Abstract
Background: Despite considerable efforts by the South African government, such as the Youth Employment Service (YES) programme, unemployment remains an enigma.
Aim: The study seeks to explore the responsiveness of sectoral employment to changes in the sectoral output in South Africa.
Setting: We focus on the agriculture and informal sectors, with the understanding that a large portion (93%) of South Africa’s unemployed population does not have tertiary education qualifications. Thus, the current South Africa’s unemployment phenomenon appears to necessitate the development of policies that will create inclusive skill-based jobs. The study’s hypothesis is theoretically underpinned by Okun’s law, according to which output growth is considered as a primary labour demand stimulus in the economy. When the labour demand function is co-integrated, Okun’s law is assumed to hold; otherwise, ‘jobless growth’ applies.
Method: The findings from the Engle-Granger two-step testing procedure on the double-log linear labour demand function over the 1993–2018 period show evidence of jobless growth in the formal agricultural sector, while the informal agriculture and informal non-agriculture sectors demonstrated features of Okun’s law.
Results: Notably, the authors found a fairly elastic (1.35%) employment intensity in output growth in the non-agriculture informal sector, with an equilibrium adjustment rate of 86% within a year, ceteris paribus.
Conclusion: The findings suggest that, while South Africa’s formal agriculture is no longer labour-intensive, due to agricultural mechanisation, economic policy consciousness in the informal sector, including agri-entrepreneurship, is necessary to create inclusive mass employment in South Africa.
Contribution: This study delves into the informal sector, which has been frequently overlooked as a potential solution to South Africa’s unemployment crisis.
Keywords: agriculture; Okun’s law; employment; jobless growth; informal sector; South Africa.
Introduction
The consistent rise in South Africa’s unemployment rate since 2008 (see Figure 1) is cause for concern, as the scenario appears to be deviating from the National Development Plan (NDP)’s goal of reducing unemployment to at most 6% by 2030. The seasonally adjusted unemployment rate in the third quarter of 2021 (34.69%) is almost six times higher than the 2030 targeted maximum threshold. Based on Okun’s law, one can assume that South Africa’s economy is underperforming. However, the country’s output, as measured by the real Gross Domestic Product (GDP), has been steadily positive, save for the COVID-19 structural break in 2020, from which the economy is gradually recovering (see Figure 1). Okun (1962) proposed the inverse output – unemployment nexus and, as a result, concluded that economic growth is a prerequisite for job creation. This proposition has been the basis for most job-creating economic growth empirics in developing countries.
 |
FIGURE 1: South Africa’s unemployment – Gross Domestic Product relationship for the period Q1 2008 to Q3 2021. |
|
In South Africa Okun’s proposition ceased to hold in the early 1990s due to structural changes (Mkhize 2019). This has been the case for the majority of African countries, regardless of the distortion period (see, for example, Ajilore & Yinusa 2011; Dada 2018; Léautier & Hanson 2013). Thus, for the past decades, the growth pattern in the majority of African countries has been described as ‘jobless growth’ (Léautier & Hanson 2013), and it seems that South Africa is no exception (Figure 1). Jobless growth tends to be a threat to inclusive growth, resulting in poverty, inequality and social unrest (Dada 2018). South Africa is in a state of disarray as a result of these challenges, which necessitate immediate policy intervention. According to the World Bank Group’s April 2020 poverty and equity statistics, approximately 55.5% of South Africa’s population lives below the national upper-bound poverty line, while the income distribution, as measured by the Gini index, stood at 63.3%. Following the severe civil unrest that the country experienced in July 2021, these statistics are likely to have worsened.
For the past three decades, the South African economy has undergone several fundamental structural reforms to boost economic growth and address the country’s ongoing tripeptide of challenges (unemployment, poverty and inequality). Educational reforms to promote human capital development through education, industrialisation, and various socioeconomic transformation initiatives are among these reforms. This period has, therefore, seen an influx of secondary and tertiary graduates into the job market, yet various industries were experiencing structural shifts from labour-intensive to capital-intensive. Such structural changes often result in a surplus in the labour force due to the accumulation of new entrants and job losses (Altman 2006). In line with Altman (2006), South Africa’s Quarterly Labour Force Survey (QLFS) statistics show that job losers and new entrants increased by approximately 50.4% and 38.4%, respectively, from the first quarter of 2008 to the first quarter of 2019. While industrialisation and human capital development through education are commendable efforts, Léautier and Hanson (2013) caution African countries not to neglect the agriculture sector. Africa remains an ‘agriculture-based society and, will need to look to the agriculture sector to generate the bulk of needed employment in the coming years’ Léautier and Hanson (2013:1). Similarly, Dada (2018) asserts that agriculture-led economic growth is inclusive, and thus has a high potential for creating mass employment in Africa.
The period has also seen a rise in employment in the informal sector. According to QLFS data, informal employment increased from 15.3% in 2013 to 18.3% in 2019, while formal employment decreased from 71.2% to 68.5% over the same period. It is assumed that the unemployed youth population living below the poverty line turned to the informal sector for employment. This is consistent with Blaauw’s (2017) assertion that in a predominantly poor society, informality is the primary means of earning income and overcoming poverty. As of 2018, one in every six employed South African citizens worked in the informal sector, which contributed approximately 18% of the country’s GDP (Fourie 2018). This brief on the informal sector exhibits the sector’s potential to support the NDP’s 2030 agenda of creating 90% of jobs by small, medium- and micro-enterprises (SMMEs). However, the sector has remained on the periphery of economic analysis and policy consciousness.
In light of the preceding discussion, it is reasonable to argue that South Africa’s dilemma of jobless growth is likely to persist as long as the agriculture and informal sectors are overlooked. While this argument does not dispute Mkhize’s (2019) recommendation to create new employment opportunities through investing in non-agriculture sectors, typically the tertiary sector, the recommendation lacks inclusiveness, which is a major shortfall in South Africa’s employment crisis. The current unemployment phenomenon in South Africa appears to necessitate the development of policies that will create inclusive skill-based jobs (see Figure 2). Figure 2 illustrates that between the first quarters of 2008 and 2019, the population of unemployed tertiary education graduates was very low (7%), compared to those who did not attain tertiary education (93%). According to these statistics, the unskilled labour force accounts for the majority of South Africa’s unemployed population. This study, therefore, builds from Mkhize’s (2019) empirical work to provide an alternative empirical analysis of the sectoral employment intensity of output growth in South Africa. The study focuses on agriculture and informal sectors, with the understanding that a large portion (93%) of South Africa’s unemployed population are relatively unskilled. In other words, the study seeks to explore the responsiveness of sectoral employment to changes in the sectoral output of South Africa’s agriculture and informal sectors to inform policymakers about the extent to which these sectors can absorb both skilled and unskilled labour.
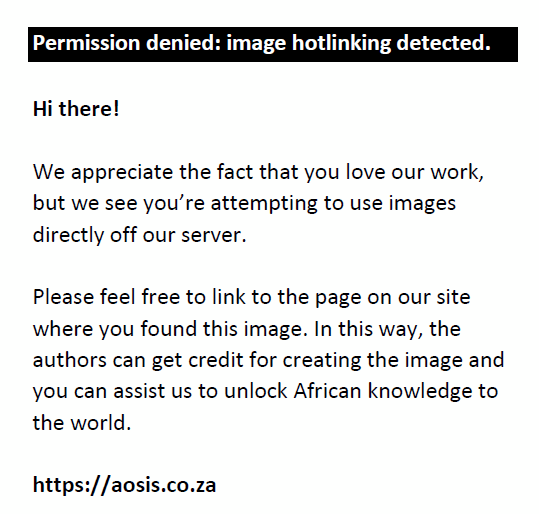 |
FIGURE 2: Average proportion of South Africa’s unemployed population according to the highest level of education for the period Q1 2008 to Q1 2019. |
|
The study is organised into five sections. Following this introductory section, a review of the literature on the relationship between labour demand and output is conducted. The methodology is discussed and specified in the third section. In section four the findings are presented and discussed, while the study is concluded in the final section.
Employment-output Nexus
Employment and output relationships can vary, depending on a variety of factors, including output per worker, time frames, labour and goods market adjustments, and sectoral economic activities (see, for example, Landmann 2004; Sahin, Tansel & Berument 2013). In the short run, output growth may not lead to an increased labour demand. Rather, it may only result in increased working hours because firms may avoid the costs of recruiting and training new workers by paying overtime to the existing workers, holding other things constant. However, constant output growth may overwhelm the existing workforce, forcing firms to increase employment capacity in the long run.
Using Australian workers, Okun (1962) proposed that a country’s output growth must be approximately 3% above the nominal rate in a year to achieve a 1% point increase in employment. In several studies, however, it is argued that, while Okun’s coefficient is a useful benchmark in the formulation of an employment-output macroeconomic policy, it varies with the economy and time frame under consideration, among other factors, that influence the employment intensity of output growth (see, for example, Karim & Aomar 2016; Okun 1970). On the other hand, while industrialisation and other structural reforms may lead to increased productivity and thus output growth both in the short and long run, they also force firms to downsize their labour force, weakening or even eliminating the positive employment-output relationship. For instance, Upender (2006) found that the employment-output relationship in the Indian agricultural sector switched from positive during the pre-reform period (1983–1991) to negative in the post-reform period (1992–2000). Thus, the fourth industrial revolution may result in jobless growth as it promotes the use of machinery in place of the considerable quantity of human labour, decreasing the demand for labour, however, with a positive impact on output.
Furthermore, as Sahin et al. (2013) point out, various movements occur in the labour and goods markets as a result of the market’s specific macroeconomic factors and institutional settings. In general, disequilibrium between the two markets tends to be a result of labour market rigidities. Firstly, the effects of the output shocks phase out faster in the short run than those of labour market shocks. Secondly, closing the long-run employment gap may take longer than closing the long-run output gap. Thirdly, changes in the labour market are smaller than changes in the goods market, and changes in output are accompanied by smaller changes in employment. As a result, the economy’s employment creation puzzle may be structural, and the supply side’s ability to generate employment may be weaker in the short run. However, as the interaction between the forces of the goods and labour markets develops, they tend to reach equilibrium in the long run.
The relationship between employment and output can also be explained in terms of the economy’s sectoral economic activities (see, for example, Mkhize 2019; Ajilore & Yinusa 2011). The heterogeneity in the sectoral employment intensity of output growth, according to Sahin et al. (2013), is due to differences in cyclical behaviour, wages, technological intensity, capital–labour substitution effects, and employment multipliers. This implies that looking through a sectoral lens is vital when considering the issue of employment intensity of output growth. However, empirical evidence on the subject is still lacking, particularly in sub-Saharan Africa, where unemployment is rampant. In Botswana, Ajilore & Yinusa (2011) found that labour demand in both the agriculture and non-agriculture sectors was inelastic (less than 1%) in response to national output growth from 1990 to 2008. Botswana’s national output predominantly depends on the mining sector (Leshoro 2014). The mining sector is capital- and technology-intensive; thus, jobless growth in the country is not a surprising outcome. In South Africa, Mkhize (2019) found disparities in employment elasticities across eight non-agriculture sectors from 2000 to 2012. His findings show that only the finance and business services sector had an elastic employment intensity of output growth (1.56%), while the rest were inelastic, confirming jobless growth features.
The empirical works of Mkhize (2019) and Ajilore & Yinusa (2011) lack explicit analyses of employment intensity of output growth in the informal sector, yet according to the International Labour Organisation (ILO)’s press release on 30 April 2018, 85.8% of employment in Africa is informal, with informal agriculture accounting for the largest proportion. The press release also emphasised the significance of education levels in informality in developing economies:
People who have completed secondary and tertiary education are less likely to be in informal employment compared to workers who have either no education or completed primary education (ILO 2018, 30 April 2018).
Considering the educational attainment of the current unemployed population in South Africa (Figure 2), the informal sector may be a significant source of employment. Figure 2 shows that 60% of the unemployed population have not attained secondary education. Furthermore, due to the country’s educational reforms, there has been an influx of tertiary education graduates in the labour market, resulting in a decrease in the demand for secondary and matric education graduates, which account for 33% of South Africa’s unemployed population. This implies that 93% of the unemployed population in South Africa can hardly be absorbed in the formal sector (Figure 2). In several studies it is suggested that when there is an excess of labour in the market, informality may serve as a cheap alternative to formal employment (see, for example, Adair & Souag 2018; Blaauw 2017; Pasipanodya 2020). Agriculture, on the other hand, is perceived as a labour-intensive and inclusive source of mass employment, typically in Africa (see, for example, Dada 2018; Léautier & Hanson 2013). Thus, the current study builds on Mkhize’s (2019) empirical work to provide an alternative empirical analysis of the sectoral employment intensity of output growth in South Africa, with a particular emphasis on agriculture and informal sectors. The analysis is carried out from the theoretical standpoint of Okun’s law.
Empirical strategy
Following Mkhize (2019), Ajilore & Yinusa (2011), and Upender (2006), this study utilises the double-log linear labour demand function (Equation 2) derived from the constant elasticity of substitution (CES) production function (Equation 1) through solving the marginal product of labour:
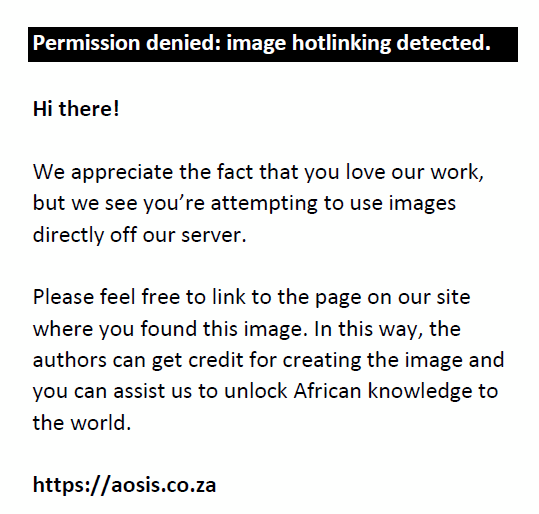
Where:
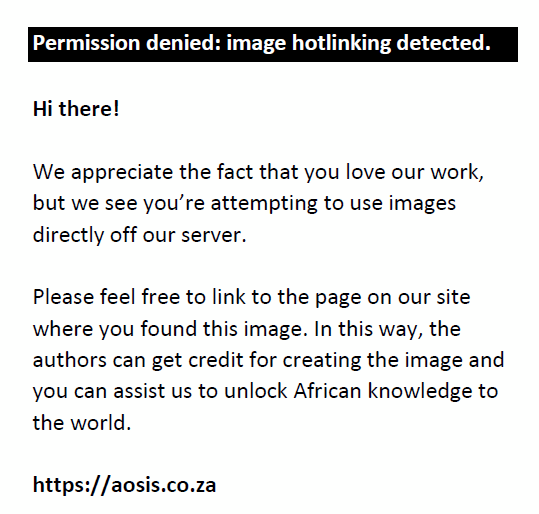
As in the Cobb-Douglas production function, y is the output, K is the capital input, L is the labour input, and A is the productivity parameter. The subscript γ represents the extent of substitution between capital and labour inputs and is related to substitution elasticity δ = 1/(1 + γ) (Romeo 2020). The subscripts ∂ and Ø proxy for the returns to scale and distribution parameters, respectively. Equation 1 can be decomposed to the double-log linear employment function specified:

For the purpose of this study, y is the sectorial gross value added (GVA) at factor cost in real terms, L is the employed population in a specific sector, X is a set of regressors that are justified in the literature as vital in explaining labour demand, ε is the disturbance term, and t represents time. These regressors include sector-specific nominal wages (seasonally adjusted), the user cost of capital, and the inflation rate. The estimated coefficients of Equation 2 are interpreted as elasticities because the model is specified in logs.
Estimation technique
The study adopted the Engle-Granger two-step testing procedure (Engle & Granger 1987) to test the null hypothesis of jobless growth assuming evidence that it exists if there is no co-integration between employment (L) and output (y). Engle-Granger’s two-step testing procedure proceeds in two linked steps. The first generates the residuals from the static co-integration equation. The generated Ordinary Least Squares (OLS) residuals are a measure of disequilibrium. A co-integration test is thus a test of whether the OLS residuals have a unit root:
H0: The residuals have a unit root.
Accepting H0 implies that there is no co-integration; thus, confirming jobless growth in that particular sector; otherwise, Okun’s law applies. If co-integration holds, the static co-integration equation is said to be super-consistent, implying that the standard OLS diagnostic test may not be required because the only thing that matters is residual stationarity. A valid error correction term (ECT[−1]) exists when a set of variables is co-integrated. Accordingly, the second step is to estimate the error correction model by fitting a regression of first-differenced residuals on lagged residuals using the generated OLS residuals from the first step. To indicate the presence of a long-run causal relationship, the ECT(−1) must be significantly negative.
In terms of data, the study utilised readily available sectoral time-series annual data from 1993 to 2018. The study period (1993–2018) is constrained by the availability of data. Table 1 describes variables and data sources.
TABLE 1: Variables description and data sources. |
The GVA of the non-agriculture informal sector was estimated by multiplying the total GVA from Quantec by the Dynamic General Equilibrium model-based (DGE) percentage estimates of informal sector output contribution, sourced from the World Bank database. The World Bank’s informal economy database provides the informal sector’s output contribution estimates based on the Multiple Indicators Multiple Causes (MIMIC) model and the GGE model. Although the difference in estimates is marginal, we chose DGE model-based estimates because they are ‘based on more volatile variables such as employment, investment and productivity’ (Elgin et al. 2021:11). Multiple Indicators Multiple Causes estimates are based on slow-moving variables, such as institutional quality. Since GVA data matching formal and informal agriculture employment is not readily available, we analysed both sectors using total agricultural (both formal and informal) GVA.
Estimated results and discussion
The findings are presented in the Engle-Granger two-step testing procedure, but we begin with descriptive statistics (Figure 3).
 |
FIGURE 3: Employment and gross value added in the South Africa’s Agriculture and Informal sectors. |
|
Figure 3 demonstrates that employment in the agriculture sector is primarily dependent on formal agriculture. Moreover, labour demand in the sector has decreased by 33% from 1 447 429 workers in 2006 to 969 172 workers in 2018. One would expect the agricultural sector’s output to have decreased in response to a decrease in labour demand. However, agriculture output has remained constant at an annual average value of R68.3 billion from 1993 to 2018. Based on agricultural statistics, it is reasonable to attribute job losses in the formal agriculture sector to agricultural mechanisation; as a result, the validity of jobless growth in this sector is most likely. The descriptive statistics also show that the country’s total output has steadily increased from R440.2bn in 1993 to R4.7 trillion in 2018. Thus, agriculture accounted for a very small share of the country’s output, 3.3% per year on average over the study period. Between 1993 and 2018, the informal agriculture sector employed an average of 270 863 people per year. Although the agriculture sector is regarded as the largest employer of informal labour in developing countries (ILO 2018), Figure 3 shows that, relative to the non-agriculture informal sector, small-scale farming in South Africa has been neglected since the early 1990s. Non-agriculture informal sector labour demand surged by 128% from 1 891 139 workers in 2003 to 4 310 406 workers in 2008 and has remained above 4 million since then. However, Rogan and Skinner (2017) and Blaauw (2017:345) indicate that the sector’s employment is still small relative to peer-group countries, yet ‘distinctly long term in nature’. The output of the non-agriculture informal sector has also steadily increased, from R114.7bn in 1993 to R1.1 trillion in 2018. The descriptive statistics of the non-agriculture informal sector appear to reflect an aspect of Okun’s law.
Co-integration tests results
As a preliminary check of the co-integration test, we conducted unit root tests of all variables using the Co-integration Augmented Dickey-Fuller (CADF) test as recommended by Engle and Granger (1987). Except for the cost of capital and inflation variables, we found that other variables have unit roots in levels and are generally differenced stationary of orders one I(1) and two I(2). Following this preliminary examination, the Engle-Granger co-integration tests for each targeted sector were performed, and the results are shown in Table 2.
TABLE 2: Co-integration ADF test results. |
Table 2 shows that the null hypothesis of no co-integration could not be rejected on total agriculture and formal agriculture models, confirming jobless growth. Similar findings were documented in Turkey by Sahin et al. (2013). They found that the Turkish agriculture sector did not demonstrate an employment-output link both in the short run and long run during the period (1988–2008), reflecting characteristics of jobless growth. This finding, as highlighted in descriptive statistics, confirms that South Africa’s agriculture sector predominantly depends on commercial agriculture. Commercial agriculture has become capital-intensive as a result of agricultural mechanisation; therefore, the evidence of jobless growth in the sector is not surprising. On the other hand, we rejected the null hypothesis in both the informal agriculture and informal non-agriculture models, demonstrating Okun’s law features in the sectors. This implies that there was a long-run relationship between the informal sector’s employment and output for the period (1993–2018).
Employment intensity of output growth results
The findings on the employment intensity of output growth in South Africa’s agriculture and informal sectors are shown in Table 3 below. Following the results in Table 2, we estimated Equation 2 in error correction form for both the informal agriculture and informal non-agriculture models to determine the adjusting speed to equilibrium, while the total agriculture and formal agriculture models were estimated within a short-run perspective. The ECT(−1) denotes the rate at which labour demand returns to equilibrium, following a change in output and other explanatory variables in the model. The coefficients employment elasticity of output growth, pointing to the causal relationship between employment and output.
TABLE 3: Labour demand elasticities in South Africa’s agriculture and informal sectors. |
Table 3 shows that the employment-output relationship is statistically insignificant in both total agriculture and formal agriculture models, even in the short run. Regarding the co-integrated models, we found negative and highly significant ECT(-1) values in both informal agriculture (−0.64) and informal non-agriculture (−0.86) models, implying a disequilibrium adjusting rate of 64% and 86% within a year, respectively. However, the informal agriculture sector’s employment intensity of output growth is statistically insignificant. This is not surprising considering that informal agriculture in South Africa has been neglected since the 1990s (see Figure 3).1 The findings of the non-agriculture informal sector model show a fairly elastic (1.35%) employment intensity of output growth over the study period, with a statistical significance at 1%, ceteris paribus. If we review our findings together with those of Mkhize (2019), we derive that the non-agriculture informal sector is the South Africa’s second most labour-intensive sector after the finance and business services sector (1.56%).
All control variables entered the respective models as expected. The estimated coefficient of cost of capital in the informal agriculture sector model is highly significant and negative, implying that the high cost of capital discourages potential agri-entrepreneurs and the growth of existing ones. Anecdotal evidence suggests that South Africa’s agri-entrepreneurs (both start-ups and existing) face capital constraints to finance raw materials, input, and operational costs. Although some raw materials can be substituted with labour, such as labour instead of tractors, productivity will be low. Conversely, the same variable is significantly positive in the non-agriculture informal sector model. This implies that non-agriculture SMMEs can substitute capital with labour when capital costs are high. This is especially true in South Africa, where the service industry, particularly trade, dominates the informal sector (Rogan & Skinner 2017). Thus, an increase in the cost of capital reduces capital demand while increasing labour demand. The estimated non-agriculture informal sector’s wage coefficient is positive and highly significant, indicating that entrepreneurs are drawn to high-paying informal economic activities, and their wages according to Zhou and Pindiriri (2015), depend on output. Inflation raises operating costs, particularly for SMMEs, and thus discourages entrepreneurship.
The long-run regressions in Table 3 are subject to residual tests performed to confirm the fundamental assumptions of the classical long-run OLS model. These tests include normality, heteroscedasticity and serial correlation tests, and the results are shown in the subsequent table.
As shown in Table 4, no null hypothesis could be rejected for any of the tests, indicating that the long-run estimates in Table 3 are robust and correctly specified.
Conclusion
Given the ILO’s assertion about the inverse relationship between education level and informality, discerning the employment intensity of output growth in the informal sector is vital, especially in developing countries where education attainment levels are relatively low. For South Africa in particular, QLFS statistics show that from 2008 to 2019, an average of 93% of the unemployed population did not have tertiary education qualifications, implying that the informal sector can be a significant source of employment. However, the sector has remained on the periphery of economic analysis and policy consciousness. Furthermore, literature perceives agriculture as an inclusive labour-intensive sector, and thus a source of mass employment in developing economies. Thus, this study examined the responsiveness of sectoral employment to changes in the sectoral output of South Africa’s agriculture and informal sectors for the period (1993–2018), using the Engle-Granger two-step testing procedure on the double-log linear labour demand function. We found that employment and output are only co-integrated in the informal sector (both agriculture and non-agriculture), confirming Okun’s law features. However, the employment elasticity of the informal agriculture sector’s output growth was statistically insignificant over the study period. This finding supports our statistics, which show that small-scale farming is neglected in South Africa, despite its potential to generate self-employment. The findings of the non-agriculture informal sector exhibited a highly significant and fairly elastic (1.35%) employment intensity of output growth over the study period, with an equilibrium adjustment rate of 86% within a year, ceteris paribus. This finding leads to the conclusion that the non-agriculture informal sector is South Africa’s second most labour-intensive sector, after the finance and business services sector. In the formal agriculture sector, the employment-output relationship appears to have been eliminated by agriculture mechanisation which promoted jobless growth in the sector. These findings suggest that South Africa’s formal agriculture is no longer labour-intensive as a result of agricultural mechanisation. However, in line with sustainable development goal (SDG) 8.3, economic policy consciousness about the informal sector, including agri-entrepreneurship, is necessary to create inclusive mass employment, which is an essential missing piece in the puzzle to curb unemployment in South Africa. Given the availability of data for a significantly longer period (prior 1993 or alternatively quarterly data), future studies can employ robust time-varying techniques for the same study.
Acknowledgements
The authors gratefully acknowledge the contributions they received when they presented this study at the University of Zululand’s Mbali Conference 2022 and the Free State province Treasury Department’s 5th Annual Free State Provincial Research Colloquium.
Competing interests
The authors declare that they have no financial or personal relationships that may have inappropriately influenced them in writing this article.
Authors’ contributions
M.N. was the primary researcher as part of his post-doc fellowship program. H.N. supervised the research.
Ethical considerations
This article followed all ethical standards for research without direct contact with human or animal subjects.
Funding information
This research received no specific grant from any funding agency in public, commercial, or not-for-profit sectors.
Data availability
The data that support the findings of this study are available from the corresponding author, M.N., upon reasonable request.
Disclaimer
The views and opinions expressed in this article are those of the authors and do not necessarily reflect the official policy or position of any affiliated agency of the authors.
References
Adair, P. & Souag, A., 2018, ‘Okun’s law, unemployment and informal employment: The impact of labour market policies in Algeria since 1997’, Les Cahiers du Cread 33(123), 129–159.
Ajilore, T. & Yinusa, O., 2011, ‘An analysis of employment intensity of sectoral output growth in Botswana’, Southern African Business Review 15(2), 26–42.
Altman, M., 2006, ‘Identifying employment-creating sectors in South Africa: The role of services industries’, Development Southern Africa 23(5), 627–647. https://doi.org/10.1080/03768350601021871
Blaauw, P.F., 2017, ‘Informal employment in South Africa: Still missing pieces in the vulnerability puzzle’, Southern African Business Review 21, 339–369.
Dada, E.A., 2018, ‘Jobless growth in Nigeria: Determining employment intensive sectors’, Journal of African Development 20(2), 69–78. https://doi.org/10.5325/jafrideve.20.2.0069
Elgin, C., Kose, M.A., Ohnsorge, F. & Yu, S., 2021, Understanding informality, C.E.P.R. Discussion Paper 16497, Centre for Economic Policy Research, London.
Engle, R.F. & Granger, C.W.J., 1987, ‘Co-Integration and error correction: Representation, estimation, and testing’, Econometrica 55(2), 251–276. https://doi.org/10.2307/1913236
Fourie, F., 2018, The South African informal sector: Creating jobs, reducing poverty, HSRC Press, Cape Town.
FRED Economic Data, 2022a, Consumer price index: All items for South Africa, viewed 01 March 2022, from https://fred.stlouisfed.org/series/ZAFCPIALLAINMEI.
FRED Economic Data, 2022b, Long-term government bond yields: 10-year: Main (including benchmark) for South Africa, viewed 01 March 2022, from https://fred.stlouisfed.org/series/IRLTLT01ZAA156N.
FRED Economic Data, 2022c, Real gross domestic product for South Africa (seasonally adjusted), viewed 01 March 2022, from https://fred.stlouisfed.org/series/NGDPRSAXDCZAQ.
FRED Economic Data, 2022d, Unemployment rate (seasonally adjusted): Aged 15–64: All persons for South Africa, viewed 01 March 2022, from https://fred.stlouisfed.org/series/LRUN64TTZAQ156S.
International Labour Organization (ILO), 2018, Informal economy: More than 60 per cent of the world’s employed population are in the informal economy, viewed 28 April 2022, from https://www.ilo.org/global/about-the-ilo/newsroom/news/WCMS_627189/lang--en/index.htm.
Karim, E.A. & Aomar, I., 2016, Policy lessons from Okun’s Law for African countries, viewed 20 April 2022, https://www.imf.org/external/np/seminars/eng/2016/GlobalLaborMarkets/pdf/Aynaoui_Session1_paper.pdf.
Landmann, O., 2004, Employment, productivity and output growth, Employment Strategy Papers 2004/17.
Léautier, F.A. & Hanson, K., 2013, Jobless economic growth: Lessons from Africa, African Capacity Building Foundation (ACBF) Working Paper No. 25.
Leshoro, T., 2014, ‘Empirical analysis of employment elasticity of growth in Botswana’, Mediterranean Journal of Social Sciences 5(2), 171–180. https://doi.org/10.5901/mjss.2014.v5n2p171
Mkhize, N.I., 2019, ‘The sectoral employment intensity of growth in South Africa’, Southern African Business Review 23, 1–24. https://doi.org/10.25159/1998-8125/4343
Okun, A., 1962, ‘Potential output: Its measurement and significance’, in Proceeding of the business and economic statistics section of the American Statistics Society, pp. 98–104, American Statistical Association. Alexandria, Virginia.
Okun, A., 1970, The political economy of prosperity, Norton, New York, NY.
Pasipanodya, T.F.K., 2020, ‘Okun’s law: An asymmetrical approach for Zimbabwe (1991–2018)’, Journal of Economics and International Finance 12(4), 233–240. https://doi.org/10.5897/JEIF2020.1082
QUANTEC, 2022, QUANTEC database, viewed 29 April 2022, from http://www.quantec.co.za/easydata/.
Rogan, M. & Skinner, C., 2017, The nature of the South African informal sector as reflected in the quarterly labour-force survey, 2008–2014, REDI3x3 Working Paper 28.
Romeo, G., 2020, ‘Microeconomic theory in a static environment’, in Elements of numerical mathematical economics with excel: Static and dynamic optimization, pp. 295–382, Academic Press, Cambridge, MA.
Sahin, A., Tansel, A. & Berument, M.H., 2013, Output-employment relationship across sectors: A long- versus short-run perspective, IZA Discussion Paper No. 7599.
StatsSA, 2008, Quarterly Labour Force Survey (QLFS) dataset, Stats SA, Pretoria.
StatsSA, 2009, Quarterly Labour Force Survey (QLFS) dataset, Stats SA, Pretoria.
StatsSA, 2010, Quarterly Labour Force Survey (QLFS) dataset, Stats SA, Pretoria.
StatsSA, 2011, Quarterly Labour Force Survey (QLFS) dataset, Stats SA, Pretoria.
StatsSA, 2012, Quarterly Labour Force Survey (QLFS) dataset, Stats SA, Pretoria.
StatsSA, 2013, Quarterly Labour Force Survey (QLFS) dataset, Stats SA, Pretoria.
StatsSA, 2014, Quarterly Labour Force Survey (QLFS) dataset, Stats SA, Pretoria.
StatsSA, 2015, Quarterly Labour Force Survey (QLFS) dataset, Stats SA, Pretoria.
StatsSA, 2016, Quarterly Labour Force Survey (QLFS) dataset, Stats SA, Pretoria.
StatsSA, 2017, Quarterly Labour Force Survey (QLFS) dataset, Stats SA, Pretoria.
StatsSA, 2018, Quarterly Labour Force Survey (QLFS) dataset, Stats SA, Pretoria.
StatsSA, 2019, Quarterly Labour Force Survey (QLFS) dataset, Stats SA, Pretoria.
Upender, M., 2006, ‘Output elasticity of employment in the Indian economy: An empirical note’, Applied Econometrics and International Development 6(1), 197–206.
World Bank Group, April 2020, Poverty and equity brief: Sub-Saharan: South Africa, viewed 01 March 2022, from https://databankfiles.worldbank.org/public/ddpext_download/poverty/33EF03BB-9722-4AE2-ABC7-AA2972D68AFE/Global_POVEQ_ZAF.pdf.
Zhou, H. & Pindiri, C., 2015, ‘Informal sector labour demand: Evidence from Zimbabwe’s urban informal metal sector’, BOJE: Botswana Journal of Economics 13(1), 26–39.
Footnote
1. However, Okun’s law validity in the sector suggests that it has the potential to significantly contribute to the country’s employment in the long run.
|