Abstract
Background: Aid volatility has been frequently discussed but its effect on health poverty has not.
Aim: The aim of this study is to evaluate the effect of aid volatility on residential health in recipient countries.
Setting: Panel data from 91 recipient countries around the world from 1989 to 2017 were analysed.
Method: The Random Forest algorithm in machine learning was used.
Results: It reveals a conductive effect of aid volatility on public health, as aid volatility accounts for approximately 2.2%, 3.6%, 2.5% significance in child mortality, maternal mortality and life expectancy, respectively. Positive volatility causes more child mortality than negative volatility, while it causes less maternal mortality, although both kinds of volatility have a significant effect on life expectancy. Additionally, multinational aid volatility has a greater impact than bilateral volatility. The age dependency ratio affects maternal health and public life expectancy, while foreign aid and aid volatility are not crucial.
Conclusion: The donor nations or institutions should keep their aid growth-rate stable in order to ensure sustainable development in both the local economy and public health, while the recipient nations are encouraged to address these issues by developing their economy internally.
Contribution: According to a conductive effect framework developed during the research, theoretically, an indirect relationship between aid volatility and the health poverty level of recipient countries exists.
Keywords: foreign aid; aid volatility; health poverty; aid-recipient countries; machine learning method.
Introduction
A wide range of studies have demonstrated that foreign aid (FA) volatility impacts economic performance (Hudson & Mosley 2008) and poverty reduction (Alvi & Senbeta 2012). Recipient countries experience fluctuating aid flows (known as aid volatility, variability, and unpredictability) for various reasons. Arguably, aid volatility (AV) is usually defined as aid fluctuations that increase or decrease dramatically and unpredictably in the short run (Clarke, Fry & Mihajilo 2008). For instance, Bulir and Hamann (2008) observed a volatility rate of 25% on average in Africa, and 29.5% in other regions. In some studies it has been demonstrated that the volatility is non-conducive to economic growth (Boateng, Agbola & Mahmood 2021; Chauvet & Guillaumont 2009; Kathavate & Mallik 2012; Markandya, Ponczek & Soonhwa 2011; Museru, Francois & Sean 2014), and in others it is argued that this volatility interrupts poverty reduction in recipient countries (Agénor & Aizenman 2010; Maqsood & Ullah 2014).
Meanwhile, in many studies an association between FA and poverty reduction in various ways has been investigated. Some researchers argue that FA may contribute to poverty reduction (Alvi & Senbeta 2012; Mahembe & Odhiambo 2020b), while others show an insignificant association (Arvin & Barillas 2002; Boone 1996; Briggs 2017). In addition, Rajan and Subramanian (2011) showed that FA was negatively associated with poverty reduction. This is because an increase in the income from FA makes no contribution to poverty reduction if a country is suffering remarkable health poverty (Ahmed, Mustafa & Khan 2015; Alkire & Fang 2019). More studies are required to examine the relationship between FA and health poverty reduction; however, conducting predictive modelling in the relationship between FA and health poverty, using machine learning methods, is rarely discussed from the vantage point of residential health in recipient countries.
Health poverty is a multidimensional concept, referred to as multidimensional health poverty. For instance, it refers to the poverty caused by huge medical expenditures and a reduction in household income (Bredenkamp, Mendola & Gragnolati 2011; Xu et al. 2003), a lack of individual health capital and a loss of health capacity (Gweshengwe & Hassan 2020), poor child health and low nutritional levels (Ervin et al. 2017), child health interventions (Black et al. 2010), and a lack of health care (Mohantay et al. 2018). The World Bank (1990) defines poverty as the presence of poor basic social services in its ‘World Development Report 1990’. Therefore, based on this multidimensional definition, the status associated with poor health plus income poverty, referred to as health poverty, is addressed in this study.
Studying the impact of aid volatility on health poverty is valuable and gives profound insights. Generally, in a vast majority of existing literature the relationship between the aid volatility effect and economic growth is investigated (Hudson 2015; Kumi, Ibrahim & Yeboah 2017), while how the volatility impacts on residential poverty from a micro-perspective remains a puzzle. We attempted to shed new light on the relationship between FA volatility and health poverty in recipient countries by deploying econometric and machine learning models, such as the Random Forest (RF). Most of the empirical literature did their analysis employing the Hodrick Prescott (HP) filter; our study brings new dimensions and shows coping strategies in FA volatility on healthy poverty. Indeed, literature investigating from this vantage point is lacking.
Under these circumstances, we seek to bridge the gap between FA and health poverty. Firstly, this article proposes a conductive effect framework to demonstrate and further investigate the indirect relationship between aid volatility and the health poverty level of recipient countries. To the best of our knowledge, it is the first aspect of significance of this study. Secondly, the RF method of machine learning was adopted to investigate the conductive effect, assessing to what extent aid volatility affects the health poverty in recipient countries. The majority of existing studies seek to evaluate the effect of FA on poverty reduction (or economic performance) using regression models, but this kind of econometric method is ineffective to accurately evaluate the extent of influence. The RF method may compensate for its disadvantages via its precise predictions (Sohnesen & Stender 2017). In addition, the empirical models investigating poverty reduction are based on a variety of theoretical hypotheses, and a disparity between the theoretical framework and the sampling data usually arises; as a result, some results are not robust and may even be doubtful. The machine learning method may be effective to cope with this issue, without the need for theoretical hypotheses, by assessing the significance and partial dependency of the variables. As Sohnesen and Stender (2017) suggested, the RF method may yield better poverty predictions. Accordingly, this research employs the machine learning methodology to accurately evaluate the extent of influence.
Literature review and theoretical framework
Related literature
Many studies show that FA impacts the macro-economy of recipient countries. Some of them show a positive effect of FA on the recipient country’s economy. Domar (1946) argued that FA was necessary for economic development in low-income nations. This viewpoint is supported by later studies. Hansen and Tarp (2001) found that international aid could promote economic growth in recipient countries. Karras (2006) also found a positive effect based on the data from 71 recipient nations from 1960 to 1997. Levy (1988) revealed a significantly positive relationship between FA and investment, and economic development during 1968–1982 in Sub-Saharan African regions. Juselius, Møller and Tarp (2014) demonstrated the same relationship in the longer period of 1960 to 2007 in 36 African countries. In developing Asian countries, Asteriou (2009), investigated the presence of long-term relationships using panel unit tests, showing a positive effect.
In fact, FA is not as efficient as expected. Some scholars have explored the factors that impact poverty reduction. For example, Urtuzuastigui (2019) and Akobeng (2020) suggested that economic growth was subject to the institutional quality in aid-dependent countries. Wako (2018) and Maruta, Banerjee and Cavoli (2020) agreed with this point of view. In addition, Biscaye, Reynolds and Anderson (2017) argued that the effectiveness of FA was determined by the quality of other macro-economic determinants. Furthermore, Dreher, Fuchs and Langlotz (2019) showed that an increasing emigration rate contributed to more aid for the original nations of migrants. Sullivan, Blanken and Rice (2018) demonstrated that aid was being moved towards the conservation of global public services and products.
By contrast, some research uncovers that FA has only an insignificant or side effect on the recipient country’s economy. Mallik (2008) revealed that FA negatively impacts national economic growth in the long run by assessing 35 years in six African countries. Wafula, Odondo and Obange (2019) revealed an insignificant contribution to economic development in the short and long run during 1974–2017 in Kenya. These results have been confirmed by Bird and Choi (2020), showing a more ambiguous impact of FA. Additionally, FA essentially affects the institutional policies, resulting in more corruption in recipient counties, which inevitably restrains economic growth. Ali et al. (2019) examined the relationship between FA and corruption in Asian nations and revealed a remarkable negative effect on the corruption perception index in the recipient countries, showing that more FA results in more corruption. This finding is supported by Brautigam and Knack (2004) and Economides, Kalyvitis and Philippopoulos (2008).
Some studies revealed more complex results. For instance, Azam and Feng (2022) employed both fixed-effects and robust squares estimators to assess the relationship between FA and economic growth in 37 developing nations, from 1985 to 2018, and suggested that FA could contribute to economic development, but that the association was very weak in low-income nations where economic development mainly depended on exports. Additionally, FA had less positive effect on economic development in upper- middle income nations. Moreover, Abate (2022) used a generalised method of momentary assessing the effect in developing countries, and uncovered an inverted U-shaped association between FA and economic performance, indicating a negative effect when the governmental quality index and economic freedom were below the threshold value, otherwise finding a positive effect.
A controversial relationship exists between FA and poverty reduction. Some literature found that FA could contribute to poverty reduction (Alvi & Senbeta 2012; Bahmani-Oskooee & Oyolola 2009; Mahembe & Odhiambo 2020b), while others found this effect insignificant (Boone 1996; Arvin & Barillas 2002; Briggs 2017). On the contrary, Rajan and Subramanian (2011) showed an adverse effect of FA on poverty reduction, and suggested that the real exchange rate increases because of aid inflows, thus the aid-recipient countries lose their export competence. This finding partly explains why FA may not contribute to poverty reduction.
The negative effect is partially attributed to aid volatility that is harmful to the economic sustainability of the recipient countries (Houndonougbo 2017). The negative effect manifests in different ways. Bulir and Hamann (2008) argued that aid volatility could distort public investment and fiscal plans, supported by others (Celasun & Walliser 2008; Hudson 2015). Kumi et al. (2017) uncovered that aid volatility in industry significantly reduces industrial production, while Chauvet and Guillaumont (2009) revealed that aid volatility could result in economic volatility, which would interrupt the economic sustainability of the aid-dependent countries. Later studies (Boateng et al. 2021; Markandya et al. 2011) supported this viewpoint. Furthermore, the performance of governance is damaged by the range of volatility (Kangoye 2013). Meanwhile, Hudson and Mosley (2008) found that the effect is complex, given the two kinds of aid volatility (negative and positive), showing that negative volatility is more harmful to the macro-economy than positive. Therefore, the unpredictability of aid volatility may account for the fluctuation in the national economies and public welfare systems of aid-recipient countries.
To sum up, in a wide range of literature the relationship between FA and a recipient country’s economy is discussed, but few of them shed light on the effects on health poverty in the recipient countries. This research bridges that gap; it employs the RF methodology of machine learning to investigate the conductive effect, assessing to what extent aid volatility affects the health poverty of aid-dependent nations.
Theoretical framework
Although the impact of volatility in FA has on the recipient country’s economy is known, how this volatility affect residential life quality in recipient countries, observed from the micro-perspective, has not really been thoroughly researched. Thus, in this study the conductive effect is further researched. Generally speaking, FA flows into three domains: industry (fe), household (fh), and government (fg).
Taking a recipient country’s (i) FA scale (Aidi,t) in year t and its initial aid scale (Aidi,0), the following equation is constructed.

ΔAidvoli,t is the aid volatility in year t. ΔAidvoli,t ≥ 0, or ΔAidvoli,t ≤ 0. The FA increment (ΔAidi,t) can be written as follows:

The three independent variables refer to the aid volatility in industry, the household, and the government, respectively.
Figure 1 outlines the theoretical framework of the conductive effect of positive aid volatility on health poverty in three ways. When a recipient nation receives a given amount of FA from others, there is always aid variance (ΔAidit) compared to the initial aid amount, and this amount is distributed between the three areas mentioned before (fe, fh, fg).
 |
FIGURE 1: Conductive effect of positive aid volatility on health poverty. |
|
In terms of increasing aid, some money will be directly allocated to the residents, and this increases household income ( ); as a result, the residential life quality, including life health, is improved (Bayale 2020). Further, a certain amount of the aid fund is invested in industry ( ) to relieve industrial capital shortages; industrial development results in economic growth, regardless of whether the aid volatilises negatively or positively, because ΔAidit > 0. Subsequently, the growing of the economy in a recipient country will increase the household income, which may contribute to residential life health and consequently decrease health poverty. The government will also use a certain amount of the aid for public expenditures and infrastructure in order to drive the national economy ( ), which will eventually improve the national living standards, including medical treatment, although in institution-deficient countries, a considerable amount of aid is kept by the government (Ali et al. 2019).
Regardless of the ways in which the aid is distributed, it will eventually increase the household income. In this context, residents’ health will be improved. Better health could reduce medical expenditures, empower human capital, and thus increase household income, and eventually promote economic development (Banerjee, Deaton & Duflflo 2004), meaning the level of health poverty improve. Therefore, life health is crucial for sustainable economic development in a recipient country.
However, if the aid volatilises negatively, the residents will have to cut their daily expenditures, which may decrease their household income and life quality; that is, poor health + income poverty = health poverty. Additionally, volatile aid may potentially impact industrial yields and worsen the national economy, which leads to social issues, including detrimental life health. Meanwhile, negative aid volatility results in declining government expenditures in the public health system. As Hudson (2015) suggested, negative aid volatility may distort governmental expenditures, and all kinds of social issues may emerge. Therefore, negative aid volatility worsens residential life health, and consequently health deteriorates.
In conclusion, the mechanism of the impact of aid volatility on health poverty in recipient nations is complex. Aid funds are allocated to a variety of recipients in three main areas (industry, household, and government). Regardless of the area, the aid fund is ultimately to be used to improve local residents’ health, as a healthy workforce is the most fundamental and sustainable resource for economic development in a country. However, when aid fluctuates significantly, it interrupts the existent poverty reduction system, and could even break the economic sustainability of recipient countries, particularly the most aid-dependent ones, meaning the resource will be damaging at first. Therefore, aid volatility initially worsens health poverty and the recipient country’s economy.
Data
The panel data from 91 recipient countries globally (including 43 Sub-Saharan African nations) from 1989 to 2017 were collected from a few datasets. The FA data were collected from the Organization for Economic Co-operation and Development (OECD), while the other data were gathered from the World Bank and Transparency International; the aid volatility was calculated by the authors.
Using the machine learning method, the dataset was processed and sorted into two subsets: a training set and a testing set. The former comprised 70%, and the latter 30%. The models can be built and the parameters of their fitting curves determined through the training set, while the testing set is utilised to evaluate the model accuracy when predicting unknown samples.
Research methods
Figure 2 outlines this research’s framework for a mind map. Breaking it down into three steps: calculating the independent variable via the Hodrick Prescott (HP) filter method, investigating the relationship between aid volatility and life health under different circumstances, and computing the extent of the aid fluctuation effects on child and maternal mortality rates, as well as life expectancy.
The research idea is firstly to measure the extent of aid fluctuations in the 91 aid recipient countries, the results are significant (see Figure 3 in manuscript). We then use econometric methods to examine the significant relationship between aid fluctuations and health poverty. Upon the results, we adopt the machine learning method to investigate further what extent the impact is.
 |
FIGURE 3: Aid volatility for 1989 to 2017 in African recipient nations. |
|
Regression model and variables
Foreign aid volatility measurement: Following Hodrick and Prescott (1997), this study employs the HP filter method calculating the trends and periodic components of FA volatility (see Figure 2). The data-smoothing technique, used primarily in macro-economics, is used to reduce the short-run fluctuations resulting from the business cycle so as to identify long-term trends. This basic model is denoted as below.

yt represents the original signals series, gt stands for the low trend component, and ct refers to the higher-frequency period and is called the cyclical component. The series yt consists of two components: a low trend component gt and a cyclical component ct. With an appropriate coefficient λ, the trend component can be calculated by:

λ refers to the penalty parameter. The first part stands for the total squared deviations of ct, namely the cyclical component. The next part is the total squared value of the difference of the trend component (gt), which is multiplied by λ, showing variations in the growth rate of the trend component. The HP filter may detect the cyclical component ct from yt via the extent of the good fit that the trend component tracks the original series yt, against the prescribed smoothness in gt.
Evaluating the effects of aid fluctuation on health poverty, this study proposes the dependent variables of public health level denoted by child death, maternal mortality, along with public life expectancy. As these variables have lag effects on public health, the lagged vectors are included as control variables. Aid volatility is proposed as an explanatory variable, and the aid scale is taken into account as a control variable. Following Alvi and Senbeta (2012) and Chong, Gradstein and Calderon (2009), a basic regression is developed as below.

Pit refers to the health poverty level of an aid-dependent nation i in time t. Pit-1 refers to the lagged health level in the recipient country. Following Clarke and Erreygers (2020), health poverty is measured by health status and life expectancy. Goal 3 of the Sustainable Development Goals of the United Nations aims to improve the global health status by reducing the global maternal and under-5 child mortality ratios to less than 70/100 000 live births, and 25/1000 live births, respectively. Thus, this study measures health status via two variables: the maternal death rate and death rate of children under 5 years old.
Aidit stands for the FA rate (measured by the received aid amount / the GDP) of the recipient country i in year t. Aidvolit refers to the aid volatility rate (measured by the HP filter method, as elaborated above). εit refers to random errors. represents some other variables. According to Alvi and Senbeta (2012), Chong et al. (2009), and Mahembe and Odhiambo (2020a), and considering the data availability, this incorporates five more health-related variables into the regression model, which are economic level (GDP per capita), openness, inflation, age dependency ratio, and social institutions (corruption perception index). Table 1 outlines the measurements of the variables and data sources.
Random forest model
As regression methods test whether the relationship between foreign volatility and health poverty is statistically significant, but cannot examine the magnitude of the effect, machine learning methods do not require strong assumptions and could observe the magnitude of the effect aid fluctuations have on poverty from a predictive perspective, we introduce a RF model to assess the magnitude. Comparing the advantages between RF and K-Nearest Neighbor (KNN),Gradient Boosting Regression and Support Vector Machine (SVM), respectively, we found that the RF model was more robust and altogether better than others. Therefore, the RF method was used along with econometric methods to address the research question.
The RF method means a machine learning technique commonly used to deal with complex classification and regression problems with large datasets. It draws a final result and increases the decision precision based on the multiple outputs of clustered decision trees (Breiman 2001). For instance, Sohnesen and Stender (2017) have demonstrated that the RF method is more accurate than many commonly used stepwise and lasso regressions, and does facilitate better poverty predictions than regression models. Therefore, this approach was adopted to predict the potential effect of aid volatility on health poverty based on the regression results.
Supposing the relationship between a target (or response) variable Y and a number of predictors (or features), X1, X2, …, Xp.
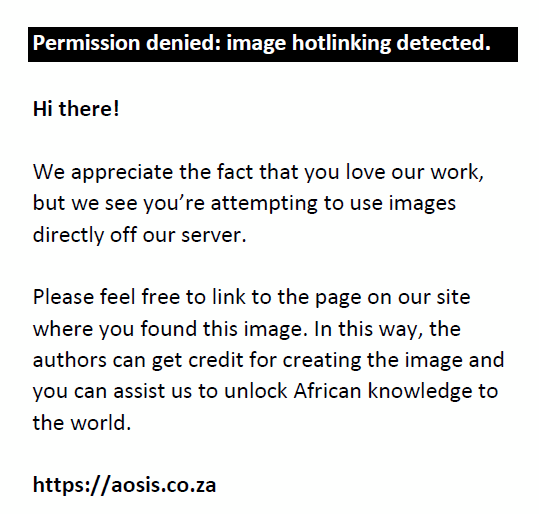
e means an error value. The value of E (Y − Ŷ)2 refers to the expected squared prediction error denoted as the value of the reducible error, calculated by . The machine learning technique may minimise the reducible error and estimate f.
The value of the response variable ŷRj is calculated by a function of the regression tree estimator, ftree (A) = Σj∈J ŷ Rj 1 {A ∈ Rj}, in which A = (X1, X2, …, Xp) represents the vector of the predictors, 1{.} stands for the indicator function, and are the regions of the predictor space obtained by minimising RSS, identifying by the mean values of the variables within the same region Rj. Therefore, denoting the number of bootstrap samples as H and using the decision tree index calculated in the sample h ∈ H by f tree (A|h), the RF index is estimated using the equation below.

The RF method is useful in predicting the effects of aid volatility on health poverty. Firstly, as an alternative to linear regression models, RF achieves higher accuracy in predicting poverty, particularly at the national level (Sohnesen & Stender 2017). Secondly, it accurately quantifies the value of the influence of particular factors, which allows its users to develop more targeted health administrations. Thirdly, the marginal effects of aid volatility on health poverty are observed in the partial dependency graphs, and this has policy and economic implications for the recipient governments.
Empirical results
Aid volatility results
Using the HP filter method, it has been calculated that the FA volatility for the period 1989 to 2017 presents the most significant findings in some recipient countries. As Figure 3 shows, there was remarkable aid volatility in most African countries during the entire period, particularly in Burundi, Central Africa, Cabo Verde, Congo, Ethiopia, Comoros, Guinea-Bissau, Sudan and Liberia. The volatility directly interrupted the economic sustainability and affected the health poverty reduction in these recipient countries.
Regression results
Table 2 shows that both the FA (Aid) and aid volatility (Aidvol) have a significant association with health poverty. Columns (1) to (6) show the negative relationships between FA and child mortality, and maternal mortality, respectively, while columns (7) to (9) show the positive association between FA and life expectancy. This result means that a growing quantity of FA may reduce both child and maternal mortality rates and promote life expectancy. However, the positive connect of aid volatility with both child and maternal mortality rates reveals that the higher the aid volatility, the higher the child and maternal mortality. By contrast, the negative association of aid volatility with life expectancy shows that significant aid volatility reduces life expectancy.
TABLE 2: Results of the basic regression analysis. |
The lagged coefficients of child and maternal mortality and life expectancy indicate that the health poverty level is significantly determined by the conditions in previous years, because the differences between the columns control variables are very slight, and there were no significant changes when including other variables. For example, we derived the following values: lagged child mortality (0.989, 0.994, 0.964), maternal mortality (0.984, 0.945, 0.960), and life expectancy (1.006, 0.985, 0.988).
Moreover, this table shows that GDP per capita has a significant association with maternal mortality and life expectancy. Inflation has no significant association with life health level. Openness is related to maternal mortality only. Age dependency influences child mortality, and the perception of corruption is connected with maternal mortality and life expectancy.
Table 3 outlines the lagged effects of aid volatility on child and maternal mortality, as well as life expectancy, in the next 2 years. Although the lagged effects are significant in columns 2–6, the connections become fewer in the following years, because these coefficients decline over the 3 years. For instance, the aid volatility coefficient decreases from 6.322 in column (3) to 5.161 in column (4). Nevertheless, both FA and aid volatility still have significant effects on health poverty in the following 2 years.
TABLE 3: The lagged effects of aid volatility on child and maternal mortality, and life expectancy. |
As the aid volatility may be positive or negative, these two kinds of contrary fluctuation will have different effects on the public health. Table 4 presents the results regarding the heterogeneity between positive and negative aid volatility. The coefficient (0.077) in column (1) shows that aid volatility has no significant effect on the child mortality rate when it fluctuates negatively (volatility < 0), while the coefficient (0.293) in column (2) indicates a significant effect when volatility > 0. This finding suggests that this effect on child death can mainly be attributed to positive aid volatility. By contrast, the coefficients (1.844 and 1.763) in columns (3) and (4) show that aid volatility may result in significant maternal mortality when volatility < 0, and that the opposite pertains when it does not, which suggests that the effect on pregnant women’s death could be attributed to negative aid volatility. Furthermore, the coefficients (–0.195 and –0.215) in columns (5) and (6) suggest that the aid volatility may significantly reduce life expectancy, regardless of whether it volatilises positively or negatively.
TABLE 4: Results regarding the heterogeneity between positive and negative aid volatility. |
Studies have shown that bilateral and multilateral aid have different effects on economic growth (Jeanneney & Tapsoba 2012; Ram 2003, 2004). It is supposed that these kinds of aid should have different effects on public health. Therefore, the HP filter method was adopted to investigate how these two kinds of aid and aid volatility affect health poverty in aid-dependent countries.
Table 5 presents the results. Generally, multinational aid volatility has greater an impact than bilateral volatility. Both bilateral and multilateral aid volatility have a significant positive effect on child and maternal mortality, which means that the two kinds of aid volatility increase the child and maternal mortality probability; therefore, multilateral aid volatility (2.378, 13.744) is riskier than the bilateral kind (2.509, 11.626). The two kinds of aid volatility have a negative effect on life expectancy, which means that volatility may result in a decline in life expectancy, while multilateral aid is stronger (–0.796) than the bilateral kind (–0.688).
TABLE 5: Results of heterogeneity between multilateral and bilateral aid volatility. |
Meanwhile, this table indicates that reductions in the two kinds of FA will result in more child and maternal deaths and a declining life expectancy. Similarly, multilateral FA has more significant effects on public health than the bilateral kind.
Random Forest analysis
The aforementioned results have demonstrated the effect of aid volatility on child and maternal mortality, as well as life expectancy. In this study the RF method to evaluate the extent of these effects has been developed further.
Effect of aid volatility on child mortality
In Figure 4 the goodness of fit between RF prediction and its real values is visualised. The predicted child mortality rates (pred) closely fit the real-sample values (y_test) with a very significant goodness of fit (0.998 degrees). Therefore, the RF method is reliable for use in investigating the effects of FA volatility on child mortality.
 |
FIGURE 4: Goodness of fit between predicted and real values of child mortality. |
|
Figure 5 shows the significance values of the determinants influencing child mortality. The lagged child mortality rate overwhelmingly dominates this ranking, representing 0.481 of the contributions, indicating a strong association between the rates in the following years. The second factor is the age dependency ratio, with a value of 0.177, which means elderly care may relate to an increasing child mortality rate. Followed by the GDP per capita, the growing economy may reduce the rate of child mortality. Foreign aid (Aid) is the fourth most significant factor, with a value of 0.067, while the aid volatility (Aidvol) has an effect value of 0.022 on child mortality.
 |
FIGURE 5: Ranking of the most significant factors affecting child mortality. |
|
A partial dependency graph is used to present the extent to which FA volatility determines child mortality. Supposing that:
x = (Aidvol, Aid,…)
y = Child mortality rate = f (Aidvol,Aid,…),
a marginal effect of the first variable (x1) (Aidvol) on child mortality (y) can be calculated by the RF model:
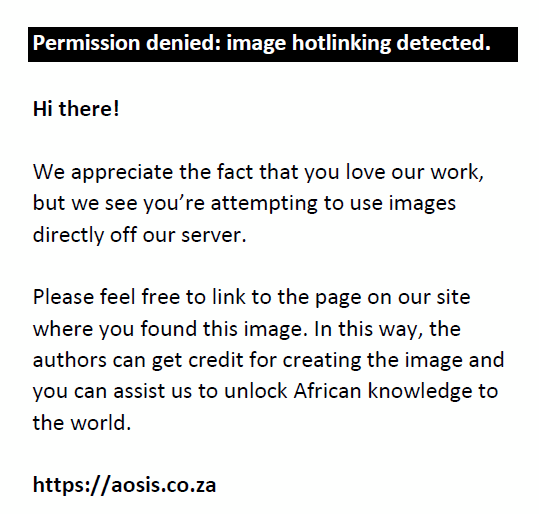
This is developed further as follows:
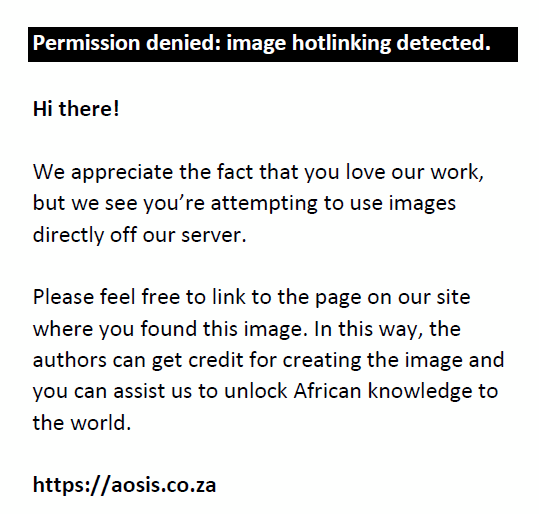
With any eigenvalue , a partial dependency graph can be constructed from the value groups ( )
Figure 6 shows the partial dependency graph, showing a V-shaped relationship between aid volatility and child mortality. The child mortality rate decreases to its lowest level when the aid volatility is zero, while the rate increases when |Aidvol|>0, regardless of whether the aid volatility increases to the right side or declines to the left side. Additionally, there is an asymmetrical effect between the two sides. The effect is greater when the volatility declines, compared to when it increases, which means that an aid reduction has a more significant negative effect on the child mortality rate than aid growth. For instance, the child mortality rate will increase by 69 units when the aid volatility decreases towards the left by 1.5 units, while the rate will grow by 65 units.
 |
FIGURE 6: Partial dependency of the aid volatility effect on child mortality. |
|
Effect of aid volatility on maternal mortality
In terms of the maternal mortality rate, the RF method is employed to examine the goodness of fit between the predicted and real values, with the same fitting degree of 0.996 (see Figure 7).
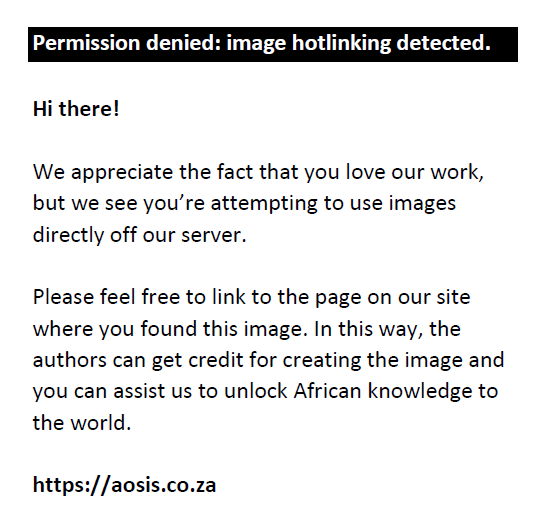 |
FIGURE 7: Goodness of fit between predicted and real values of maternal mortality. |
|
Figure 8 shows the significance of the determinants of maternal mortality. Similarly, the lag maternal mortality rate (0.443) dominates this ranking, indicating a strong association between the rates in the coming years, age dependency ratio (0.189), with GDP (Gross Domestic Product) per capita (0.156) following it. The aid volatility (0.036) is ranked sixth.
 |
FIGURE 8: Ranking of the significant factors affecting maternal mortality. |
|
Similarly, Figure 9 shows a V-shaped relationship between aid volatility and maternal mortality. An asymmetrical effect exists between the two sides. The effect is much more dominant on the left side than on the right. For example, the maternal mortality rate will increase by 370 units when the aid volatility decreases on the left by 1.5 units, while the rate will grow by 330 units.
 |
FIGURE 9: Partial dependency of aid volatility effect on maternal mortality. |
|
Effect of aid volatility on life expectancy
Figure 10 indicates the perfect goodness of fit (0.998) between the predicted (pred) and real sample values (y_test). Figure 11 indicates that the aid volatility has an effect value of 0.025 on life expectancy.
 |
FIGURE 10: Goodness of fit between predicted and real values of life expectancy. |
|
 |
FIGURE 11: The significant factors affecting life expectancy. |
|
On the other hand, Figure 12 shows an inverted V-shaped relationship between aid volatility and life expectancy, and an asymmetrical effect between the two sides. The life expectancy reaches its peak when the aid volatility is zero, while the rate increases positively and negatively as |Aidvol|> 0, according to which the life expectancy will decline remarkably towards the left side. For instance, the life expectancy will decline to 63.5 when the aid volatility decreases on the left by 1 unit, while it drops to 64.5 on the right with a 1-year gap. Therefore, aid volatility potentially reduces human life expectancy.
 |
FIGURE 12: Partial dependency of aid volatility effect on life expectancy. |
|
Table 6 summarises the sequence of significant factors and compares the values of aid volatility’s effects on health poverty. Aid volatility has a more significant effect on both maternal mortality (3.6%) and life expectancy (2.5%) than child mortality (2.2%). The aid volatility has a V-shaped partial dependency on both child and maternal mortality, but an inverted V-shape relation to life expectancy. Nevertheless, the RF method has a perfect goodness of fit (0.98–0.99). Thus, this metric does address the research question. In addition, the sequence of the significant factors confirms the conductive effect hypothesis, according to which the resident health level in a recipient country is mainly determined by its economic strength, which is impacted by the aid volatility in the particular country.
TABLE 6: Comparison of the significant factors and the values of aid volatility’s effects on health poverty. |
Conclusions
The aim of this research was to evaluate the effect of aid volatility on residential health in recipient countries, and this objective has been answered by collecting FA data from 91 recipient countries from the period 1989 to 2017. The RF method has been employed as a machine learning technique to evaluate and give a new perspective on the effect of aid volatility on residential health in recipient countries. The findings are as follow:
Foreign aid volatilises in the majority of recipient countries. A positive relationship between aid volatility and child death, maternal health was found. We found a conductive effect of aid volatility on local health poverty; particularly, aid volatility accounts at approximately 2.2%, 3.6%, 2.5% significance regarding child mortality, maternal mortality and life expectancy, respectively, in the recipient countries. Positive volatility results in more child mortality than negative volatility, while it results in less maternal mortality; and both kinds of volatility have a significant effect on life expectancy. Additionally, multinational aid volatility has a greater impact than bilateral volatility.
National economic strength significantly affects child health, while the age dependency ratio remarkably affects maternal health and public life expectancy. In contrast, FA and aid volatility are not the most crucial determinants. Meanwhile, a lagged effect of aid volatility is felt in local health poverty.
This research has potential health and economic implications for policy makers, enabling them to take further steps to improve wellness in recipient countries. Firstly, the international community, especially developed countries, should reduce the fluctuation of aid funds, because aid fluctuations have a negative impact on the health of impoverished countries, and both negative and positive aid fluctuations are detrimental to poverty reduction in recipient countries. Therefore, countries should strive to maintain stability and a steady growth in aid funds, avoiding significant changes, to maximise the effectiveness of aid. Meanwhile, the international community should urge developed countries to enhance the stability of aid funds.
To reduce aid volatility, developed countries could adopt a multilateral approach, reducing the proportion of bilateral aid. The international community should strengthen aid coordination to avoid concentrated inflows and outflows of aid funds, ultimately reducing aid fluctuations. Transparency in aid information should also be increased, enabling recipients to plan their budgets based on anticipated increases or decreases in external funding to mitigate the negative impact of external fund fluctuations.
Secondly, international organisations should play an active role in raising, distributing, and coordinating aid funds. The advantages of international organisations should be leveraged in aid fundraising, distribution, project coordination, information dissemination, and aid assessment, actively guiding aid donors and recipients worldwide to allocate aid funds scientifically and strengthen aid management, fundamentally reducing the negative impact of aid fluctuations.
Thirdly, achieving poverty reduction in recipient countries, it is mainly dependent on the strength of the recipient country itself, whether the impact of external aid is limited. Therefore, the international community should uphold the aid concept of ‘giving a fish is not as good as teaching him how to fish’.
This article has two research limitations. While it is based on national economic data, future studies should perhaps investigate this issue from a regional perspective, because the volatile effect should be more significant in underdeveloped countries and towns. Additionally, a limited number of variables has been used due to data availability, and more factors should be considered in further studies.
Acknowledgements
Competing interests
The authors have declared that no competing interest exists.
Authors’ contributions
Q.X. was in charge of conceptualisation, methodology, formal analysis, writing of original draft, and funding acquisition. L.L. was in charge of investigation, project administration, software, data duration, and resources. H.L. played a role in methodology, formal analysis software, as well as writing, review and editing.
Ethical considerations
Ethical waiver was received from Jiangxi Science and Technology Normal University with the ethical clearance number 2023040305.
Funding information
Author Q.X. received funding from The National Social Science Fund of China (Grant number: 18BJL103).
Data availability
The data that support the findings of this study are available from the corresponding author, [H.L.], upon reasonable request.
Disclaimer
Opinions expressed in the submitted article are our own and not an official position of the institution or funder.
References
Abate, C.M., 2022, ‘The relationship between aid and economic growth of developing countries: Does institutional quality and economic freedom matter?’, Cogent Economics & Finance 10(1), 2062092. https://doi.org/10.1080/23322039.2022.2062092
Agénor, P.R. & Aizenman, J., 2010, ‘Aid volatility and poverty traps’, Journal of Development Economics 91(1), 1–7. https://doi.org/10.1016/j.jdeveco.2009.03.003
Ahmed, R., Mustafa, U. & Khan, A.U., 2015, ‘Socio-economic status of transferred and non-transferred urban slums: A case study from Faisalabad’, Pakistan Development Review 54(2), 947–962. https://doi.org/10.30541/v54i4I-IIpp.947-962
Akobeng, E., 2020, ‘Harnessing foreign aid for the poor: Role of institutional democracy’, Journal of Economic Studies 47(7), 1689–1710. https://doi.org/10.1108/JES-05-2019-0225
Alvi, E. & Senbeta, A., 2012, ‘Does foreign aid reduce poverty’, Journal of International Development 24(8), 955–976. https://doi.org/10.1002/jid.1790
Ali, M., Khan, L., Sohail, A. & Puah, C.H., 2019, ‘The relationship between foreign aid and corruption: A case of selected Asian countries’, Journal of Financial Crime 26(3), 692–704. https://doi.org/10.1108/JFC-08-2018-0089
Alkire, S. & Fang, Y., 2019, ‘Dynamics of multidimensional poverty and uni-dimensional income poverty: An evidence of stability analysis from China’, Social Indicators Research 142(1), 25–64. https://doi.org/10.1007/s11205-018-1895-2
Arvin, B.M. & Barillas, F., 2002, ‘Foreign aid, poverty reduction, and democracy’, Applied Economics 34(17), 2151–2156. https://doi.org/10.1080/00036840210136718
Asteriou, D., 2009, ‘Foreign aid and economic growth: New evidence from a panel data approach for five South Asian countries’, Journal of Policy Modeling 31(1), 155–161. https://doi.org/10.1016/j.jpolmod.2008.04.012
Azam, M. & Feng, Y., 2022, ‘Does foreign aid stimulate economic growth in developing countries? Further evidence in both aggregate and disaggregated samples’, Quality and Quantity 56, 533–556. https://doi.org/10.1007/s11135-021-01143-5
Bahmani-Oskooee, M. & Oyolola, M., 2009, ‘Poverty reduction and aid: Cross-country evidence’, International Journal of Sociology and Social Policy 29(5/6), 264–273. https://doi.org/10.1108/01443330910965796
Banerjee, A., Deaton, A. & Duflflo, E., 2004, ‘Wealth, health, and health services in rural Rajasthan’, American Economic Review 94(2), 326–330. https://doi.org/10.1257/0002828041301902
Bayale, N., 2020, ‘Foreign aid and fiscal resources mobilization in WAEMU countries: Ambiguous effects and new questions’, African Journal of Economic Review 8(2), 17–38.
Bird, G. & Choi, Y., 2020, ‘The effects of remittances, foreign direct investment, and foreign aid on economic growth: An empirical analysis’, Review of Development Economics 24(1), 1–30. https://doi.org/10.1111/rode.12630
Biscaye, P.E., Reynolds, T.W. & Anderson, C.L., 2017, ‘Relative effectiveness of bilateral and multilateral aid on development outcomes’, Review of Development Economics 21(4), 1425–1447. https://doi.org/10.1111/rode.12303
Black, R.E., Cousens, S., Johnson, H.L., Lawn, J.E., Rudan, I., Bassani, D.G. et al., 2010, ‘Global, regional, and national causes of child mortality in 2008: A systematic analysis’, The Lancet 375(9730), 1969–1987. https://doi.org/10.1016/S0140-6736(10)60549-1
Boateng, E., Agbola, F.W. & Mahmood, A., 2021, ‘Foreign aid volatility and economic growth in Sub-Saharan Africa: Does institutional quality matter?’, Economic Modelling 96, 111–127. https://doi.org/10.1016/j.econmod.2020.12.032
Boone, P., 1996, ‘Politics and the effectiveness of foreign aid’, European Economic Review 40(2), 289–329. https://doi.org/10.1016/0014-2921(95)00127-1
Brautigam, D.A. & Knack, S., 2004, ‘Foreign aid, institutions, and governance in Sub-Saharan Africa’, Economic Development and Cultural Change 52(2), 255–285. https://doi.org/10.1086/380592
Bredenkamp, C., Mendola, M. & Gragnolati, M., 2011, ‘Catastrophic and impoverishing effects of health expenditure: New evidence from the Western Balkans’, Health Policy Plan 26(4), 349–356. https://doi.org/10.1093/heapol/czq070
Breiman, L., 2001, ‘Random forests’, Machine Learning 45(1), 5–32. https://doi.org/10.1023/A:1010933404324
Briggs, R.C., 2017, ‘Does foreign aid target the poorest?’, International Organization 71(1), 187–206. https://doi.org/10.1017/S0020818316000345
Bulir, A. & Hamann, A.J., 2008, ‘Volatility of development aid: From the frying pan into the fire?’, World Development 36(10), 2048–2066. https://doi.org/10.1016/j.worlddev.2007.02.019
Celasun, O. & Walliser, J., 2008, ‘Predictability of aid: Do fickle donors undermine aid effectiveness’, Economic Policy 23(55), 546–594. https://doi.org/10.1111/j.1468-0327.2008.00206.x
Chauvet, L. & Patrick, G., 2009, ‘Aid, volatility, and growth again: When aid volatility matters and when it does not’, Review of Development Economics 13(3), 452–463. https://doi.org/10.1111/j.1467-9361.2009.00501.x
Chong, A., Gradstein, M. & Calderon, C., 2009, ‘Can foreign aid reduce income inequality and poverty?’, Public Choice 140(1/2), 59–84. https://doi.org/10.1007/s11127-009-9412-4
Clarke, M., Fry, T. & Mihajilo, S., 2008, ‘The volatility of aid to small island states’, Pacific Economic Bulletin 23(2), 179–202.
Clarke, P. & Erreygers, G., 2020, ‘Defining and measuring health poverty’, Social Science & Medicine 244, 112633. https://doi.org/10.1016/j.socscimed.2019.112633
Domar, E.D., 1946, ‘Capital expansion, rate of growth, and employment’, Econometrica 14(2), 137–147. https://doi.org/10.2307/1905364
Dreher, A., Fuchs, A. & Langlotz, S., 2019, ‘The effects of foreign aid on refugee flows’, European Economic Review 112, 127–147. https://doi.org/10.1016/j.euroecorev.2018.12.001
Economides, G., Kalyvitis, S. & Philippopoulos, A., 2008, ‘Does foreign aid distort incentives and hurt growth? Theory and evidence from 75 aid-recipient countries’, Public Choice 134, 463–488. https://doi.org/10.1007/s11127-007-9239-9
Ervin, P.A., De Ervin, L.G., Vega, J.R.M. & Sacco, F.G., 2017, ‘Multidimensional poverty in Paraguay: Trends from 2000 to 2015’, Social Indicators Research 140(3), 1035–1076. https://doi.org/10.1007/s11205-017-1795-x
Gweshengwe, B. & Hassan, N.H., 2020, ‘Defining the characteristics of poverty and their implications for poverty analysis’, Cogent Social Science 6(1), 1768669. https://doi.org/10.1080/23311886.2020.1768669
Hansen, H. & Tarp, F., 2001, ‘Aid and growth regressions’, Journal of Development Economics 64(2), 547–570. https://doi.org/10.1016/S0304-3878(00)00150-4
Hodrick, R.J. & Prescott, E.C., 1997, ‘Postwar U.S. business cycles: An empirical investigation’, Journal of Money, Credit, and Banking 29(1), 1–16. https://doi.org/10.2307/2953682
Houndonougbo, A.N., 2017, ‘Aid volatility and real business cycles in a developing open economy’, Southern Economic Journal 83(3), 756–773. https://doi.org/10.1002/soej.12178
Hudson, J., 2015, ‘Consequences of aid volatility for macroeconomic management and aid effectiveness’, World Development 69, 62–74. https://doi.org/10.1016/j.worlddev.2013.12.010
Hudson, J. & Mosley, P., 2008, ‘Aid volatility, policy and development’, World Development 36(10), 2082–2102.
Jeanneney, S.G. & Tapsoba, S., 2012, ‘Aid and income stabilization’, Review of Development Economics 16(2), 216–229. https://doi.org/10.1111/j.1467-9361.2012.00657.x
Juselius, K., Møller, N.F. & Tarp, F., 2014, ‘The long-run impact of foreign aid in 36 African countries: Insights from multivariate time series analysis’, Oxford Bulletin of Economics and Statistics 76(2), 0305–9049. https://doi.org/10.1111/obes.12012
Kangoye, T., 2013, ‘Does aid unpredictability weaken governance? Evidence from developing countries’, Developing Economies 51(2), 121–144.
Karras, G., 2006, ‘Foreign aid and long-run economic growth: Empirical evidence for a panel of developing countries’, Journal of International Development 18(1), 15–28. https://doi.org/10.1002/jid.1187
Kathavate, J. & Girijasankar, M., 2012, ‘The impact of the interaction between institutional quality and aid volatility on growth: Theory and evidence’, Economic Modelling 29(3), 716–724. https://doi.org/10.1016/j.econmod.2012.01.020
Kumi, E., Ibrahim, M. & Yeboah, T., 2017, ‘Aid, aid volatility and sectoral growth in Sub-Saharan Africa: Does finance matter?’, Journal of African Business 18(4), 435–456. https://doi.org/10.1080/15228916.2017.1363358
Levy, V., 1988, ‘Aid and growth in Sub-Saharan Africa: The recent experience’, European Economic Review 32(9), 1777–1795. https://doi.org/10.1016/0014-2921(88)90085-2
Mahembe, E. & Odhiambo, N.M., 2020a, ‘Development aid and its impact on poverty reduction in developing countries: A dynamic panel data approach’, International Journal of Development Issues 19(2), 145–168. https://doi.org/10.1108/IJDI-08-2019-0144
Mahembe, E. & Odhiambo, N.M., 2020b, ‘Does foreign aid reduce poverty? A dynamic panel data analysis for Sub-Saharan African countries’, The Journal of Economic Inequality 19(4), 875–893. https://doi.org/10.1007/s10888-021-09496-5
Mallik, G., 2008, ‘Foreign aid and economic growth: A cointegration analysis of the six poorest African countries’, Economic Analysis and Policy 38(2), 251–260. https://doi.org/10.1016/S0313-5926(08)50020-8
Maqsood, F. & Ullah, S., 2014, ‘Foreign aid volatility and socio-economic dimensions of human development index: A case study of Pakistan’, Pakistan Journal of Social Sciences 34(1), 59–67.
Markandya, A., Ponczek, V. & Soonhwa, Y., 2011, ‘What are the links between aid volatility and growth?’, The Journal of Developing Areas 44(2), 41–68. https://doi.org/10.1353/jda.0.0105
Maruta, A.A., Banerjee, R. & Cavoli, T., 2020, ‘Foreign aid, institutional quality and economic growth: Evidence from the developing world’, Economic Modelling 89(1), 444–463.
Mohantay, S.K., Rasul, G., Mahapatra, B., Choudhury, D., Tuladhar, S. & Holmgren, E.V., 2018, ‘Multidimensional poverty in mountainous regions: Shan and Chin in Myanmar’, Social Indicators Research 138(1), 23–44. https://doi.org/10.1007/s11205-017-1662-9
Museru, M., Francois, T. & Sean, G., 2014, ‘The impact of aid and public investment volatility on economic growth in Sub-Saharan Africa’, World Development 57(5), 138–147. https://doi.org/10.1016/j.worlddev.2013.12.001
Rajan, R.G. & Subramanian, A., 2011, ‘Aid, Dutch disease, and manufacturing growth’, Journal of Development Economics 94(1), 106–118.
Ram, R., 2003, ‘Roles of bilateral and multilateral aid in economic growth of developing countries’, Kyklos 56(1), 95–110. https://doi.org/10.1111/1467-6435.00211
Ram, R., 2004, ‘Recipient country’s “policies” and the effect of foreign aid on economic growth in developing countries: Additional evidence’, Journal of International Development 16(2), 201–211. https://doi.org/10.1002/jid.1071
Sohnesen, T.P. & Stender, N., 2017, ‘Is Random Forest a superior methodology for predicting poverty? An empirical assessment’, Poverty & Public Policy 9(1), 118–133.
Sullivan, P.L., Blanken, L.J. & Rice, I.C., 2018, ‘Arming the peace: Foreign security assistance and human rights conditions in post-conflict countries’, Defence and Peace Economics 31(2), 1–24. https://doi.org/10.1080/10242694.2018.1558388
The World Bank, 1990, World development report 1990, Oxford University Press, Washington DC.
Urtuzuastigui, G.A., 2019, ‘Bilateral aid in sub-Saharan Africa: Are donor delivery tactics stimulating economic growth and development?’, Journal of Contemporary African Studies 37(1), 128–147. https://doi.org/10.1080/02589001.2018.1541502
Wafula, M., Odondo, A. & Obange, N., 2019, ‘Effect of foreign multilateral aid and foreign bilateral aid on capital formation in Kenya’, Journal of Economics and Development Studies 7(3), 1–16. https://doi.org/10.15640/jeds.v7n3a1
Wako, H.A., 2018, ‘Aid, institutions and economic growth in sub-Saharan Africa: Heterogeneous donors and heterogeneous responses’, Review of Development Economic 22(1), 23–44. https://doi.org/10.1111/rode.12319
Xu, K., Evans, D.B., Kawabata, K., Zeramdini, R., Klavus, J. & Murray, C.J.L., 2003, ‘Household catastrophic health expenditure: A multicountry analysis’, The Lancet 362(9378), 111–117. https://doi.org/10.1016/S0140-6736(03)13861-5
|