higher wages) were the ones that exported multiple products (i.e. they were multi-product exporters) to multiple destinations (multi-destination exporters) (Bernard et al. 2009). Furthermore, the more products exported and destinations served, the higher the wage and employment levels were (Bernard et al. 2009).
It is not only the number of destinations that influences an exporting firm’s wages, but also the ‘type’ of destination. There is a link between the type of destination country (high, middle or low income), the product quality required, the type of worker who can manufacture it and the worker’s wages (Brambilla et al. 2012; Brambilla & Porto 2016; Verhoogen 2008). Verhoogen (2008) studied Mexican manufacturing exporters for the periods 1993–2001 and 1984–2001. His results showed that when firms exported to high-income countries, their products needed a quality upgrade, which required more skilled workers. In a similar vein, in a study conducted by Brambilla et al. (2012) there was a so-called ‘skills bias’ in export destinations for Argentinian manufacturers. This ‘skills bias’ was evident in the fact that exports to high-income countries (relative to middle-income countries or their domestic market) necessitated higher worker skills levels, which in turn implied higher wages (Brambilla et al. 2012). A more recent study by Brambilla and Porto (2016) confirmed this. In their study of 82 countries they showed that high-income countries demanded higher-quality goods (and firms exporting to these destinations subsequently paid higher wages).
The following section focuses on the South African literature on the wage differential between exporting and non-exporting firms.
South African literature overview
The South African literature on the within-firm wage distribution of exporting and non-exporting firms is very limited. However, the literature does reveal a wage differential between exporting and non-exporting firms (Bhorat et al. 2017; Edwards, Sanfilippo & Sundaram 2016; Matthee et al. 2016; Rankin 2001, 2013; Rankin & Schoër 2013). This wage differential seems to vary by type of exporter.
By using newly available administrative data on South African manufacturing firms, Bhorat et al. (2017) measured the contribution of firm and individual level characteristics to South Africa’s wage formulation. They found that approximately 61% of wage variance is due to individual effects, while at least 13% is due to firm-level effect. It is typically firms that are profitable, older, more capital-intensive, more productive and involved in international trade that pay higher wages, on average. A paper by Bhorat et al. (2017) shed some light on the theoretical underpinnings of why wages differ within firms. It is clear that wage differences within firms are strongly related to basic measures of productivity. Furthermore, Bhorat et al. (2017) illustrate that there is a direct relationship between wages and capital intensity. Moreover, they show that the less important labour costs are to capital costs, the greater the possibility of workers extracting rents (Bhorat et al. 2017). A high capital to labour ratio may be indicative of high fixed costs and barriers to entry and hence product market power. These product market power rents may be shared between workers and may be asymmetrical across the wage distribution.
Edwards et al. (2016) identified a wage premium among firms involved in international trade. They further distinguished between the wages of three types of traders, namely exporter only, importer only and two-way trader. They showed that the wage premium was the highest for two-way traders, followed by importers only and then exporters only.
By using the same data as Edwards et al. (2016), Matthee et al. (2016) confirmed the export wage premium in South Africa, showing that exporters paid 31% higher wages than non-exporters (even after controlling for firm size). They further distinguished between different types of exporter in terms of the number of products they exported (multiple-product versus single-product exporters), the number of destinations they served (multiple-destination versus single-destination exporters) and the market they served (Africa or non-Africa). Their results showed that multiple-destination exporters paid higher wages than single-destination exporters. The same was true for multiple-product exporters (paying higher wages than single-product exporters). Furthermore, they showed that exporting firms serving only the African market paid higher wages than domestic firms, but it was the exporters serving both African and non-African countries that had the highest export wage premium (Matthee et al. 2016).
In a similar vein, Rankin (2001; 2013) and Rankin and Schoër (2013) classified South African exporters into two groups, namely those who serve regional (lower-income) markets and those who serve international (high-income) markets. Rankin (2001) showed that firms exporting to countries outside of the Southern African Development Community region pay the highest wages. In a later study, Rankin (2013) confirmed these findings by also grouping South African exporters into two markets served, namely those who export to high-income markets (Europe and North America) and those who export to lower-income markets (Africa). The firms exporting to high-income markets pay higher wages than those exporting to lower-income markets (which pay even less than non-exporters). Another study by Rankin and Schoër (2013) related this phenomenon to product quality and worker quality. Moreover, the high-income destinations require higher-quality products, which are manufactured by more skilled (high-quality) workers who are paid a skills premium (Rankin & Schoër 2013).
The link between trade and skills was also investigated by Edwards (2001). He found that trade liberalisation had resulted in a skills bias. Edwards (2001) analysed the impact of trade and structural change on employment in South Africa from 1984 to 1997. Even though the study was not on a firm level, the results showed that technological change had brought about a rise in demand for high-skilled employment and a decrease in the demand for ‘elementary-skilled employment’ (Edwards 2001:54). Furthermore, Edwards (2001) showed that there was also a skills bias in the manufacturing export sector. Therefore, growth in trade will more likely create skilled jobs which are paid a skills premium, adding to wage inequality.
Even though South African exporters are heterogeneous in terms of the number of products they export, the number of destinations they serve and the types of destination they serve, it is clear that these exporting firms pay higher wages. However, limited research has been conducted on how these higher wages are distributed within the exporting firm (e.g. do all employees earn a wage premium or only some of them?) and how the wage gap contributes to wage inequality. This article therefore set out to evaluate the link between export participation and the within-firm wage distribution.
Empirical analysis
Data
The availability of detailed, longitudinal administrative data in the international literature has recently cleared the way for a more precise evaluation to be done of the link between wages (more specifically the within-firm wage distribution) and export status (Bernini et al. 2015). This article made use of South African tax administrative data (from SARS) with a view to investigating the link between a firm’s export status, wage distribution and wage inequality. In order to do this, a panel data set was created by linking three sets of data, namely the CIT data, employee data (IRP5) and customs data.
The first data set (i.e. the CIT data) contained the balance sheets and income statements of firms. From the CIT, the following variables describing firm characteristics were obtained: property, plant and equipment (which measure capital intensity), gross income (which measures sales and output) and the profit code classifier or main industry code (which identifies the sector in which a firm operates). The profit code classifier was condensed to the four-digit International Standard Industrial Classification (ISIC) used by SARS to select the firms in the manufacturing sector (ISIC four-digit codes 1010–1033). It is important to note that the form that firms use to complete their income tax return changed in May 2013. The IT14 was replaced by the ITR14. With the transition from the IT14 to the ITR14, it became evident that some firms completed the IT14 and others completed the ITR14; therefore, these two forms were merged to form a panel of data.
The second data set used in this article (the IRP5 data) contained the IRP5 certificates of employees completed by or on their behalf by their employers. Hence, the data are reported per job (a unique employer [firm]–employee [individual] combination). The IRP5 contains information on the period that an individual worked in a specific job (in days), their income and their birth date. The raw IRP5 data was adjusted to remove duplicate certificates, multiple job spells and invalid periods worked (see Table 1-A1 in Online Appendix 1). Two variables were created from the IRP5 data, namely the number of employees per firm and the within-firm distribution of wages. Firstly, the weighted number of employees per firm was created by calculating the length of employment (in days) for each worker within a year, arriving at a total within the firm and dividing by 365. The aim was essentially to generate the stock of worker inputs into production per firm for the whole year. Secondly, the within-firm distribution of wages was created in two steps. Step one involved generating the weighted monthly wages per worker by taking the income and dividing it by the number of days worked (to get the daily wage equivalent), and then multiplying this by 30 to get the monthly equivalent of wages. Step two involved taking the weighted monthly wages per person for each firm and measuring the distribution of wages within the firm (i.e. measuring the average wage in the firm at the 5th, 25th, 50th, 75th and 95th percentiles).
The third and final data set used in the article (the customs transaction data) reported the export transactions of South African firms on a monthly basis. From this database, the following variables were obtained: trader identifier (firm identifier), tariff code (product code on HS6-digit level), country of destination (market), country of origin (South Africa), customs value of the transaction (South African rand) and the statistical value (number of units). Two adjustments were made to the raw customs data. Firstly, duplicate transactions were removed and, secondly, only exporters trading more than R10 000 per year were kept in the data set (which, however, still covered 99% of export data).
In the empirical analysis sections of this article, the customs data were utilised on two different levels (on firm level and on transaction level). For the within-firm wage distribution and inequality section, the customs data were used to create the following firm-level variables: dummy variables to indicate if a firm was an exporter (or not), the destination to which it exported (South African Customs Union2 only, Africa only or international), exporter dynamics (if a firm entered,3 exited4 or continued5 in the export market), the number of products (on HS6-digit level) a firm exported and the number of destinations served. When considering the possible sources of wage inequality (namely the type of product exported and destination served), transaction level data were used, which allows one to control for the type of product being exported (HS6-digit product code), its price and the gross domestic product (GDP) per capita6 of the destination country. The product price per firm was calculated in two steps:
Step 1: 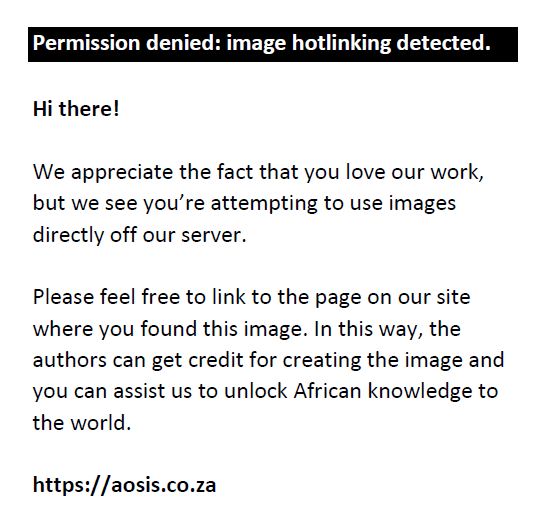
Step 2: 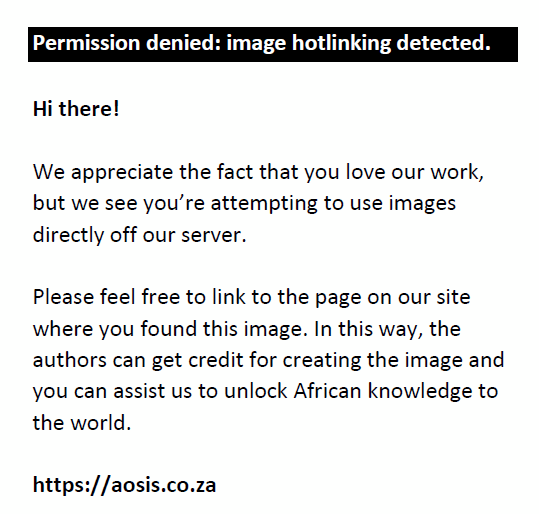
Step one involved taking the customs value per transaction and dividing by its statistical quantity (to get the HS6 price). Step two involved determining the difference between the HS6 price and the average price of all products with the same HS6 code (this provides the price as a measure of the deviation from the average price per product).
To create a panel containing all three data sets, a conjunction table (provided by SARS) was used to link the various data sets through different firm identifiers. As all three data sets worked on different reporting years or periods, the dates of the data sets were aligned. After these adjustments were made, the panel data set on South African manufacturing firms, spanning the period 2010–2014, was completed.
Descriptive statistics on manufacturing exporters and their characteristics
An overview of the data is provided in the form of an illustration of the average number of firms in the manufacturing sector from 2010 to 2014 and these firms’ export status. From Figure 1 it is clear that, on average, 21% of all manufacturing firms export. These exporters are heterogeneous in the markets they serve, as 5% of these firms export only to SACU, 7% export only to other African countries (i.e. excluding SACU exporters) and 9% export to international markets (these firms export to both African and non-African countries).
 |
FIGURE 1: The average number of manufacturing non-exporters and exporters (serving different destinations) from 2010 to 2014. |
|
Exporters differ not only in terms of the markets they serve, but also in terms of their export dynamics (i.e. a firm can enter into, continue in or exit the export market). Figure 2 illustrates the average number of manufacturing exporters in each export dynamic group per export destination. It is clear that, on average, 64% of firms continue to export year on year. Furthermore, an average of 29% of firms enter into the export market each year and only 7% exit.
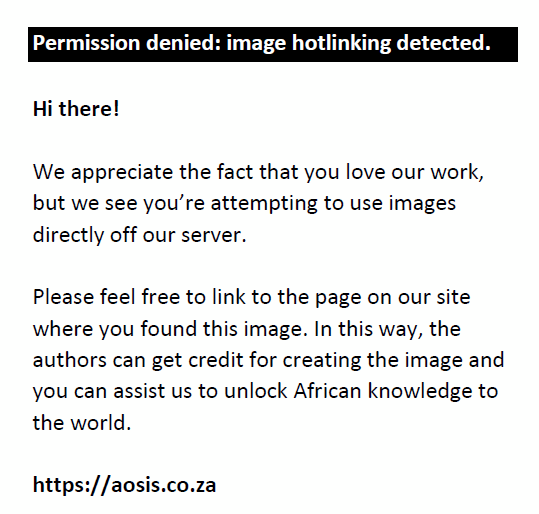 |
FIGURE 2: Exporter dynamics per destination (average number of firms from 2010 to 2014). |
|
It is interesting to note that across all the dynamic groups, the number of firms exporting to African countries only is larger than the number of firms exporting to the international market (exporting to both African and non-African countries). A possible reason for the larger number of firms in the African market is the size, competitiveness and proportion of output exported by these firms (i.e. there are large numbers of small, less competitive firms exporting a small proportion of their output to Africa). Moreover, the literature suggests that a large share of South African firms export, but only a few firms specialise in exporting (the top 5% South African exporters export 85% of the total export value) (Naughtin & Rankin 2014). The firms exporting to Africa most likely form part of the remaining firms that export only a small amount of their output and are no more productive than domestic firms (Rankin 2001). Table 1 confirms that it is the smaller firms (in terms of number of employees) that export to the African market only (relative to the firms exporting to the international market). This suggests that the threshold for entering the Africa-only market is lower, and consequently a larger number of (smaller) firms are able to enter and remain in this market.
TABLE 1: Characteristics of different firms (serving domestic, African and non-African countries). |
Table 1 provides more detail on the characteristics (number of employees, capital per worker and output per worker) of firms entering, continuing in or exiting the international or African markets. Relative to non-exporters and African exporters, continuing and entering international exporters are larger (in terms of number of employees and capital per worker) and more productive (in terms of output per worker). Even though smaller than international exporters, all (continuing, entering or exiting) African exporters have a larger number of employees, more capital per worker and larger output per worker than non-exporting firms.
It is also important to take note of the wages (per person) paid to workers in the different types of exporting firms, as well as to consider the within-firm wage distribution (i.e. consider the average monthly wages per person paid at the 5th, 25th, 50th, 75th and 95th percentiles of a firm’s wage distribution). Figure 3 provides a visual representation of the within-firm wage7 distribution. From Figure 3 it is clear that the average wages from the 5th to 95th percentiles increase with the distance of the markets that these exporters serve (first serving the domestic market, followed by exporting to SACU countries only, then exporting to African countries only and finally also exporting to international markets). The hierarchy appears to be across all parts of the distribution and is larger at the top end. This, together with the increase in the interquartile range (at the right-hand side of the graph), suggests that the wage inequality within firms increases as the market served gets further away.
 |
FIGURE 3: Wage distribution: non-exporters versus exporters (serving African and non-African countries) average from 2010 to 2014. |
|
The next section analyses the wage distribution within these heterogeneous firms and investigates possible sources of wage inequality.
Regressions on within-firm wage distribution and possible sources of wage inequality
From Figure 3 it is apparent that there is a wage differential and wage inequality between firms that serve different markets (first serving the domestic market, followed by exporting to SACU countries only, then exporting to African countries only, and finally also exporting to international markets). However, as Figure 3 simply represents wage levels, the observed inequality should be investigated further by means of regressions with various control variables. To this end, the following sections consider the within-firm wage distribution to which different controls (in terms of firm characteristics) are added, with a view to shedding light on the possible sources of the wage differential and wage inequality, and the characteristics of the destination economy (proxied by GDP per capita) and quality of exports (proxied by the price of the manufactured export product) as other possible explanations for the wage inequality.
Within-firm wage distribution and inequality
Estimation strategy: Within-firm wage distribution and inequality
The wage differential and wage inequality between exporters (serving different destinations) and non-exporters observed in Figure 3 could be due to exporters just being different types of firms (for example, larger and more capital-intensive firms serving different industries). One way to compare ‘like-for-like’ is to estimate regressions with control variables. Consequently, to examine the within-firm wage distribution of exporters (with different dynamics and destinations) relative to non-exporters (with control variables), this article followed the methodology of Bernard and Jensen (1997) in measuring the export premia (in terms of monthly wages). This was done by estimating regressions of the general form:
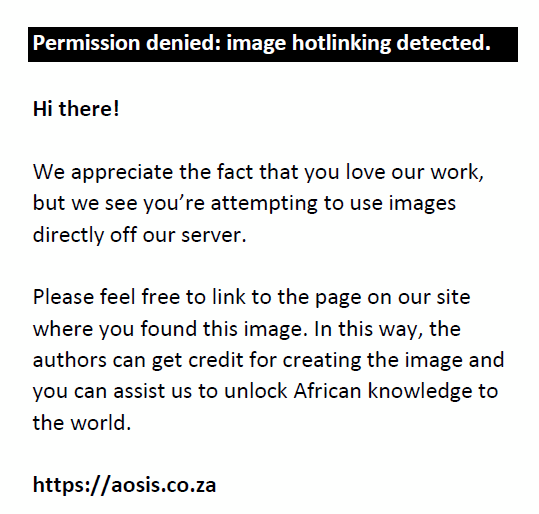
In Equation 1, ln (X)it is the logarithm of monthly wages earned by workers at each percentile of the firm’s wage distribution (5th percentile, 25th percentile, 75th percentile and 95th percentile), as well as the standard deviation and interquartile range of these wages per firm. The ‘Expoteri’ is a dummy variable indicating the export status of a firm. The export status can vary between a non-exporter and an exporter exporting to Africa, SACU or the international market. Furthermore, export status can also indicate a firm’s dynamics (enter, exit or continue). The number of destinations (No.destit) to which a firm exports and the number of products (No.prodit) it exports are also accounted for (in the case of non-exporting firms this variable is simply a zero). Real capital per worker (lklit) is also included in the equation. Equation 1 further adds two control dummies. The first is for the industry (Industryit) in which the firm operates (classified according to the four-digit ISIC code) and the second is for the years (2010–2014) in the panel (yeari). Finally, the export premia (of the wages) are represented by (βit), as well as the error term (µit) (also see Table 3-A1 of Online Appendix 1 for a detailed description on the regression variables).
Even though Equation 1 takes into account the capital per worker (lklit) in a firm (in real terms), other firm characteristics could influence the wages that a firm pays to workers in different parts of its wage distribution. Equation 2 expands on the model (Equation 1) by adding firm characteristics that measure firm size and labour productivity:
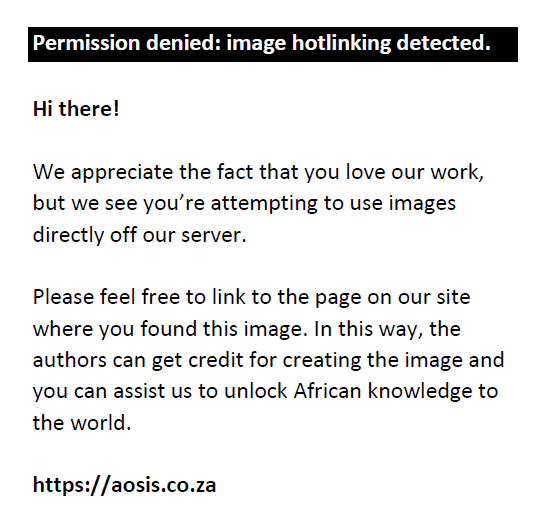
In Equation 2 (llit) is the natural log of the number of employees which measures firm size, whereas (lklit) is the natural log of the output per worker (in real terms) which serves as a measure of labour productivity. By adding these two controls, it is possible to determine the impact of firm size and labour productivity on wages (and the wage distribution in a firm) when Equation 1 and Equation 2 are compared.
Results: Within-firm wage distribution and inequality
Table 2 shows the distribution of wages between exporting firms serving different destinations relative to non-exporters (by estimating Equation 1, i.e. controlling for the number of destinations, number of products, capital per worker, industry and year). Right across the distribution (from the 5th to the 95th percentiles) there is a hierarchy in the wage premium for firms, the further away the destination is (i.e. SACU-only exporters’ wages are lower than Africa-only exporters’ wages, which are in turn lower than international exporters’ wages). For example, at the 25th percentile the wage premium for SACU-only exporters is 9.23% lower than that for Africa-only exporters, which is in turn 6.8% lower than that for international exporters. Therefore, a job at an international firm pays higher wages, but what about the wage inequality in these firms?
TABLE 2: Wage distribution: Firms serving domestic, African and non-African countries. |
The wage inequality can be seen by considering the standard deviation and interquartile range in the last two columns of Table 2. It is clear that (similar to the wage premium) the standard deviation and interquartile range also increase as the export destination becomes more distant. Therefore, a job at an international firm pays better (are better jobs), but there is higher wage inequality within these firms.
When considering the number of products a firm exports and the number of destinations to which they export, it is clear that both the number of destinations and the number of products are more significant and have a bigger quantitative effect, as one moves from the lower tail to the upper tail of the distribution (from the 5th to the 95th percentiles). For example, exporting to one more destination increases the 95th percentile (2.0%) by more than it increases the 5th percentile (0.6%). It is also important to note that, with destinations, the wage effect of adding a destination is the largest at the 95th percentile while, with products, the wage effect is almost equal at each percentile. One of the arguments put forward in the literature as to why exporters pay more, is that they need more sophisticated management. For example, they may be exporting multiple products to multiple destinations which is a complex process that requires quality upgrades and the enhancement of operational services and skills. Exporters therefore need more sophisticated staff on the factory floor, but also greater management or technical expertise found in higher-skilled workers (which are seen at the upper tail of the distribution) (Brambilla, Chauvin & Porto 2015).
Figure 4 provides an illustration of the estimation of both Equation 1 (also see Table 2) and Equation 2. Therefore, Figure 4 plots the coefficient of the wage premium from the regressions on a line graph to illustrate the wage distribution within a firm. The difference between the two equations is that Equation 1 (represented by the solid lines) only controls for capital intensity (capital per worker) whereas Equation 2 (represented by the dotted lines) also controls for firm size (in terms of number of employees) and labour productivity (in terms of output per worker). The solid lines (results of Equation 1) show the within-firm wage distribution of international, African and SACU exporters relative to non-exporters. Here, the export wage premium is very clear. This observed premium also increases in magnitude towards the upper tail (75th and 95th percentiles) of the within-firm wage distribution (which indicates wage inequality). When more firm characteristics are added (by estimating Equation 2), the distribution changes shape (which is represented by the dotted lines). Essentially, this shows that the wage inequality observed from the solid lines is mostly due to firm characteristics (i.e. different types of firms export internationally, compared to those that export regionally).
 |
FIGURE 4: Distribution of the coefficients of the wage premium: firms serving domestic, African and non-African countries, with different controls. |
|
As seen in the descriptive statistics, exporters do not only differ in terms of the markets that they serve, but also in terms of their export dynamics (i.e. a firm can enter, continue or exit the export market). Table 4-A1 and Table 5-A1 and Figure 5 focus on the wage distribution between exporters with different dynamics.
 |
FIGURE 5: Distribution of the coefficients of the wage premium: exporter dynamics (enter, exit and continue), with different controls. |
|
Comparing firms that enter the export market with those that continue to export or exit the export market, provides an indication of what happens to an exporting firm’s wage distribution over time. Table 4-A1 and Table 5-A1 present the results of estimating Equation 1 (with and without controls for the number of products and destinations). When controlling for the number of products and the number of destinations, Table 5-A1 shows that the distribution of wages within a firm is higher for entering firms than for continuing firms (from the 5th to the 75th percentile). Without the control (see Table 4-A1 in Appendix 1), the continuing firm’s wage distribution is higher (wider). Therefore, as firms continue exporting, they grow in terms of the number of products exported and the destinations served. Table 5-A1 also shows that when a firm enters the export market, the bottom 5th percentile, on average, earns 1.7% more than continuing firms (exporting the same number of products to the same number of destinations). However, when a firm enters the export market, the top 95th percentile on average earns 6.5% less than continuing firms. Therefore, relative to entering firms, continuing firms pay more as one moves to the upper tail of the distribution. However, there is not a big difference between entering and continuing firms. Overall, Table 4-A1 and Table 5-A1 suggest that actually prior to or within the first year of entry, firms already pay higher wages and have higher wage inequality right through the distribution.
Figure 5 provides a graphical illustration of estimating Equation 1 and Equation 2 (also see Table 2) for firms with different exporter dynamics. Equation 1 (represented by the solid lines) only controls for one firm characteristic, namely capital intensity (capital per worker), whereas Equation 2 also controls for firm size (in terms of number of employees) and labour productivity (in terms of output per worker). From the results of Equation 1 (represented by the solid lines) in Figure 5, it is clear that, relative to non-exporters, the wage premium for firms in the export market increases in magnitude towards the top end of the distribution. However, when controlling for more firm characteristics (estimating Equation 2), the distribution changes shape and the large wage inequality at the 75th and 95th percentiles drops (see dotted lines). Therefore, the observed wage inequality can mostly be explained by firm characteristics (i.e. firm size and worker productivity).
Table 4-A1 and Table 5-A1 and Figure 5 illustrate how the wage distribution differs between exporters with different dynamics (enter, exit or continue). The next point to consider is the destination or market that these exporters enter, continue in or exit. Figure 6 and Figure 7 add destination categories, showing the wage distribution of exporters that enter, continue in or exit from different destinations (using different controls).
 |
FIGURE 6: Distribution of the coefficients of the wage premium: exporter dynamics (to African and non-African countries). |
|
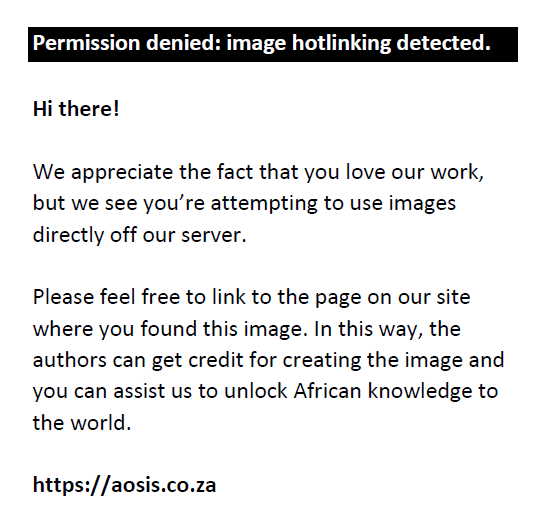 |
FIGURE 7: Wage inequality in terms of exporter status. |
|
Figure 6 combines the exporter dynamics with the destination served. Similar to Figure 4 and Figure 5, this figure is a visual representation of estimating Equation 1 and Equation 2 (for which the regression results are available on request). The difference between the equations is that Equation 1 only controls for one type of firm characteristic (namely capital per worker) whereas Equation 2 controls for two more firm characteristics (namely firm size and worker productivity). The results of Equation 1 are presented as the full bars (upper and lower end of each bar) and Equation 2’s results are presented as the lower (darker coloured) end of each bar.
Overall, from the results of Equation 1 and Equation 2, there is a clear export premium across the wage distribution. When distinguishing between exports to African and international markets, the premium is larger for exporters serving international markets. This is true across all export dynamic groups. Furthermore, the shape of the distribution of continuing and entering exporters is relatively similar.
The results of Equation 1 (represented by each full bar) show that there is an export premium across the wage distribution. Relative to non-exporters, higher wages are paid by exporters throughout the distribution. This premium increases in magnitude towards the top end of the distribution. Exporters, relative to non-exporters, pay much higher wages at the top end than at the bottom end.
In Figure 6, the darker coloured, lower end of each bar represents the premium when firm characteristics (number of employees and output per worker) are controlled for, while the lighter coloured, upper end is the additional premium without the controls. Overall, this indicates that the wage inequality is driven by the distribution of firm size (measured by the number of employees) and labour productivity (which is output per worker) within exporters compared to non-exporters (see Table 1), with much of the observed wage inequality being because larger, more productive firms are more likely to be exporters.
In addition to Figure 6, Figure 7 shows the standard deviation of each of these groups before (estimating Equation 1 – see bars on the left-hand side) and after (estimating Equation 2 – see bars on the right-hand side) controls for firm size and labour productivity are added. On the left-hand side, it is evident that there is a wide dispersion of wages within exporters (particularly within international exporters). However, almost all that dispersion (particularly within continuing exporters) is explained by labour productivity and the size of these firms (see bars on the right-hand side). This suggests that there is a large degree of dispersion of these variables (namely number of employees and output per worker) for these firm groups (represented by each bar) relative to non-exporters.
Even though the standard deviation (Figure 7) is substantially smaller after controlling for firm size and output per worker, there is still a difference in the within-firm wage distribution of exporters relative to non-exporters, as well as within exporters. From the literature it is clear that skills do have an influence on wage inequality. As there are no variables available on skills, an indirect way to take skills into account is by considering the GDP of the destination country and the quality of the product. Verhoogen (2008), Brambilla et al. (2012), Brambilla and Porto (2016), as well as Rankin and Schoër (2013) allude to the fact that the type of product (high quality versus low quality) exported or the type of destination (high or low income per capita) exported to, could influence workers’ wages. This is due to higher-quality goods being manufactured by more skilled workers who get paid a skills premium (Brambilla & Porto 2016; Rankin & Schoër 2013). Also, high-income countries have been shown to demand higher-quality goods, and firms exporting to these destinations subsequently pay higher wages (Brambilla & Porto 2016). These factors (type of destination country and type of product) could, therefore, influence the wage distribution and, therefore, wage inequality between exporters and non-exporters (as well as within exporters). The following section investigates these factors as possible sources of wage inequality.8
Possible sources of wage inequality
Estimation strategy: Possible sources of wage inequality
To be able to consider the impact of the type of export product and destination on within-firm wage distribution, the transaction-level customs data is used as it reports on the product being exported (HS6-digit), the export price and the country of destination. The panel data (dated from 2010 to 2014) used in this section were constructed using the customs transaction data as the base and merging firm characteristics (obtained from the CIT and IRP5 data sets) onto it. The starting point for this analysis is estimating the following regression of the general form:
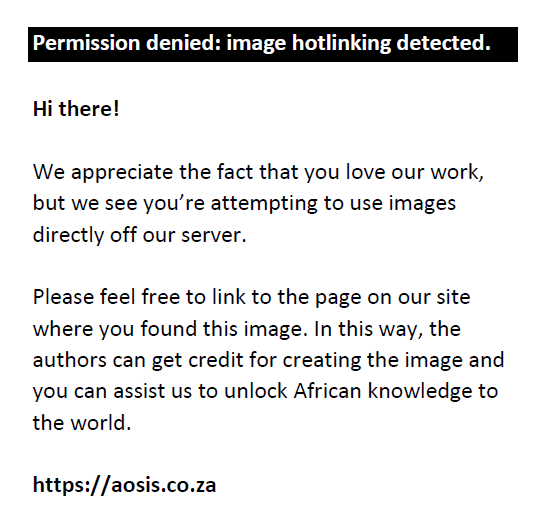
In Equation 3:
- Xijk,t – within-firm distribution of monthly wages (measured at the 5th, 25th, 50th, 75th and 95th percentiles)
- Exporterijk,t – dummy variable of export status (SACU, Africa, international, enter, continue)
- No.destijk,t – control dummy (number of destinations exported to by firm)
- No.prodijk,t – control dummy (number of products exported by firm)
- Industryijk,t – control dummy (four-digit ISIC classification) to account for heterogeneity
- firmjk,t – control for firm characteristics (natural logs of capital per worker, number of employees and output per worker)
- yearijk – control dummy for the years 2010–2014
- priceik,t – control for type of product (price is in real terms)
- GDPij,t – control for type of destination
- prod fe – product-fixed effects (at HS6-digit level)
- uijk,t – error term
The results of estimating Equation 3 are presented in the following section. Figure 6 shows that wage inequality is higher among international exporters than within African exporters and the same is true for entering versus continuing exporters. Figure 8 represents the distributions to African and non-African countries, Figure 9 represents exporter dynamics and Figure 10 represents a combination of destinations and dynamics.
 |
FIGURE 8: Distribution of the coefficients of the wage premium (inequality): firms exporting to African and non-African countries. |
|
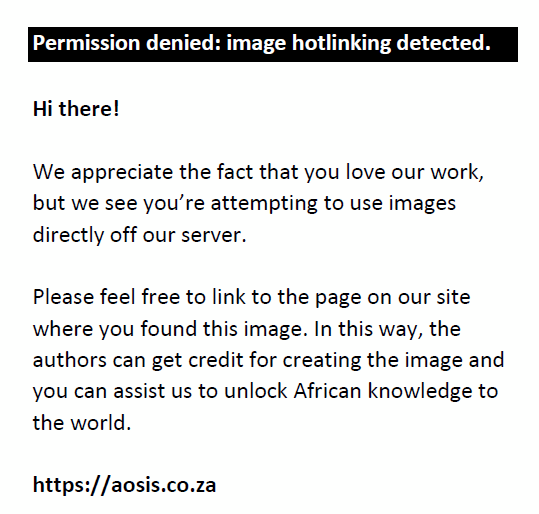 |
FIGURE 9: Distribution of the coefficients of the wage premium (inequality): exporter dynamics. |
|
 |
FIGURE 10: Distribution of the coefficients of the wage premium (inequality): exporter dynamics (to African and non-African countries). |
|
Results: Possible sources of wage inequality
Figure 8 provides an illustration of the distribution of the coefficients of the wage premium for African exporters relative to international exporters (the base). Similar to Figure 4, Figure 8 shows that international firms (the base) have higher wage premia across the distribution. Furthermore, each line in Figure 8 represents different combinations of Equation 3. Firstly, the Africa-only line illustrates the results of estimating Equation 3 without any type of product or destination controls. This provides a base (reference point) for the wage distribution and inequality.
When adding product price, the distribution shifts slightly down (shifts in the mean) but does not change shape. Therefore, the wage inequality does not seem to be due to product quality. By adding GDP per capita of the destination country, the distribution also shows a slight downward shift but does not flatten out (does not change shape). Thus, the destination country does not seem to influence the wage distribution. Finally, when adding product-fixed effects in combination with type of product and destination controls, the distribution’s shape stays similar. Thus, the wage inequality is not due to a specific product type or within-product type. For example, the fact that an exporter pays higher wages or has an unequal distribution of wages is not due to a specific product (e.g. car) being exported. Hence, the observed wage inequality has little to do with the destination country (high or low income per capita), the quality of the product (high-quality versus low-quality product) or the type of product being exported.
Not only is the wage inequality higher among international exporters, but Figure 6 shows that firms entering the export market have higher wage inequality than those continuing in the export market. The next section considers the wage inequality of exporters with different dynamics. It also investigates if the unequal distribution of wages changes shape when controls for product quality, GDP and fixed effects are added.
Figure 9 shows the wage distribution of entering firms relative to continuing firms.9 Similar to Figure 5, Figure 9 demonstrates that there is a wage premium for firms entering the export market. As in Figure 8, the distribution does not change shape when adding a control for product price, a GDP control or product-fixed effects. This confirms that, with entrants, wage inequality is not caused by the type of product or destination.
From Figure 8 and Figure 9 it is clear that the wage inequality is higher within international exporters (who form the base), than within African exporters, with the same being true for entering versus continuing exporters. The next section combines these two figures by considering the wage inequality of firms that enter and continue to export to African and non-African countries.
Figure 10 shows the wage distribution of firms entering the international market, entering the African market and continuing in the African market relative to firms continuing in the international market. This figure confirms two of the findings from Figure 6. Firstly that, relative to firms continuing in the international market (the base), continuing or entering firms in Africa do not have a wage premium, but firms entering the international market do. The within-firm wage distributions of entering (Africa only) and continuing (Africa only) exporters are very similar.
Secondly, Figure 10 adds to Figure 6 by considering if the type of product (high-quality versus low-quality product) exported or the type of destination (high or low income per capita) exported to could have an effect on the within-firm wage distribution. The results show that the within-firm wage distribution of firms entering the international export market (represented by the four top solid lines), relative to continuing international exporting firms (the base), do not change shape (flatten out) as product control, destination GDP control or product-fixed effects are added. Therefore, the wage inequality seems to have little to do with the destination country, the quality of the product or the type of product. It might suggest that it has something to do with the process of exporting or simply being in the export market (a firm’s export status). Another possible explanation is that the remaining wage inequality is due to a specific type of firm which has this (unequal) wage distribution even before it starts to participate in the export market. In such a case it is most likely that the firm is willing (and able) to pay a premium for a specific skill or skill set that is high in demand, but scarce. As explained by Bacchetta et al. (2017), if the supply of skills is inadequate for the demand, the price of skilled jobs increases and there is an increase in inequality.
Conclusions and policy recommendations
The aim of this article was to more fully understand the wage differences between non-exporters and exporters and within exporters (by considering exporter dynamics and destinations served). In short, it investigated within-firm wage distributions and inequality among South African manufacturing firms, with a focus on exporting firms. Twenty one per cent of all South African manufacturing firms export and these firms employ on average 19 workers, whereas firms that do not export employ on average 7 workers. Therefore, the impact of exporting firms’ wage inequality may have a significant impact on overall wage inequality in South Africa.
The results firstly showed that, right across the within-firm wage distribution (from the 5th to the 95th percentiles), there is a hierarchy in the wage premium for firms the further away a destination is located (i.e. SACU-only exporters’ wages are lower than Africa-only exporters’ wages, which are in turn lower than international exporters’ wages). Secondly, the results showed that the wage premium increases in magnitude towards the upper tail (75th and 95th percentiles) of the within-firm wage distribution (which illustrates wage inequality). Therefore, exporters (specifically international exporters) seem to pay higher wages (and thus provide better jobs), although there is a higher degree of wage inequality within these firms.
To arrive at potential explanations for this apparent wage inequality, the article considered the effect of firm characteristics (capital per worker, firm size and labour productivity). When firm size and labour productivity are controlled for, the wage distribution changes shape. This suggests that the wage inequality is largely driven by the size (number of employees) and labour productivity (output per worker) of exporters relative to non-exporters. Most of the observed wage inequality is because larger, more productive firms are more likely to be exporters. In other words, wage inquality may be accentuated by further trade liberalisation because it leads to a re-allocation of resources from less productive to more productive firms (as explained in Melitz 2003).
Even though the observed wage inequality is substantially smaller after controlling for firm size and output per worker, there is still a difference in the within-firm wage distribution of exporters relative to non-exporters, as well as within exporters. From the literature it emerged that there are two other possible sources of wage inequality – which relate to the skills levels required to manufacture goods – namely the characteristics of the destination economy (proxied by GDP per capita) and the quality of exports (proxied by price relative to the average price). When the characteristics of the destination economy and quality of exports are controlled for, the results show little evidence of wage inequality being driven by these. Therefore, the observed wage inequality has little to do with the destination country (high or low income per capita), the quality of the product (high-quality versus low-quality product) or the type of product being exported. Another possible explanation is that the remaining wage inequality is due to a specific type of firm (employing a specific type of person) that has this (unequal) wage distribution even before it enters the export market. In such a case it is most likely that the exporting firm is willing (and able) to pay a premium for a specific skill or skill set that is scarce but very valuable to the industry in question (e.g. highly effective marketers, technology experts or CEOs).
Clearly, policymakers cannot simply look to increased exports as a way of achieving more inclusive growth. Policies need to support specific types of exporting firms (the firms that have the potential to become the larger, more productive exporters). Specific interventions might include: providing financial support and market information (using tools such as the TRADE Decision Support Model) so that firms are better equipped to take advantage of available export opportunities, targeting investment in sectors with strong export growth potential, adapting trade and investment policy to allow cost-effective sourcing from abroad and to stimulate knowledge and technology transfer, and encouraging more competition in the local market.
However, at a more fundamental level, if the wage gap between exporting and non-exporting companies is to be narrowed (and indeed wage inequality within firms is to be addressed), much more serious attention needs to be paid at the policy level to improving education and skills development in the country. This would involve driving high-quality teaching and learning by building capacity and accountability in schools and other education and training institutions (OECD 2016), reducing government red tape in the education and training sectors to encourage greater participation by providers and quicker and better results, and providing greater (including financial) incentive to firms to engage in staff training and development. Another policy imperative should be to open up the education and training sector to more foreign participation, and promoting the regional exchange of expertise and cross-recognition of qualifications to encourage a more mobile workforce in southern Africa. Furthermore, accentuated within-firm wage inequality (as a result of trade liberalisation) might be addressed by ensuring that low-skilled individuals participate in life-long learning opportunities, thereby creating a more skilled cohort of workers and prompting a more inclusive approach to productivity enhancement.
Ultimately, through these various measures, the supply of skilled workers would increase, thereby reducing the premium paid to scarce skilled workers and spreading the workload and commercial benefits more evenly throughout society.
In conclusion, there is much scope for further research to be done in the field covered by the study, which will further enhance people’s understanding of the labour dynamics of exporting firms in South Africa. This in turn will help to give greater impetus to South Africa’s currently troubled export and job creation efforts.
Acknowledgements
All the authors would like to thank the United Nations University World Institute for Development Economics Research and National Treasury for funding support. Also, they would like to thank the South African Revenue Service for the data.
Competing interests
The authors have declared that no competing interest exists.
Authors’ contributions
This article is based on a chapter C.B.’s PhD thesis and C.B. constructed the article. M.M. and N.R. were her supervisors, and assisted with the conceptual construction of the study (and article).
Ethical consideration
North-West University Research Committee meeting (TRADE), ethical clearance number EMS/15/09/15-04-01, 27 February 2017.
Funding information
This works received support from United Nations University World Institute for Development Economics Research and National Treasury. M.M. also acknowledges support from the National Research Foundation (NRF) of South Africa (grant no. 127652).
Data availability statement
The data is held by the National Treasury.
Disclaimer
The views and opinions expressed in this article are those of the authors and do not necessarily reflect the official policy or position of any affiliated agency of the authors. Any opinion, finding and conclusion or recommendation expressed in this material is that of the authors and the NRF does not accept any liability in this regard.
References
Bacchetta, M., Gregg, C., Rubínová, S. & Klok, B.T., 2017, Investing in skills for inclusive trade, World Trade Organization (WTO) and International Labour Office (ILO), Geneva, viewed 29 August 2017, from https://www.wto.org/english/res_e/booksp_e/investinsskills_e.pdf.
Bas, M., 2012, ‘Technology adoption, export status, and skill upgrading: Theory and evidence’, Review of International Economics 20(2), 315–331.
Bernard, A.B. & Jensen, J.B., 1997, ‘Exporters, skill upgrading, and the wage gap’, Journal of International Economics 42(1), 3–31.
Bernard, A.B., Jensen, J.B. & Schott, P.K., 2009, ‘Importers, exporters and multinationals: A portrait of firms in the US that trade goods’, in T. Dunne, J.B. Jensen & M.J. Roberts (eds.), Producer dynamics: New evidence from micro data, pp. 513–552, University of Chicago Press, Cambridge, MA.
Bernini, M., Guillou, S. & Treibich, T., 2015, Export behavior and labor characteristics, viewed 15 November 2016, from https://www.unige.ch/degit/files/1014/3937/9937/Treibich.pdf.
Bhorat, H., Oosthuizen, M., Lilenstein, K. & Steenkamp, F., 2017, Firm-level determinants of earnings in the formal sector of the South African labour market (No. 2017/25), WIDER Working Paper, United Nations University, Helsinki.
Brambilla, I., Chauvin, N.D. & Porto, G., 2015, Wage and employment gains from exports: Evidence from developing countries, CEPII Working Paper no. 2015, 28 December, viewed 19 January 2017, from http://www.cepii.fr/PDF_PUB/wp/2015/wp2015-28.pdf.
Brambilla, I., Lederman, D. & Porto, G., 2012, ‘Exports, export destinations, and skills’, The American Economic Review 102(7), 3406–3438.
Brambilla, I. & Porto, G.G., 2016, ‘High-income export destinations, quality and wages’, Journal of International Economics 98, 21–35.
Edwards, L., 2001, ‘Globalisation and the skills bias of occupational employment in South Africa’, South African Journal of Economics 69(1), 40–71.
Edwards, L., Sanfilippo, M. & Sundaram, A., 2016, Importing and firm performance: New evidence from South Africa (No. 2016/39), WIDER Working Paper, United Nations University, Helsinki.
Fu, D. & Wu, Y., 2013, ‘Export wage premium in China’s manufacturing sector: A firm level analysis’, China Economic Review 26, 182–196.
Klein, M.W., Moser, C. & Urban, D.M., 2013, ‘Exporting, skills and wage inequality’, Labour Economics 25, 76–85.
Krugman, P.R., 2008, ‘Trade and wages, reconsidered’, Brookings Papers on Economic Activity 2008(1), 103–154.
Matthee, M., Rankin, N. & Bezuidenhout, C., 2017, Labour demand and the distribution of wages in South African manufacturing exporters (No. 2017/11), WIDER Working Paper, United Nations University, Helsinki.
Matthee, M., Rankin, N., Naughtin, T. & Bezuidenhout, C., 2016, The South African manufacturing exporter story (No. 2016/38), WIDER Working Paper, United Nations University, Helsinki.
Melitz, M., 2003, ‘The impact of trade on intra-industry reallocations and aggregate industry productivity’, Econometrica 71(6), 1695–1725.
Melitz, M. & Redding, S.J., 2012, Heterogeneous firms and trade (No. w18652), National Bureau of Economic Research, Cambridge, MA.
Naughtin, T. & Rankin, N.A., 2014, South African super-exporters: Are they different and what does this mean for policy?, viewed 14 February 2017, from http://www.tips.org.za/research-archive/manufacturing-conference-2014/item/2798-south-african-super-exporters-are-they-different-and-what-does-this-mean-for-policy
OECD, 2016, The productivity-inclusiveness nexus, viewed 05 September 2017, from https://www.oecd.org/global-forum-productivity/library/The-Productivity-Inclusiveness-Nexus-Preliminary.pdf.
Rankin, N., 2001, ‘The export behaviour of South African manufacturing firms’, Munich Personal RePEc Archive paper No 16904, viewed 14 February 2017, from https://mpra.ub.uni-muenchen.de/16904/1/MPRA_paper_16904.pdf.
Rankin, N., 2013, Exporting and export dynamics among South African firms, Occasional Paper No 149, June 2013, South African Institute of International Affairs, Johannesburg.
Rankin, N. & Schöer, V., 2013, ‘Export destination, product quality and wages in a middle-income country, the case of South Africa’, Review of Development Economics 17(1), 64–73.
Stolper, W.F. & Samuelson, P.A., 1941, ‘Protection and real wages’, The Review of Economic Studies 9(1), 58–73.
Trademap, 2020, Trade statistics for international business development, viewed 10 February 2020, from https://www.trademap.org.
UNU-WIDER (United Nations University, World Institute for Development Economics Research), 2017, Regional growth and development in Southern Africa, viewed 20 February 2017, from https://www.wider.unu.edu/project/regional-growth-and-development-southern-africa.
Van Der Berg, S., 2011, ‘Current poverty and income distribution in the context of South African history’, Economic History of Developing Regions 26(1), 120–140.
Verhoogen, E.A., 2008, ‘Trade, quality upgrading and wage inequality in the Mexican manufacturing sector’, Quarterly Journal of Economics 123(2), 489–530. https://doi.org/10.1162/qjec.2008.123.2.489
World Bank, 2016, World development indicators, viewed 10 March 2016, from http://databank.worldbank.org/data/reports.aspx?source=2&series=NY.GDP.PCAP.PP.CD&country
World Bank, 2017, World Bank county overview, viewed 23 March 2017, from http://www.worldbank.org/en/country/southafrica/overview.
Footnotes
1. A part of this article was incorporated into a UNU-WIDER working paper (2017/11) titled ‘Labour demand and the distribution of wages in South African manufacturing exporters’ (Matthee, Rankin & Bezuidenhout 2017).
2. SACU is a customs union between Botswana, Lesotho, Namibia, Swaziland and South Africa.
3. Entering firms are defined as firms that did not export in year t–1, but exported in year t.
4. Exiting firms are firms that exported in year t–1, but not in year t.
5. Continuing firms are firms that exported in both year t–1 and year t.
6. The GDP per capita was obtained from the World Bank (2016).
7. The wage distribution is the average figures (in wage per person) per quantile for descriptive purposes.
8. A recommendation for further research is to better measure the sources of inequality by using the Theil index. The Theil index allows one to determine the sources of inequality (domestic versus external factors) as the index can be decomposed into inequality within and between different defined subgroups.
9. There are no exiting firms because they do not trade and therefore would not be included in the customs transaction data.
|