Abstract
Background: The scourge of malnutrition in Africa is a significant obstacle to children’s education, impeding their learning ability and perpetuating poor health.
Aim: In line with Millennium Development Goals (MDG) 1 and 2 and Sustainable Development Goals (SDG) 1 and 4 objectives, the study examined the impact of malnutrition on education inequality before and during development goals, in some selected African countries, based on data availability.
Setting: The analysis covered the period of 1998–2020 and the data for the study were sourced from the United Nations International Children’s Emergency Fund (UNICEF), World Health Organization (WHO), and World Bank Online Data Base among other sources.
Method: The authors employ a continuous wavelet coherence analytical tool that considers time–frequency variations in the co-movement to examine the correlation between the series in 28 African countries from 1998 to 2020.
Results: Results revealed a positive high-to-low-frequency co-movement between the two series, with malnutrition causing educational inequality from 1998 to 2010, and yet disappearing in subsequent periods. All in all, our findings indicate that malnutrition had a positive effect on educational inequality until the year 2010.
Conclusion: The authors conclude that high malnutrition rates impeded educational equality in numerous African countries during the 1990s and 2000s. However, efforts to eradicate hunger, policy intervention in education, and other factors may have altered this narrative in the post-2010 era.
Contribution: While previous African studies focused on child growth, the gender gap in education, and the incidence of poverty, completely neglecting the malnutrition variables, in this study a more profound understanding of education inequality in Africa is provided and the correlation between malnutrition and education inequality is considered which was not covered by previous authors.
Keywords: education inequality; malnutrition; wavelet coherence; partial wavelet coherence; Africa.
Introduction
Access to primary education in Africa has undergone a fundamental transformation since the Millennium Development Goals (MDG) and Sustainable Development Goals (SDG) were initiated, as reflected in the upward trend of primary school enrolment from the year 2000 (see Figure 2). The current primary enrolment rate stands at a staggering 98.75%, gradually closing the gap between the world average (World Development Indicator [WDI] 2022). However, while significant success can be said to be recorded in primary school enrolment, access to education beyond the primary level in many African countries still largely depends on household income, limiting the poor from accessing quality education, and thereby creating a huge educational gap (Rasaq 2022). In 2018, according to the United Nations (UNDP), secondary school enrolment in African countries ranged between 30% to 50%, while tertiary enrolment was as low as 7% in some African countries (UNDP 2018). Also, the recent United Nations Educational, Scientific and Cultural Organization (UNESCO) report, published in 2022, shows that out of 244 million children, aged between 6–18, currently out of school, more than 40%, or 98 million live in sub-Saharan Africa, citing family and school circumstances among the main causes of absenteeism (UNESCO 2022). However, while family factors that jeopardise children’s access to quality education include, among others, ill health, and family income level, malnutrition has a significant impact on children’s education and its effect could be permanent (Union 2022).
Malnutrition is a ‘lack of proper nutrition or bad nutrition’ which includes both the cases of stunting and obesity. While the case of obesity is more pronounced in developed countries, stunting has reduced to 30.7% in Africa; nevertheless, the percentage is still higher than the global average (United Nations International Children’s Emergency Fund [UNICEF] 2023). Malnutrition impairs growth and affects the physical function of an individual body to the point where an individual can no longer maintain adequate bodily performance processes (WFP 2000). In children, malnutrition affects children not only at birth but the fetus during pregnancy as well; one thousand days from the beginning of pregnancy and a child’s second birthday is usually referred to as the critical period for brain development when a high-nutritional intake for both mother and child at birth is critical (Union 2022). Malnutrition is the consequence of chronic poverty and hidden hunger; the concepts are like puzzles that may be used interchangeably and seem to reinforce one another in a vicious cycle. Malnutrition is a lack of access to food security which is associated with individuals with an extremely low income and the burden of poverty (Siddiqui et al. 2020). While malnutrition arising from poverty could directly keep children out of school, malnutrition during the brain development process could indirectly affect the learning ability, interaction, and how children engage with the world, creating school enrolment inequality and intellectual inequality (Union 2022; World Health Organization [WHO] 2014).
Therefore, given objectives 1 and 2 of MDG in eradicating hunger and making basic education accessible to all, the SDG 1 and 4 goal with similar objectives, as reflected in the statistical publication of the Food and Agriculture Organization (FAO) of the United Nations, which shows that the number of people living with hunger has reduced to 257 million in Africa, representing just 21%, as against the 24% in the year 2000; WHO 2019 publication that additionally shows that stunting has decreased among African children from 38.3% to 30.3% between 2000 to 2017, as well as the upward trend of primary enrolment and secondary completion rate in Africa as revealed in World Bank indicator (WBI) statistics (Bongaarts, 2021; WDI 2022). We hypothesise a correlation between malnutrition and educational inequality in Africa; check the relationship between the variables prior to and during trying to reach MDG and SDG goals, and lastly, we control the influence of the Gross Domestic Product Growth Rate (GDPG) on both malnutrition and educational inequality to check the likely effect of GDP growth on the two variables. This is important to evaluate since several initiatives and policies, such as the MDG and SDG, aimed at reducing malnutrition in Africa, have been initiated over the last few decades, including school feeding programmes geared towards enhancing children’s cognitive capacity, promoting school attendance, and addressing the gender gap in education by targeting factors that impede girls’ access to education (Gelli, Meir & Espejo 2007).
Notwithstanding the fact that the existing literature on the causes and effects of malnutrition in Africa, focusing on children’s growth, has been examined in several studies such as Bain et al. (2013), Fotso (2007), Harttgen, Klasen and Vollmer (2013), Vollmer et al. (2014), Rashad and Sharaf (2018), and Amugsi, Dimbuene and Kyobutungi (2019), no study has been found to directly examine the effect of malnutrition on educational inequality in Africa. However, we do acknowledge that some previous studies explored the relationship between poverty and education inequality in Africa (Buchmann 1999), assessing the role of school feeding programmes and nutritional status (Chabite et al. 2018), as well as evaluating the regional effects of school meal programmes in Africa (Wineman et al. 2022). Our study builds on these previous works by examining the impact of malnutrition on educational inequality in a sample of African countries.
To ensure a robust empirical analysis, we employ the wavelet coherence analysis which is a formidable analytical tool for considering the time–frequency variations in the co-movement between malnutrition and the Gini Coefficient for Education. Therefore, the analysis remains robust to structural beaks and cyclical co-movements, caused by varying lengths of economic cycles, and further considers causality effects due to the phase-difference dynamics established within the synchronisations. We further perform sensitivity analysis by controlling for GDP growth rate, within a multivariate time – frequency framework, via partial wavelet coherence analysis. Overall, our findings confirm a positive high-to-low-frequency co-movement between malnutrition and educational inequality between 1988 and 2010 and yet disappeared in subsequent periods. These findings hold for most of our sample even after controlling for the effects of economic growth. We interpret these findings to reflect the success of global and local initiatives taken to improve levels of malnutrition and inequality in Africa, although these efforts pushed the trajectory of the variables away from their common equilibrium. The associated policy implications are further discussed.
The article is structured as follows: the introduction is followed by overviews of concepts of education inequality in Africa in the ‘Overview of malnutrition and educational inequality in Africa’ section. The ‘Literature review’ section presents both the theoretical and empirical literature. The ‘Methodology’ section outlines the methodology adopted. The ‘Data and results’ section presents the data and empirical findings, followed by the conclusion that brings the article to a close.
Overview of malnutrition and educational inequality in Africa
Overview of malnutrition in Africa
Malnutrition is a well-documented consequence of hunger and poverty (Bain et al. 2013). It results from marginal dietary intake, inadequate access to potable water, good sanitation, and a lack of the required minimum money to guarantee access to sustainable food. Malnutrition is also compounded by being denied access to basic amenities that could enhance sustainability and living in a poor environment (Rasaq 2022; Setboonsarng 2005). While income alone cannot adequately measure chronic poverty, malnutrition is a good indicator to measure poverty as it reflects the level of hunger in a country via the affected individual health status. The World Health Organization (2020) stressed that malnutrition is both an economic and social issue affecting not only developing countries, but the dimension of malnutrition is also found in developed countries. Malnutrition affects both adults and children but malnutrition in children below the age of five is more common (UNICEF & WHO 2020). Statistically, WHO shows that out of 1.9 billion people who are overweight, 41 million are children the age of five. The statistics also revealed that 159 million people are stunted, and 462 million individuals are underweight (WHO 2020). Malnutrition is more prevalent in African countries because many poor households often opt for cheaper, less nutritious foods and consume more food packed with high cholesterol due to their financial status. Others do not even have enough income to purchase the cheapest staple food, increasing the number of underweight individuals (Rasaq 2022; Bain et al., 2013).
According to UNICEF (2023), the global figure of stunting has decreased from 33% to 22.3% in 2022 in the space of 23 years, and the undernourished reduced to 278 million. Nevertheless, malnutrition is still on the high side in Africa due to the prevalence of poverty in the region and an increase in the cost of living. Poverty and the high cost of living have forced over 1.4 million children to live with stunting in Eastern and Southern Africa; also in West and Central Africa, more than 6.5 million people are stunted due to the high cost of living (Rasaq 2022; UNICEF 2023). Figure 1 (based on data collected from UNICEF and the World Bank) shows the time-series data for malnutrition in sub-Saharan Africa, indicating an increase in malnutrition over the last two decades, with a slowdown in growth after 2010.
Dimensions of educational inequality in Africa
Countries within the African continent have made commendable progress in achieving a significant milestone in school enrolment, as reflected in the World Bank dataset (WDI 2022), particularly in primary education, as demonstrated by the narrowing of the primary school enrolment gap with other regions of the world, as illustrated in Figure 2. However, despite the increasing access to basic primary education, education inequality remains prevalent on the continent, primarily determined by household income and other related factors (Musau 2018).
 |
FIGURE 2: Trends of primary school enrolment in Africa. |
|
Figure 2 illustrates the trends in primary school enrolment in sub-Saharan Africa, sourced from the WBI in 2022. Education inequality in Africa manifests in different forms, with income-based education inequality being the most common (Nabassaga et al. 2020). While many African governments and non-governmental organisations provide basic primary education, access to secondary and tertiary education is usually determined by a family’s financial capacity and parental education background (UNICEF, 2023). However, the significant income inequality gap present in many African societies, as demonstrated in Figure 3, perpetuates inequality in secondary and tertiary institutions (World Inequality Report [WIR] 2022).
Figure 3 displays the income share by region and is sourced from the WIR (2022). Education inequality also exists between urban and rural areas in Africa in terms of the quality of education provided. The myriad of challenges faced by rural schools include a shortage of qualified teachers, lack of adequate support from the community and parents, infrastructure being dilapidated, shortage of government funding and bad roads, which often restrict accessibility to school (Rasaq 2022). These highlighted factors, among others, reduce the quality of education in rural communities and create an education quality gap as noted by the UN Research Institute for Social Development (2005).
Gender-based education inequality is another dimension of inequality present in many African countries. The school attendance odds always favour the boys due to African cultures and norms being unfavourable towards female education (Ombati & Ombati 2012). This disparity is evident in the enrolment ratio of boys to girls in Africa, as illustrated in Figure 4, which presents secondary school enrolments in sub-Saharan Africa. The data are sourced from the WDI in 2021, and the graph is presented by the author.
 |
FIGURE 4: Secondary school enrolment in Africa. |
|
Measuring educational inequality
The aim with measuring inequality in education is to highlight the uneven distribution of academic resources across different demographic groups such as gender, region, and socioeconomic status. Various measurement concepts have been used in the literature to assess education inequality with the common ones, including school enrolment ratios and completion ratios. Another method ranked the quality of education by the quantity of resources that go into education sectors and the year of schooling. However, the Education Gini Index remains the preferred choice due to its ability to provide a more accurate indicator of school distribution inequality. Like the conventional Gini Coefficient, the Education Gini Index combines three education indicators (enrolment, attainment, and average years of schooling data) to measure the gap in education. The value of the Gini Index of Education ranges from zero to one (Rasaq 2022).
Early design of the Education Gini Index relies on school enrolment and financing data for its computation, but Thomas, Wang and Fan (2002) are the first authors to design the index with education attainment, years of schooling and human capital stock data, as provided in Barro & Lee publications, Psacharopoulos & Arriagada publication and Nehru, Swanson & Dubey article, respectively (Barro & Lee, 1993, 1996; Nehru, Swanson & Dubey 1995; Psacharopoulos & Arriagada 1986; Rasaq 2022). They derived the education Gini Coefficient by modifying the conventional Gini Coefficient Model. While the conventional Gini Coefficient is given in Equation 1:
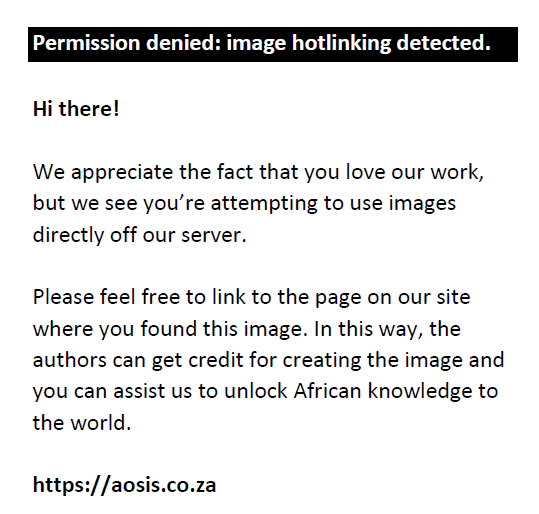
They modified the conventional formula to provide education-based Gini on the variables highlighted above:
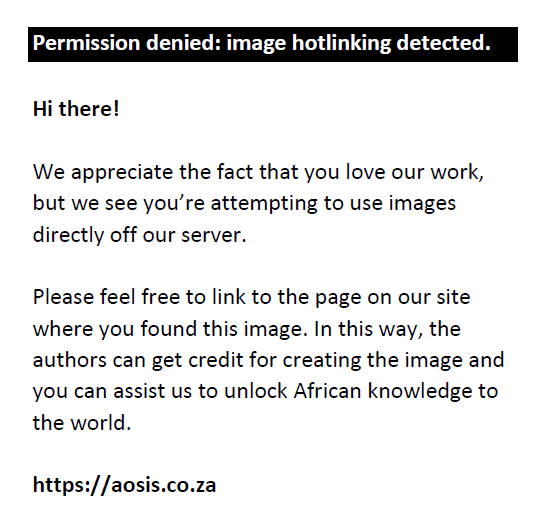
where EL represent the Education Gini Coefficient. The EL is calculated based on education attainment ratio; large population; ω is the mean year of schooling for certain age groups; si and sj are the share of population with certain degrees; yi and yj are the years of schooling for a different degree; and n is the number of educational categories (Thomas et al. 2002; eds. Zajda, Biraimah & Gaudelli 2008). Researchers like Checchi (2006); Almeida-Filho, Lim and Tang (2008); Morrison and Murtin (2010); Meschi and Scervini (2010); Castelló-Climent and Hidalgo-Cabrillana (2012); Foldvari & Van Leeuwen (2011), Wail, Hanchane and Kamal (2011); Jorda and Alonso (2015) also employed this method to create an Education Gini Index; and like the study by Rasaq (2022), we also rely on the Education Gini Index data as contained in the publication of Benaabdelaali, Hanchane and Kamal (2012) and Ziesemer (2016) for our analysis.
The trend of the education index in 32 African countries is presented in Figure 5 to show the prevalence of a high education index in many African countries based on the data of Benaabdelaali et al. (2012) and Ziesemer (2016). As observed in Figure 5, even though the trends maintained a relative downward slope, a higher education index was noticed in the 1990s and early 2000s in all represented countries. Also, it is observed that though the trend in most countries fluctuated significantly during the study period, the average education index in many African countries still stands above 0.5, indicating that the success recorded over the years is not enough to bridge the education gap in many of these countries (Rasaq 2022). Finally, it is also noted that low-income African countries are beset with a wider education gap, especially French-colonised countries in Africa like Niger, Mali and Central African Republic, among others.
 |
FIGURE 5: Education Gini for 32 African countries. |
|
Literature review
Theoretical underpinnings
The theoretical link between family income and education is conveyed in the Galor-Zeira model publication in 1988. The authors stress the importance of family wealth in the form of inheritance as a determining factor in school enrolment. Individuals are assumed to be identical in potential skills, differing only with respect to their inherited wealth, which dictates their school enrolment decisions (Galor & Zeira 1993). Consequently, individual inheritances determine their investment in education. The authors categorised dynasties with a smaller inheritance as poor, suffering from poverty, hunger, and malnutrition, which consequently restrict their access to education. Also, the prevalence of poverty and inequality in developing countries further creates intellectual inequality from country to country. On the aggregate, the distribution of wealth and economic status of each dynasty determine aggregate investment in education, the number of country-skilled and unskilled workers, and lastly, the economic output (Galor & Zeira 1993).
Furthermore, the effect of the wealth dynasties is not limited to only the short run, as education consequently determines the distribution of income and the concentration of wealth in the long run. The rich dynasties, with an inheritance, will continue investing in education, work as skilled labour, and leave a large bequest to their children, while the poor dynasties, with no inheritance, will be restricted by school costs, creating education inequality in the country. The education gap further creates an unequal society in which the rich can always afford education, while the poor live in poverty and are undernourished (Galor & Zeira 1993).
Another theory that incorporates the effect of income dynasties on education and economic growth is the unified theory of Oded Galor and Omer Moav (2004). The theory reconciled the conflicting viewpoints about the effect of income distribution on the developmental process in classical economics and the credit market imperfection model, via the analysis of investment in education. Considering the empirically important effect of education on economic growth, as revealed in the studies of Abramovitz and David (2000), as well as Goldin and Katz (2001) in the United States, the theory concludes that classicalists’ preference for physical capital in the development process, as against human capital via investment in quality education, advocated by credit market imperfections, reflects the various stages of industrialisation at different points in time, and in agreement with the above studies to recognise the importance of education in modern times (Galor & Moav 2004). Like the Galor-Zeira model, the structure of the unified model is also based on a bequest and two periods of schooling and working. The level of human capital in every society is determined by the outcome of individual education decisions during the first period. During this period individuals are identical in their education choices and inborn ability, though they may differ in family wealth. However, suppose individuals cannot borrow to finance education due to credit constraints, access to education will be limited by aggregate wealth transfer from parents and this creates education inequality between those who inherited wealth and the poor who are left with no bequest.
Empirical review
In the wake of the MDG, and the subsequent SDG, adopted between 2000 and 2015, an increase in research on malnutrition and education inequality has been observed. While several studies investigated the issue of malnutrition (Amugsi et al. 2019; Fotso 2007; Harttgen, Klasen & Vollmer 2012; Harttgen et al. 2013; Rashad & Sharaf 2018; Vollmer et al. 2014), relatively fewer studies focused on education inequality, particularly in Africa. Most authors studying malnutrition focused solely on economic growth (Harttgen et al. 2012, 2013; Vollmer et al., 2014) and poverty (Amugsi et al. 2019; Fotso 2007). In studies on education inequality, on the other hand, the issue of malnutrition has been largely overlooked (Buchmann 1999; Obasuyi & Rasiah 2019; Ombati & Ombati 2012).
To provide a concise empirical review, we limited our search to Africa-based studies utilising secondary data and focused on either education inequality or malnutrition. In one such study by Obasuyi and Rasiah (2019) the nexus between the wealth gap and education inequality across 25 African countries is investigated, also examined are gender disparities in education attainment. The authors employ the education attainment ratio to proxy education inequality and found overwhelming evidence of social exclusion in several African countries, recording significant disparities in secondary and tertiary enrolment, except in Zimbabwe.
Also, the study of Ombati and Ombati (2012) examined the issue of the gender-related gap in education based on the argument that in Africa, access to education is uneven for boys and girls, and biased through gender, regions, and location, resulting in a higher male literacy rate compared to girls and women. Analysing political, economic, cultural, institutional, and instructional factors, the paper concludes that poverty, child marriage, sexual harassment, and negative cultural values are, among other factors, highlighted in the paper as the leading contributors that create the gender gap in education.
Buchman (1999) examines the reality of education inequality and poverty, and explores the relationship between the two concepts in Africa after discussing the state of African poverty and the declining trend of school enrolment in many African countries. The author represents poverty using two different datasets, the poverty line index and the share of income household spent on consumption, the education index was proxy with school enrolment at primary, secondary, and tertiary levels. In the study it is found that stagnation in the quality of life and a continuous decline in educational enrolment in Africa are the outcomes of several factors, including Africa’s debt profile, declining developmental assistance from donor countries, fiscal mismanagement, corruption, and weak governance, among others (Buchman 1999).
In studies focused on malnutrition, Fotso (2007) used multilevel modelling to examine urban – rural differences in child malnutrition in Africa employing quantitative data from demographic and health surveys (DHS) of 15 sub-Saharan African countries. The study revealed a significant difference in urban – rural malnutrition in all countries considered, with the gap widening in some countries due to a fast decline in urban malnutrition. Amugsi et al. (2019) investigated the correlation of the double burden of malnutrition among women in Ghana, Nigeria, Kenya, Mozambique, and the Democratic Republic of Congo (DRC) using DHS data. In their analysis, they found a strong correlation between overweight, underweight, and education in the countries considered. Also, a positive association between living in better-off households where obesity was observed, while a negative association was observed between education and underweight.
Based on our review of empirical studies, we identified and addressed a minimum of three gaps in the literature on educational inequality. Firstly and foremost, we build on existing research, particularly the African studies conducted by Buchmann (1999), Ombati and Ombati (2012), and Obasuyi and Rasiah (2019), in which educational inequality, using secondary data, was considered. Secondly, while previous studies utilised enrolment (Buchmann 1999) and attainment data (Obasuyi & Rasiah 2019), studies focusing on the Gini Coefficients of Education have neglected Africa. As such, in our study we endeavour to bridge this gap. Lastly, prior investigations have primarily concentrated on the relationship between gender and education, such as those by Ombati and Ombati (2012) and Obasuyi and Rasiah (2019). The closest research to ours is the poverty – education attainment regression conducted by Buchmann (1999). However, this study was limited by the utilisation of incomplete educational inequality data as a proxy for educational inequality. Therefore, our investigation provides a more profound understanding of educational inequality in Africa and examined the correlation between malnutrition and educational inequality in the region.
Methodology
Drawing from the dynamics of the Galor-Zeira (1993) model framework and unified hypothesis, we specify the following augmented regression function which links malnutrition (MAL) and economic growth (GDP) to educational inequality (E/I):

where it is expected that MAL and GDP are supposed to widen and reduce E/I, respectively. The empirical analysis is carried out by employing the three-step procedure described in Aguiar-Conraria and Soares (2014) and Mihanovic, Orlić & Pasarić (2009) to investigate the relationship between malnutrition (x1), economic growth (x2), and the Education Gini (y) in a time – frequency space using continuous complex wavelet tools (Figure 6). Firstly, we convolute the individual time series with a set of complex-valued ‘daughter wavelets’ which are generated by a common ‘mother’ wavelet. The convolution process generates the wavelet coefficients that are responsible for the amplitude and phased dynamics in time–frequency space. The daughter wavelets for each series are defined as:
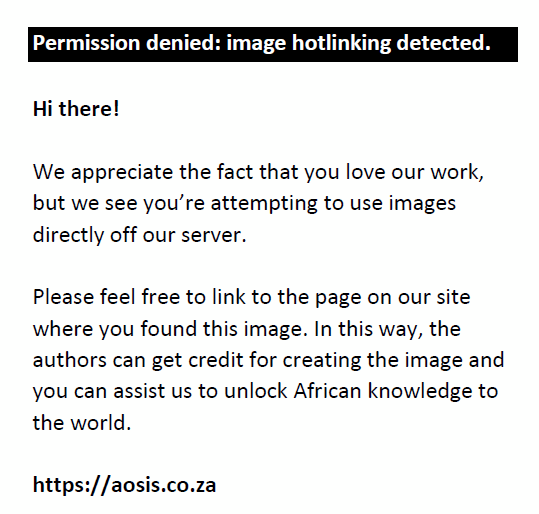


where * is the conjugate of the complex number, τ and s are the translation and dilation parameters responsible for amplitude and phase dynamics in time– frequency space; while ψ is the mother Morlet wavelet defined:
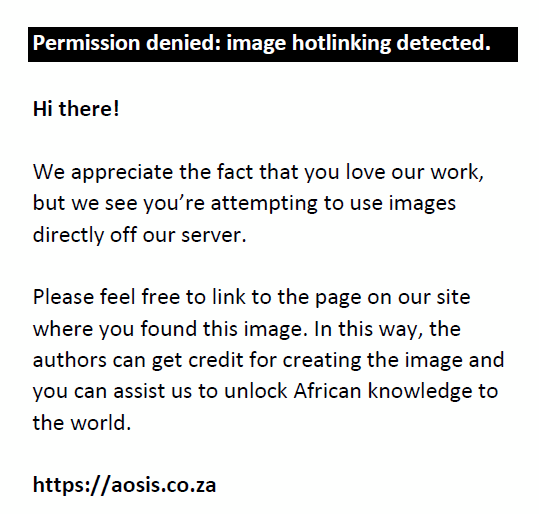
where ω0 is set at 2π to ensure optimal joint time–frequency resolution compared to other types of wavelet functions, such as the Mexican Hat, Paul, and Gabor wavelets. In other words, the Morlet wavelets are most efficient in dealing with the Heisenberg uncertainty principle which insinuates a trade-off between time and frequency, i.e. when one is more precise, the other becomes less precise (Grinsted, Moore & Jevrejeva 2004; Mihanovic, Orlić & Pasarić, 2009; Torrence & Webster 1999). Secondly, we extract the wavelet power spectrum (WPS) of the y(t) and (x(t) series (i.e. Wxx = |Wx|2 and Wyy = |Wy|2), as well as their Cross-Wavelet Power Spectrum (CWPS) (WPS)xy = Wxy = |Wxy|, from which the wavelet coherence, is computed:

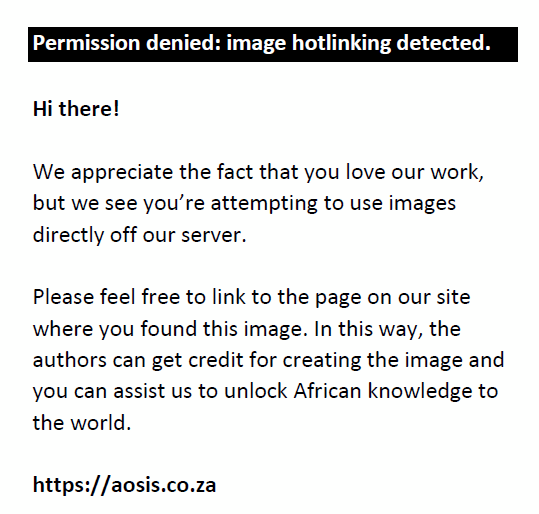

where S is a smoothing operator in both time and scale. The phase-difference dynamics are determined:

where π < ϕx,y < -π and provides information on (1) whether the pair of series are in-phase (positive) or anti-phase (negative) synchronised and (2) whether x leads y or vice-versa. Thirdly, we extend the wavelet coherence framework to control for other variables, hence transforming the bivariate time–frequency analysis into a multivariate case. To this end, we compute the following partial wavelet coherence coefficients:
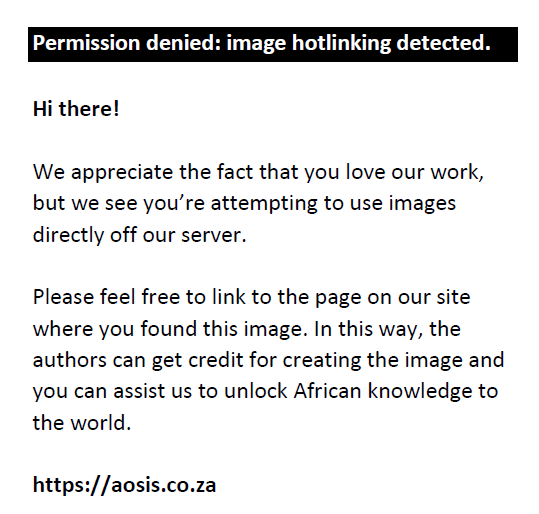
Data and results
Data description and summary statistics
Twenty-eight African countries’ statistical data were employed for our study. The three-time-series data used to investigate the relationship between malnutrition and education inequality in Africa are: the Gini Coefficient for Education, as computed by Benaabdelaali et al. (2012) and Ziesemer (2016), is employed to proxy education inequality; malnutrition data were obtained from UNICEF, WHO and the World Bank Group and Gross Domestic Product Growth Rate, retrieved from World Bank Development Index, were used to proxy economic growth. Table 1 presents the feel of the three-time-series dataset used for the study. Table 1 shows the mean and standard deviation for each of the 28 African countries involved. The countries are further grouped based on the regions. On average the Gini Coefficient for Education is high across African countries. Morocco and Egypt in the northern region have the highest Gini Coefficient for Education; Cameroon and Eswatini have the highest in the central and southern region; Gambia, Mali, and Liberia have the highest among the West African countries, while Rwanda and Mozambique have the highest in East African region. As for malnutrition, Burundi has the highest in Africa while Egypt, Zambia, and Liberia have the highest at their respective regional levels. For Gross Domestic Product Growth Rate (GDPG), Zimbabwe has the highest with a value of 19.67532 while Morocco, Mauritania, and Rwanda have the highest at the regional level.
Interpreting wavelet heat maps
This section explores the wavelet coherence results for all 28 African countries considered in this study, using the ‘biwavelet’ package in R. The section is divided into two parts. The first describes the dynamic correlation between education inequality and malnutrition across a time–frequency domain with all the countries involved. In the second part the findings are discussed of partial wavelet co-movement between education inequality and malnutrition after removing the effect of GDPG. The two results are presented in ‘heat maps’ in Online Appendix 1 and Online Appendix 2, respectively. For the wavelet graph, the time dimension is measured on the horizontal axis; frequency is measured on the vertical axis; colour contours in the heat maps measure the intensity of the co-movement between malnutrition and education inequality, with cooler colours indicating weaker correlation between the series, while the warmer colours represent strong co-movement between the series. The 5% significance level is represented by the black round lines surrounding the colour contours, while the inverted cone shape (solid black line) is the ‘cone of influence’ which accounts for edge effects. Lastly, the phase-dynamics are represented by the arrow notation, which shows the ‘sign’ on the relationship (negative or positive) and the direction of causality. This is better understood with the help of the diagram in Figure 5 and the outcomes are summarised as follows:
- A positive or in-phase relationship, with educational inequality leading (lagging), malnutrition/economic growth is denoted by the arrows pointing ↑, ↗, → (↘)
- A negative or anti-phase relationship, with educational inequality leading (lagging), malnutrition/economic growth is denoted by the arrows pointing ↓, ↙, ← (↖).
Wavelet coherence results
Online Appendix 1 presents the wavelet coherence results between education inequality and malnutrition across the 28 African countries studied. The result clearly shows that malnutrition caused education inequality in Africa from 1998 to 2010. The situation is similar across all African countries as most arrows are in-phase with warmer colours signifying the existence of a strong positive correlation between malnutrition and educational inequality in Africa during the highlighted periods. The wavelet result, presented in Online Appendix 1 grouped countries by region, and it is interesting to point out that regardless of the region, the situation was the same during the highlighted period which denotes the period before and early period of pursuing the development goals (MDG). The results show a higher cyclical frequency (strong positive correlation) between malnutrition and educational inequality in most countries noticeable during the period of 13 years (1998–2010). This is indicated by red contours with arrows in-phase pointing to the right direction indicating that malnutrition leads to educational inequality.
However, during the latter years of pursuing the MDG and the beginning of SDG i.e., from 2010–2020, a lower frequency is observed in most countries, reflecting the impact of MDG 1, and 2 SDG 1 and 4, among other initiatives, targeted to eradicate hunger and increase basic education accessibility. Although hunger has not been completely eradicated in Africa and traces of correlation between these two variables (MAL and E/I) are still found in countries like Egypt, Rwanda, Kenya, Ghana, Eswatini, Mauritius, Namibia, and Cameroon, statistical figures for chronic poverty and stunting have improved over the years as revealed via the statistical publications of World Bank, UNICEF, UNFAO, (Bongaarts 2021; UNICEF 2021; WDI 2022) as contained in the introductory section of this study. Also, Africa’s primary enrolment rate increased since the 1990s (WDI 2022). Therefore, on average, judging by the direction of the arrows, we could conclude that the synchronisation between the two series is in-phase with malnutrition leading educational inequality from 1998–2010. However, the narrative has changed due to several initiatives directed at improving education, especially at the basic level, and eradicating hunger in Africa.
TABLE 2: Summary of wavelet coherence results. |
Partial wavelet coherence results
Online Appendix 2 shows the result of partial wavelet coherence between educational inequality and malnutrition (EDU GINI, MAL, and GDPG), after eliminating the effect of the growth rate in the Gross Domestic Product. Online Appendix 2, when compared with Online Appendix 1, that contained the wavelet coherence analysis between educational inequality and malnutrition, showing that there is barely a change in the wavelet plots between educational inequality and malnutrition in most countries before and after removing the effect of GDPG. A little change is, however, observed in countries like Algeria, Cameroon, Ghana, Lesotho, Kenya, Mozambique, South Africa, Senegal, Tanzania, Uganda, and Zambia, while a significant change in the high power is observed only in Egypt. This clearly indicates that the previous increased output in African countries is not adequate to impact significantly on educational inequality.
Conclusion
The study is sought to examine the correlation between educational inequality and malnutrition in twenty-eight African countries holding the influence of growth constant prior to and during the pursuit of the MDG and SDG; specifically, the periods between 1998 and 2020, using the wavelet coherence method which illustrates the synchronisation the series involve in time–frequency space.
The wavelet coherence analysis findings revealed a strong positive correlation between educational inequality and malnutrition in all the countries involved, prior to and during the early years of MDG. The results show a positive relationship between the variable from 1998–2010 with the prevalence of malnutrition causing educational inequality across Africa. However, towards the latter years of MDG and in the early years of SDG, specifically from 2010, the relationship appeared to change in most countries, observed by the absence of wavelet coherence arrow direction from 2011–2020 as shown in Online Appendix 1. Hence, this could be a result of MDG 1 and 2, and SDG 2 and 4, among other efforts to eradicate hunger and make education more accessible to all. Some programmes adopted to reduce malnutrition among school children, such as school feeding programmes, as well as educational equality policies may be helping in bridging the educational gap in Africa.
Furthermore, subsequent analysis using partial wavelet coherence analysis after controlling the influence of previous output growth showed that the effect of GDP growth rate on educational inequality and malnutrition is just artificial in 16 out of 28 countries considered, as the results show that there is barely a change in the wavelet plots between educational inequality and malnutrition in these countries before and after removing the effect of GDPG. In countries like Algeria, Cameroon, Ghana, Lesotho, Kenya, Mozambique, South Africa, Senegal, Tanzania, Uganda, and Zambia, the growth does have little impact as revealed by the partial wavelet coherence plot, while only Egypt’s case is different from the rest of the countries. We therefore conclude that while high malnutrition in Africa was partly responsible for the wide educational gap on the continent during the 1990s and early 2000s, policy effectiveness may have changed this relationship.
Acknowledgements
This article is partially based on R.R., the first author’s thesis entitled ‘Being a thesis submitted in fulfilment of the requirements’, towards the degree of Doctor of Philosophy (Economics) in the Department of Economics, Nelson Mandela University, South Africa, on December 2022, with supervisor Prof. Andrew Phiri. It is available here: http://vital.seals.ac.za:8080/vital/access/manager/PdfViewer/vital:62483/SOURCE1?viewPdfInternal=1.
Competing interests
The authors declare that they have no financial or personal relationship(s) that may have inappropriately influenced them in writing this article.
Authors’ contributions
R.R. was responsible for the conceptualisation, methodology, software, formal analysis, investigation, resources, data curation, the writing of the original draft and visualisation of this article. A.P. was responsible for the conceptualisation, validation, investigation, resources, data curation, writing of the article, reviewing and editing, visualisation, supervision, and project administration.
Ethical considerations
Ethical clearance approval was received from the Faculty Ethics Committee of the Faculty of Business and Economic Science of Nelson Mandela University on 24 January 2022 with Ethical clearance number H22-BES-ECO-001.
Funding information
This research received no specific grant from any funding agency in the public, commercial, or not-for-profit sectors.
Data availability
The data that support the findings of this study are available from the corresponding author, R.R, on reasonable request.
Disclaimer
The views and opinions expressed in this article are those of the authors and do not necessarily reflect the official policy or position of any affiliated agency of the authors.
References
Almeida-Filho, N., Lessa, I., Magalhães, L., Araújo, M.J., Aquino, E., James, S.A. et al., 2004, ‘Social inequality and depressive disorders in Bahia, Brazil: Interactions of gender, ethnicity, and social class’, Social Science & Medicine 59(7), 1339–1353. https://doi.org/10.1016/j.socscimed.2003.11.037
Abramovitz, M. & David, P., 2001, Two centuries of American macroeconomic growth, Stanford Institute for Economic Policy Research Policy Paper.
Aguiar-Conraria, L., & Soares, M.J., 2014, ‘The continuous wavelet transform: Moving beyond uni- and bivariate analysis’, Journal of Economic Surveys 28(2), 344–375. https://doi.org/10.1016/j.physa.2008.01.063
Amugsi, D.A., Dimbuene, Z.T. & Kyobutungi, C., 2019, ‘Correlates of the double burden of malnutrition among women: An analysis of cross sectional survey data from sub-Saharan Africa’, BMJ Open 9(7), 3–5. https://doi.org/10.1136/bmjopen-2019-029545
Bain, L.E., Awah, P.K., Geraldine, N., Kindong, N.P., Siga, Y., Bernard, N. et al., 2013, ‘Malnutrition in Sub-Saharan Africa: Burden, causes and prospects’, Pan African Medical Journal 15(1), 1–3. https://doi.org/10.11604/pamj.2013.15.120.2535
Barro, R.J. & Lee, J.W., 1993, ‘Losers and winners in economic growth’, The World Bank Economic Review 7 (suppl. 1), 267–298. https://doi.org/10.1093/wber/7.suppl_1.267
Barro, R.J. & Lee, J.W., 1996, ‘International measures of schooling years and schooling quality’, The American Economic Review 86(2), 218–223.
Benaabdelaali, W., Hanchane, S. & Kamal, A., 2012, ‘Educational inequality in the world, 1950–2010: Estimates from a new dataset’, in Inequality, mobility and segregation: Essays in honor of Jacques Silber, vol. 20, pp. 337–366, Emerald Group Publishing Limited.
Bongaarts, J., 2021, ‘FAO, IFAD, UNICEF, WFP and WHO The state of food security and nutrition in the world 2020. Transforming food systems for affordable healthy diets FAO, FAO, Rome.
Buchmann, C., 1999, ‘Poverty and educational inequality in sub-Saharan Africa’, Prospects 29(4), 503–515. https://doi.org/10.1007/BF02736901
Castelló-Climent, A. & Hidalgo-Cabrillana, A., 2012, ‘The role of educational quality and quantity in the process of economic development’, Economics of Education Review 31(4), 391–409. https://doi.org/10.1016/j.econedurev.2011.11.004
Chabite, I.T., Garrine, C., Ferrão, L.J. & Fernandes, T.H., 2018, ‘Malnutrition and school feeding programmes’, Journal of Nutritional Health & Food Engineering 8(5), 340–344.
Checchi, D., 2006, The economics of education: Human capital, family background and inequality, Cambridge University Press.
Fotso, J.C., 2007, ‘Urban–rural differentials in child malnutrition: Trends and socioeconomic correlates in sub-Saharan Africa’, Health & Place 13(1), 205–223. https://doi.org/10.1016/j.healthplace.2006.01.004
Galor, O. & Moav, O., 2004, ‘From physical to human capital accumulation: Inequality and the process of development’, The Review of Economic Studies 71(4), 1001–1026. https://doi.org/10.1111/0034-6527.00312
Galor, O. & Zeira, J., 1993, ‘Income distribution and macroeconomics’, The Review of Economic Studies 60(1), 35–52. https://doi.org/10.2307/2297811
Gelli, A., Meir, U. & Espejo, F., 2007, ‘Does provision of food in school increase girls’ enrolment? Evidence from schools in sub-Saharan Africa’, Food and Nutrition Bulletin 28(2), 149–155. https://doi.org/10.1177/156482650702800203
Goldin, C. & Katz, L.F., 2001, ‘The legacy of US educational leadership: Notes on distribution and economic growth in the 20th century’, American Economic Review 91(2), 18–23.
Grinsted, A., Moore, J.C. & Jevrejeva, S., 2004, ‘Application of the cross wavelet transform and wavelet coherence to geophysical time series’, Nonlinear Processes in Geophysics, 11(5/6), 561–566.
Harttgen, K., Klasen, S. & Vollmer, S., 2012, Economic growth and child undernutrition in Africa (No. 14), Global Food Discussion Papers, No. 14, GeorgAugust-Universität Göttingen, Research Training Group (RTG) 1666 – GlobalFood, Göttingen.
Harttgen, K., Klasen, S. & Vollmer, S., 2013, ‘Economic growth and child undernutrition in sub-Saharan Africa’, Population and Development Review 39(3), 397–412. https://doi.org/10.1111/j.1728-4457.2013.00609.x
Jorda, V. & Alonso, J.M., 2015, Measuring educational attainment as a continuous variable: A new database (1970–2010). https://arxiv.org/abs/1508.01351
Lim, A.S.K. & Tang, K.K., 2008, ‘Human capital inequality and the Kuznets curve’, The Developing Economies 46(1), 26–51. https://doi.org/10.1111/j.1746-1049.2007.00054.x
Meschi, E. & Scervini, F., 2010, GINI DP 3: New dataset of educational inequality (No. 3), AIAS, Amsterdam Institute for Advanced Labour Studies.
Mihanović, H., Orlić, M. & Pasarić, Z., 2009, ‘Diurnal thermocline oscillations driven by tidal flow around an island in the Middle Adriatic’, Journal of Marine Systems 78, S157–S168. https://doi.org/10.1016/j.jmarsys.2009.01.021
Morrison, C. & Murtin, F., 2010, The Kuznets curve of education: A global perspective on education inequalities, CEE DP 116, Centre for the Economics of Education (NJ1).
Musau, Z., 2018, ‘Africa grapples with huge disparities in education’, Africa Renewal 31(3), 10–11. https://doi.org/10.18356/1a71d0ef-en
Nabassaga, T., Chuku, C., Mukasa, A. & Amusa, H., 2020, How does educational inequality affect income inequality in Africa?, Working Paper Series 2469, African Development Bank Abidjan, Côte d’Ivoire.
Nehru, V., Swanson, E. & Dubey, A., 1995, ‘A new database on human capital stock in developing and industrial countries: Sources, methodology, and results’, Journal of Development Economics 46(2), 379–401. https://doi.org/10.1016/0304-3878(94)00054-G
Obasuyi, F.O.T. & Rasiah, R., 2019, ‘Addressing education inequality in sub-Saharan Africa’, African Journal of Science, Technology, Innovation and Development 11(5), 629–641. https://doi.org/10.1080/20421338.2019.1567655
Ombati, V. & Ombati, M., 2012, ‘Gender inequality in education in sub-Saharan Africa’, Journal of Women’s Entrepreneurship and Education (3-4), 114–136.
Psacharopoulos, G. & Arriagada, A.M., 1986, ‘The educational composition of the labour force: An international comparison’, International Labour Review 125, 561.
Rasaq, R., 2022, ‘Different dimensions of inequality and sustainable economic growth in Africa’, PhD thesis, Dept. of Economics, Nelson Mandela University.
Rashad, A.S. & Sharaf, M.F., 2018, ‘Economic growth and child malnutrition in Egypt: New evidence from national demographic and health survey’, Social Indicators Research 135, 769–795. https://doi.org/10.1007/s11205-016-1515-y
Setboonsarng, S., 2005, Child malnutrition as a poverty indicator: an evaluation in the context of different development interventions in Indonesia (No. 21), ADBI Discussion Paper.
Thomas, V., Wang, Y. & Fan, X., 2002, A new dataset on inequality in education: Gini and Theil indices of schooling for 140 countries 1960–2000, World Bank Working Paper, World Bank, Washington, DC, viewed 25 Feb. 2021, from www33.brinkster.com/yanwang2/EducGinirevised10-25-02.pdf.
Torrence, C. & Webster, P.J., 1999, ‘Interdecadal changes in the ENSO-monsoon system’, Journal of Climate 12(8), 2679–2690. https://doi.org/10.1175/1520-0442(1999)012<2679:ICITEM>2.0.CO;2
Siddiqui, F., Salam, R.A., Lassi, Z.S. & Das, J.K., 2020, ‘The intertwined relationship between malnutrition and poverty’, Frontiers in Public Health 8, 453. https://doi.org/10.3389/fpubh.2020.00453
UNDP, 2018, Human development indices and indicator 2018 statistical update, pp. 26–29, UNDP, New York.
United Nations Educational, Scientific and Cultural Organization (UNESCO), 2022, New estimation confirms out-of-school population is growing in sub-Saharan Africa, pp. 1–10. Factsheet, 62 (policy paper 48).
UNICEF, 2020, What have we learnt?: Overview of findings from a survey of ministries of education on national responses to COVID-19, UNICEF Publications, New York.
UNICEF, 2021, UNICEF global databases – Infant and young child feeding, UNICEF, New York, NY.
United Nations Children’s Fund, 2023, Undernourished and Overlooked: A Global Nutrition Crisis in Adolescent Girls and Women, UNICEF Child Nutrition Report Series, 2022, UNICEF, New York, NY.
UNICEF, 2023, Stunting has declined steadily since 2000 – But faster progress is needed to reach the 2030 target, UNICEF, New York, NY.
Union, A., 2022, Born to learn: Spotlight on basic education completion and foundational learning in Africa, vol. 2022, UNESCO Publishing, Paris.
United Nations Research Institute for Social Development, 2005, Gender equality: Striving for justice in an unequal world, United Nations Research Institute for Social Development, Geneva.
Vollmer, S., Harttgen, K., Subramanyam, M.A., Finlay, J., Klasen, S. & Subramanian, S.V., 2014, ‘Association between economic growth and early childhood undernutrition: Evidence from 121 demographic and health surveys from 36 low-income and middle-income countries’, The Lancet Global Health 2(4), e225–e234. https://doi.org/10.1016/S2214-109X(14)70025-7
Wail, B., Hanchane, S. & Kamal, A., 2011, A new data set of educational inequality in the world, 1950–2010: Gini index of education by age group, pp. 1950–2010, SSRN, viewed n.d., from https://papers.ssrn.com/sol3/papers.cfm?abstract_id=1895496#paper-references-widget.
WDI, 2022, World Development Indicators, World Bank, Washington, DC.
World Bank, 2014, World Development Indicators 2015, World Bank, Washington, DC.
Wineman, A., Ekwueme, M.C., Bigayimpunzi, L., Martin-Daihirou, A., De Gois V.N., Rodrigues, E.L. et al., 2022, ‘School meal programs in Africa: Regional results from the 2019 global survey of school meal programs’, Frontiers in Public Health 10, 871866. https://doi.org/10.3389/fpubh.2022.871866
World Inequality Report (WIR), 2022, World Inequality Report 2022, Harvard University Press, viewed n.d., from https://wir2022.wid.world/www-site/uploads/2021/12/WorldInequalityReport2022_Full_Report.pdf.
World Bank, 2017, World development report 2018: Learning to realize education’s promise, World Bank, Washington, DC.
World Bank, 2020, Monitoring global poverty, World Bank Group Bank, Washington, DC.
World Bank, 2021a, Learners with disabilities and COVID-19 school closures: Findings from a global survey conducted by the World Bank’s inclusive education initiative, World Bank, Washington, DC, viewed 17 April 2023, from http://hdl.handle.net/10986/36326.
World Bank, 2021b, World development report 2021: Data for better lives, World Bank, Washington, DC.
World Food Programme (WFP), 2000, Food and nutrition handbook, Annex 3.2, World Food Programme, Rome.
World Health Organization, 2020, The state of food security and nutrition in the world 2020: transforming food systems for affordable healthy diets, vol. 2020, FAO, Rome.
World Health Organization, 2014, Global nutrition targets 2025: Low birth weight policy brief (No. WHO/NMH/NHD/14.5), World Health Organization, Geneva.
UNICEF and WHO, 2020, Levels and trends in child malnutrition: Key findings of the 2019 Edition of the Joint Child Malnutrition Estimates, World Health Organization, Geneva.
Zajda, J., Biraimah, K. & Gaudelli, W. (eds.), 2008, Education and social inequality in the global culture, vol. 1, Springer Science & Business Media, New York, NY.
Ziesemer, T., 2016, ‘Gini coefficients of education for 146 countries, 1950–2010’, Bulletin of Applied Economics 3(2), 1–8.
|