Abstract
Background: Gains in improving bank efficiency have widely been regarded as one of the most effective means of ensuring sustainability in a financial system.
Aim: It is proposed in this article to measure monetary policy impulses and the dynamic panel data model to examine the linear relationship.
Setting: The nexus between financial structure and monetary policy impulses is analysed, considering 21 sub-Saharan African (SSA) nations during the time period from 2011 to 2022.
Method: The Vector Autoregressive (VAR) analysis is employed to measure monetary policy impulses and the dynamic panel data model to examine the linear relationship.
Results: A significant positive association between competition in the banking system and monetary policy impulses was found. Monetary policy impulses are inversely and significantly related to financial development. Also, the size of the central bank has a significant positive influence on the effectiveness of monetary policy.
Conclusion: The financial structure has a significant role to play in the monetary transmission mechanism and credit markets help propagate and amplify shocks to the macro-economy.
Contribution: The relationship between financial structure and monetary policy is not a one-way street as these variables tend to influence one another. Empirical literature on the subject is not very recent and come to differing conclusions, hence the rationale for conducting this article in SSA, employing both the structural VAR model and the dynamic panel data model.
Keywords: monetary policy impulses; financial structure; sub-Saharan Africa; banking system competition; financial development; central bank size.
Introduction
Contemporary perspectives on the monetary transmission mechanism assign a vital role to the development of the financial sector and the set-up of the financial structure when analysing monetary policy effectiveness on output and prices. Financial structure is defined by Cotarelli and Kourelis (1994) as the existence and evolution of financial markets, the extent of market concentration, and the existence of foreign financial sources. The primal goal of monetary policy is maintaining price stability, although many nations’ central banks may also have other goals in mind, such as ensuring long-term growth and recovery, a favourable balance of payments, and higher standards of living. Central banks intervene in financial markets to influence the real economy in order to attain these goals. In this way, financial markets act as the connecting link in the monetary policy-to-real economy transmission mechanism (Hildebrand 2006). Monetary policy transmission is in essence a financial procedure in which the financial sector acts as the connecting link through which monetary policy impulses affect the real economy (Effiong, Esu & Chuku 2017).
There is no doubt that when implementing the monetary policy framework, monetary authorities pay close attention to commercial banks and other financial intermediaries (Bean, Larsen & Nikolov 2002). This does not, however, mean that central banks perceive financial intermediary behaviour as a critical component of monetary policy, as they may have goals other than price stability. Banks are not neutral ‘conveyers’ of monetary policy impulses, according to economic literature (Bernanke 1993; Bernanke & Gertler 1989). The unresponsiveness of lending rates in relation to the money market rates is frequently viewed as a barrier to the even diffusion of monetary policy impulses (Cotarelli & Kourelis 1994; ed. Ncube 2008). In addition, in as much as monetary policy influences the operational work of financial markets, financial market prices replicate market expectations about future economic developments such as output, inflation, and the path of monetary policy (Bernanke 1993; Hildebrand 2006).
There is quite a vast number of countries in sub-Saharan Africa (SSA), and while their banking and institutional structures tend to be systematically different from those in emerging markets and advanced economies, they are also heterogeneous among themselves. The need to explore the relationship between financial structure and monetary policy in SSA is necessitated by the existence of divergent results from previous studies. According to Mishra and Montiel (2012), the financial structure of an economy, as well as some features of its nonmonetary regimes, influence the channels of monetary transmission. While economic theory has documented this link, most practical research has focused on the effect of operational changes on financial market on money demand (for example Corker & Tseng 1991). Some previous empirical studies have found that monetary policy effectiveness hinges on competitive, as well as well-functioning financial systems (Effiong et al. 2017; Mishra & Montiel 2012; ed. Ncube 2008). However, the nature of the relationship between financial structure and monetary policy impulses in SSA, is not yet well established and understood. Accordingly, this issue is taken up in this article by employing both the structural Vector Autoregressive (VAR) model and dynamic panel data model in analysing the relationship between the financial structure and monetary policy impulses in SSA.
Background
The late 1980s and early 1990s saw quite a substantial number of SSA countries moving towards the removal of administrative control of the rate of interest and credit, and a shift towards indirect monetary policy (ed. Ncube 2008). However, due to the illiquidity of the financial markets, including secondary bill markets, various governments could not afford to use tools such as open market operations (OMOs), an important element in indirect monetary management, until the late 2000s. Over the past two decades, most SSA nations have made progress in terms of financial development. Financial institutions and financial markets are, nonetheless, underdeveloped in comparison to other developing regions, with the exception of the region’s middle-income countries like South Africa (Mlachila et al. 2016).
When considering private credit provision, as well as financial intermediation, the news is even worse in SSA. In the pre-crisis period of 2007, the average domestic credit to the private sector ratio for commercial banks (as a percent of Gross Domestic Product [GDP]) was only 32.21% in SSA, but the same ratio ranged from 45% to 60% in other developing countries (World Bank 2021). The domestic credit ratio to the private sector by banks during the decade from 2010 to 2019 has been below the pre-2010 levels indicating a declining role of the domestic financial sector in contributing to national income. The commercial banks’ population in every 100 000 adults is still below five in SSA although the figures are gradually rising. Bank liquid reserves to bank assets ratio has been following a steady growth from 2002 to 2019, with figures ranging between 16% and 20%. This gives a clear indication that the region is still underdeveloped in terms of finance.
However, these reported financial development indices fail to highlight crucial new advances in the SSA’s financial systems. Recent changes in the economic and regulatory contexts have been linked to improvements (Allen et al. 2016). There are currently major factors at work to enhance SSA’s chances of integrating into the global financial industry.
For example, global capital markets, including those in developing market economies, are becoming more integrated, resulting in more capital mobility. Barriers to international capital movements have been greatly removed in SSA and the region has experienced the rapid adoption of new financial products, such as cryptocurrency. In addition, information technology (IT) is rapidly linking Africa to the world economy, allowing outside and foreign investors seeking the benefits of international diversification to efficiently access African financial systems (Otchere, Senbet & Simbanegavi 2017). Despite this outstanding achievement in terms of financial sector reforms and economic growth, according to Green (2013), financial systems in SSA are much more underdeveloped than in most other markets around the globe on practically all financial development indicators.
In Africa there are significant development and financial inclusion gaps in both banking and non-banking systems (Allen et al. 2016). In the latter, for example, most African stock markets are quite thin, with low levels of liquidity provision, based on standard metrics of trading activity and capitalisation (Allen, Otchere & Senbet 2011; Senbet & Otchere 2010). African stock markets’ performance was quite remarkable during the pre-crisis period of 2008 in terms of risk-adjusted and absolute returns. Significant problems in terms of liquidity and depth still exist in African stock markets, but increases in capitalisation and trading activity have been realised. Sub-Saharan Africa’s stock markets, with the exception of South Africa and Nigeria, are still small in terms of market capitalisation and the number of listed companies. The data on listed domestic companies and market capitalisation, in relation to GDP from 2009 to 2022, are presented in Table 1. The Southern African region has relatively higher market capitalisation as a percentage of GDP driven mainly by the South African markets. Table 1 also shows that the mean market capitalisation for SSA has been increasing steadily from 2009 to 2019.
TABLE 1: Depth of sub-Saharan Africa stock markets. |
According to Green (2013), the SSA experience itself is widely diverse, having South Africa, and to a certain extent, Kenya and Nigeria realising increased maturity in financial market deepening, whereas the rest of the countries have small, fragmented, and relatively underdeveloped financial markets. Even among the more prosperous countries, the pathways taken, appear to be widely varied. In Kenya for example, financial broadening appears to be at the centre of financial sector development, while the South African case is deeply rooted in financial deepening. For all rising and pre-emerging economies, including those in SSA, the global financial crisis and previous crises have created additional concerns. Traditional banking and informal finance dominate the financial systems of SSA, with South Africa doing exceptionally well in terms of finance.
However, as a result of substantial economic reforms, carried out over the past three decades, there have been significant changes in the banking environment with a rise in non-bank financing, notably in the establishment of stock markets that have spread through Africa. These achievements are suspected to be in response to the progress made in reforming the regulatory and economic environments that the entire region has realised in recent years.
Central banks and deposit-taking institutions make up the SSA banking system. Although central banks are technically independent of government control, they collaborate mostly with their individual Ministries of Finance to assist in the establishment and implementation of government policies (Allen et al. 2011). In most SSA countries, state-owned banks or a few major, sometimes global, institutions lead the banking system. However, as part of the African countries’ restructuring agenda, the banking sector in Africa is undertaking reforms centred on transforming state-owned banks into privately owned ones so as to increase the quality of the banks. The banks in Nigeria and the Southern African Development Community’s (SADC) countries of Botswana, Malawi, Seychelles, and South Africa are well-capitalised and dynamic, and they pursue banking practices in a highly innovative way. The Seychelles’ banking sector is extremely modernised and somewhat similar to those in most advanced economies.
Western and Eastern African countries, such as Cameroon, are part of monetary union, as well as members of the Southern Africa Currency Union (SACU), such as South Africa and Namibia. Member countries in most of the monetary arrangements in Africa, such as SACU, and the Franc Zones, have almost similar financial systems. Except for Botswana, all SACU member states form the common monetary area, which allows the South African Rand to be fully convertible in these countries. This implies that South Africa’s exchange rate and monetary policy is conveyed across SACU. The financial sectors in these countries and the local legal systems follow Roman-Dutch and Common Law backgrounds. Countries in West Africa such as Cameroon, Chad, and Equatorial Guinea are affiliated with one or two monetary unions. The form of monetary integration within these regional groupings presents opportunities for deeper monetary integration in Africa with the operation of the African Continental Free Trade Area (AfCFTA).
Until recently, Africa has realised unhindered expansion and has been one of the world’s fastest-growing areas in many years (Rotberg 2013). Sub-Saharan Africa has long been the world’s most economically underdeveloped region, but its economies appear to be growing, though growth was subdued in 2019 and 2020 due to the global coronavirus pandemic. Figure 1 indicates that the 2019 GDP growth rate in SSA was 2.28% (down from 2.41% in 2018), while the average regional growth rate during the period from 2009 to 2019 was 3.47%. The inflation rate averaged 4.43% from 2009 to 2019 and depicts a relatively downward trend from 1976 to 2019. The inflation rate was at an all-time high of 27.45% in 1994 and has recorded figures below 10% from 1996 to 2019, except in 2008 when 10.46% was recorded. However, the outlook is quite different at the country level with places like Zimbabwe recording inflation rates as high as 231 million percent in 2008. Broad money grew steadily in SSA from 1984 to 2019, while total reserves, as a percentage of total external debt, has been subject to wide fluctuations during the period between 1971 and 1982, and also 2003 and 2017. Figure 1 shows the trend in broad money, GDP growth, total reserves as a percentage of total external debt, and the inflation rate for SSA from 1970 to 2022.
 |
FIGURE 1: Trend in broad money, Gross Domestic Product growth, total reserves, and inflation in sub-Saharan Africa (1970–2022). |
|
Furthermore, monetary policy plays a significant role in the majority of SSA countries, although in most instances, in a submissive way to fiscal policy in influencing aggregate demand. While the primary goal is maintaining price stability, monetary authorities do work closely with the fiscal authorities, and in some countries, they are not entirely independent of the central government’s control, except for a few countries like South Africa and Mauritius. The control of money supply rests upon the monetary authorities and credit expansion has a more far- reaching impact on aggregate demand and inflation in developing countries. However, expansive monetary policy involving an increase in money supply has to be well-targeted to guard against fierce inflationary pressures and rapid exchange-rate depreciation. Well-functioning financial markets with links to the international settlement system have evolved over the past decade in SSA and so has financial sector regulation. Mobile money services in countries like Kenya have replaced conventional financial transaction channels, while financial deregulation has been fundamental in enabling new developments that have raised the standards of living of the populace and significantly reduced transaction costs.
Statement of the problem
The financial system in SSA is thin and its role in the growth of the economy is still minimal (Adelegan & Radzewicz-Bak 2009; Effiong et al. 2017; Mlachila et al. 2016). The banking sector in developing economies is generally small, the banking competition level is low combined with feeble financial intermediation, and the legal setup for financial activities is constantly moving in these economies. Additionally, a well-developed financial system could lessen the effectiveness of monetary policy as new innovations may be introduced to hedge against unanticipated monetary shocks. Some common features combine to render SSA countries’ financial systems highly susceptible to internal and external shocks.
Maintaining a steady price level and stable growth is key to long-term prosperity and when these variables become too volatile, there are unforeseen consequences for and effects on the economy that keep financial markets from functioning at their optimal level of efficiency. Financial markets are characterised by information asymmetry and the Central Bank often has instruments to achieve the set objectives (Gertler & Gilchrist 1994). The instruments do not directly affect the goals but the variables, such as reserves and loans, which in turn affect goal variables such as output and inflation with a lag. Policymakers face difficulties in predicting the precise effect of monetary policy actions on the real economy and price level, since the monetary transmission mechanism is characterised by long, variable, and uncertain time lags. In this regard, analysing the nexus between financial structure and monetary policy impulses empirically is crucial to understand how the various financial indicators contribute to monetary policy effectiveness in influencing economic activity. Financial sector inefficiencies are predicted to play a crucial role in the monetary policy transmission mechanism by amplifying and propagating the influence of monetary policy on the real economy (Bernanke, Gertler & Gilchrist 1999; Gertler & Gilchrist 1993).
However, due to a lack of clear understanding of such mechanisms in developing countries, there is a need to study the nexus between financial structure and monetary policy impulses in SSA.
Research objectives
The key objective is to investigate the nexus between financial structure and monetary policy impulses in SSA. The specific objectives are to:
- Examine the significance of the degree of banking sector competition on monetary policy impulses.
- Examine the impact of the level of financial sector development on monetary policy transmission to economic activity.
- Determine how the size of the central bank influences the impact of monetary policy on the real economy.
Hypotheses
The article is based on the following hypotheses:
H0: There is no significant impact of the degree of banking sector competition on monetary policy impulses.
H1: There is a significant impact of the degree of banking sector competition on monetary policy impulses.
H0: The level of financial sector development does not have a significant impact on the monetary policy transmission to economic activity.
H1: The level of the financial sector development has a significant impact on the monetary policy transmission to economic activity.
H0: The size of the central bank does not have a significant influence on monetary policy impulses.
H1: The size of the central bank has a significant influence on monetary policy impulses.
Empirical literature
The debate over the relationship between financial structure and monetary policy impulses has raged for a long time, and has piqued the interest of academics in both rich and developing countries. Effiong et al. (2017) carried out an investigation to check if financial development has an influence on monetary policy effectiveness in Africa. Panel data techniques were employed on annual data covering 39 African countries spanning the period from 1990 to 2015. The analysis discovered a weak link between the efficiency of monetary policy and financial development. In the case of inflation, a negative association was discovered, but only at their contemporaneous levels. The research suggested that deepening financial sector growth is necessary to boost the monetary transmission mechanism in African economies. Most African countries’ financial systems are claimed to be underdeveloped, having structural flaws that undermine the efficiency of monetary policy.
Mishra and Montiel (2012) reviewed the monetary transmission mechanism in low-income nations, with focus on the implications of financial market structure on the monetary transmission. With a small number of macro-economic variables such as interest rates, inflation, and output, the researchers employed variance decomposition and the impulse response functions (IRFs), based on VAR, to analyse the monetary transmission mechanism. According to the article, transmission channels are very country-specific and heavily dependent on each economy’s financial structure. Also, a wide range of empirical methodologies were said to have failed to produce evidence of successful monetary transmission in developing nations. The bank lending channel was determined to be the most common and dominant means of monetary transmission.
Carranza, Galdon-Sanchez and Gomez-Biscarri (2010) analysed the relationship between financial development and monetary policy by estimating a set of dynamic panels that test the significance of relationships between the indicators of financial development and monetary policy effectiveness. Factor analysis was used to obtain meaningful measures of financial development and several VAR-based measures of the effectiveness of monetary policy across several developed and developing nations. However, the researchers failed to rationalise either a positive or negative relationship between financial development and monetary policy effectiveness. The theoretical arguments were suggested to rationalise both a positive and a negative relationship. The conclusion was that monetary policy has a greater influence when the financial system is underdeveloped. The size of the central bank was found to be positively related to the effectiveness of monetary policy. However, the results were presented in the form of a list of stylised facts which require further attention.
Assenmacher-Wesche and Gerlach (2008) studied the effects of monetary policy shocks on residential property and equity prices, inflation, and economic activity. The researchers used single-country VARs and panel VARs to assess the nature of the responses, which were based on a panel of 17 countries for the period from 1986 to 2007. The findings show that monetary policy has significant and predictable effects on residential property values, which are approximately in line with its effects on the real economy. According to the paper, monetary policy could be utilised to tamp down property price booms. However, the use of the VAR methodology alone has drawbacks in terms of providing efficient estimates since the VAR model is not based on any economic theory and use of wrong lag lengths has dire consequences such as biased estimates.
Ferreira (2009) confirmed the relevance of the tendencies in the financial system in the European Union’s (EU) credit channel of monetary policy. For the period from 1999 to 2006, the researcher employed panel fixed-effect estimation using quarterly data from 26 EU countries. The importance of financial systems was examined, using the Bernanke and Blinder (1988) model. According to the article’s findings, bank performance conditions are particularly important for the expansion of bank lending in the European Union.
Ncube (2008) examined the importance of the financial system in the evolution and conduct of monetary policy in Africa where capital markets, non-banking financial institutions, and the banking system make up the financial system. According to their study, the effectiveness and operation of indirect monetary policy are influenced by the financial structure. The extent of competition between banks and non-bank financial firms, the balance sheet structure of financial and non-financial institutions, and the regulatory environment were suggested as the financial system’s main features that may influence the operation of monetary policy. The researcher established that the banking system’s structure, notably the lack of competition between banks, hinders the transmission mechanism, causing lending and deposits to respond slowly to changes in monetary policy stance. Furthermore, the impact of financial structure on monetary policy impulses varies greatly across countries. However, the findings from this article are not sufficient to warrant that the financial structure inhibits monetary policy transmission since it is purely narrative.
Zakaria (2007) specifically investigated whether monetary policy actions hinge on the countries’ financial structure for both the common-law countries and civil-law countries. A VAR model was estimated for each country by employing quarterly data for the period from 1980 to 2003. In civil-law nations, the effect of an increase in the rate of interest on output was found to be higher and to respond faster than in common-law nations. This was attributed to the fact that most financial arrangements in common-law countries are more market-based. The study concluded that in common-law nations, the greater the availability of substitutes for bank loans, the lesser the contractionary effect of monetary policy following a reduction in credit supply.
Conceptual framework
In this article a quantitative analytical framework is adopted to investigate the nature of the relationship between financial structure and monetary policy in SSA. The nonstandard approach to modelling will be adopted to facilitate aggregation and permit an endogenous financial structure. Figure 2 shows two possible links between monetary policy and the financial system as discovered in the literature. Link 1 has been widely discussed in the literature. It follows that monetary policy influences the financial system directly and indirectly through regulation of the financial markets and that the central bank may also participate in the financial market. However, link 2 is still questionable as empirical literature on this is scanty and inconclusive.
 |
FIGURE 2: Link between monetary policy transmission and the financial system. |
|
Figure 2 entails that financial market pricing reflects market expectations about future economic events such as output, inflation, and path of monetary policy, to the extent that monetary policy influences financial market activity. Financial contracts and institutions are, according to Bernanke et al. (1999), endogenous, and outcomes based on arbitrary constraints on financial ties may be misleading. Theoretical work on the impact of financial structure on economic fluctuations has largely focused on the asymmetric information that exists in the financial markets. Theoretical models highlight that credit in general, not simply bank lending, plays a role in the monetary transmission process.
According to Gertler and Gilchrist (1994), credit market frictions may aid in the business cycle propagation, and they may also play a key part in the transmission of monetary policy. According to a broader credit perspective of the monetary transmission mechanism, credit market flaws could play a role in the spread of both real and monetary shocks in the macro-economy, even if the monetary authorities may fail to directly regulate the bank credit flow (Bernanke & Gertler 1989; Gertler & Gilchrist 1993). According to Bernanke and Gertler (1989), the consequences of economic shocks on borrowers’ cash flows are to amplify and propagate them.
Credit market crises, according to Bernanke et al. (1999), increase the real costs of issuing credit and lower the efficiency of the intermediation process, and these events may have far-reaching economic consequences. Credit markets help to transmit and exacerbate macro-economic shocks in this way (Bernanke et al. 1994). The key mechanism encompasses the link between ‘external finance premium’ and the net worth of a pool of potential borrowers. Standard models with information asymmetry within the lending markets imply that the external finance premium depends negatively on the net worth of borrowers in the presence of credit-market imperfections, while holding the total amount of required funding constant. The negative association emerges as borrowers face limited finance for projects; the possibility of a conflict of interest between the borrower and external fund providers is greater, resulting in agency costs. Lenders must be paid for higher agency costs by a higher premium in order to achieve equilibrium. The external finance premium will be countercyclical, whereas the net worth of borrowers is pro-cyclical. This amplifies borrowing fluctuations, which in turn amplifies swings in investment, expenditure, and production. In accordance with the new Keynesian paradigm, Bernanke et al. (1999) integrated price-stickiness, as well as money into the analysis. This allowed the researchers to study the effects of monetary policy in an economy with credit market frictions.
Empirical model specification
A panel data model which is meant to relate a measure of monetary policy impulses (MPI) to financial structure (FS) will be estimated, while at the same time controlling for macro-economic heterogeneity. The dynamic panel data model (DPD) takes the form specified by Carranza et al. (2010) when analysing the relationship between financial development and monetary policy (MP) (see Equation 1):
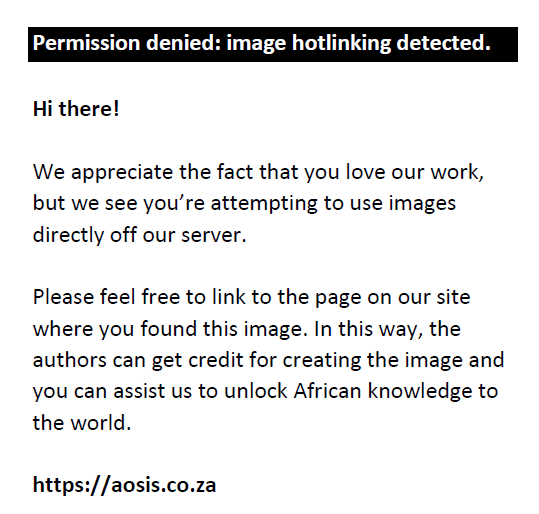
where mpiit is the specific MPI measure for country i in year t, FSj,it is the value of the FS factor j for country i in year t, zit is a vector of control variables and εit is an independently and identically distributed (i.i.d) error. The study employs Arellano and Bond’s (AB) (1991) General Method of Moments (GMM) estimator for the DPD model in which the measure of mpi is a continuous variable. The AB estimator is chosen because it takes all the orthogonality conditions into account and addresses the endogeneity problem through the use of instrumental variables.
The GMM is employed in this study since the dependent variable (MPI) is assumed to be dynamic and depends on its realisation in the past. Also, the right-hand variables (FS indicators such as competition in the banking industry) are considered to be not strictly exogenous (Hildebrand 2006). As already mentioned, financial market pricing reflects market expectations about future economic events such as output, inflation, and the future path of MP, to the extent that financial market activities influence MP. Financial contracts and institutions, according to Bernanke et al. (1999), are endogenous, and outcomes based on arbitrary constraints on financial ties may be misleading. Also, the panel dataset consists of a small number of time periods (2011–2018) and many individual units (21 SSA countries). Therefore, the AB estimator is suitable to estimate the functional relationship in Equation 1.
Definition and measurement of variables
Dependent variable
Monetary policy impulses
Monetary policy impulses are shocks transmitted to influence the real economy. To measure monetary policy impulses, previous empirical literature has mostly utilized VAR analysis (Carranza et al. 2010; Christiano, Eichenbaum & Evans 1999, 2005), or structural macro-econometric models (Boivin & Giannoni 2006; Fair 2005). The VAR model is advantageous over structural macro-economic models, because the estimates from the standard VAR model are flexible and less demanding in both information and time. Following the previous studies that were based on the VAR model and also incorporating information on prices, output, and a monetary policy instrument, this article builds a measure of monetary policy impulses.
The output of the country-specific VAR models will be used to compute the time it takes for changes in the monetary policy instrument to have a substantial influence on output, as well as the intensity of the impact. The article defines a reduced-form VAR model as follows (see Equation 2):

where . Y1t represents the output growth rate; MPt represents the monetary policy instrument (such as reserve money supply growth); and Y2t represents the inflation rate. The structural shocks are identified in a ‘block-Cholesky’ fashion and the impulses extracted in eight steps assigned to the years from 2011 to 2018. The final collection of the IRFs is carefully chosen to overlap with those for which an appropriate measure of monetary policy impulses can be obtained. The article collected data on output growth, reserve growth, inflation (consumer prices), inflation (GDP deflator) and broad money (% of GDP) from 1985 to 2018. These variables are included in a three-variable VAR model which consists of output growth, reserve growth (or broad money) as a monetary policy instrument, and inflation, consumer prices (or inflation, GDP deflator). The broad money (% of GDP) and inflation (GDP deflator) are used in cross-sectional units (countries) in which data on reserves and inflation (consumer prices) are not available. The problem of data unavailability in some SSA countries prompted the use of the VAR methodology as it is not based on any economic theory regarding the linkages between the output, reserve money growth, and inflation.
In line with Bernanke et al. (1999), the monetary policy instrument is ordered after the output growth rate, and the inflation rate after the monetary policy instrument to identify the policy shock, based on the notion that monetary policy responds contemporaneously to these variables but could affect them only with a lag. The article followed a recursive process in order to obtain a time series of annual values of the mpi measures which were then used along with the annual data on markcon, finsecsiz, findev, exchratereg, and centrbanksiz. The VARs are run for each country using at least 30 observations. The monetary policy impulses are then computed and assigned to year t, where t is the year that corresponds to each step under the IRFs. Moreover, the VAR model is estimated for each country, and tests for autocorrelation, as well as stability, are conducted. Impulses were extracted only after the specified VAR model has satisfied the stability condition; that is, when the eigenvalues all fall within the unit circle. An appropriate summation of the IRFs yields the responses for GDP to the variables; reserve growth (broad money) and inflation.
Independent variables
Financial structure (Core variables)
The level of the banking system competition was proxied by the number of branches per 100 000 inhabitants (Cotarelli & Kourelis 1994) which measures the degree of concentration in the banking system. Commercial bank branches, according to the World Bank (2021), are retail sites of resident commercial banks and other banks that provide banking services to consumers and are physically isolated from the main office, but not legally organised as independent corporations. The coefficient of competition in the banking system (markcon) is expected to have a negative sign and significant effect on monetary policy transmission since the financial market structure is characterised by oligopolistic competition in SSA.
The size of the financial sector (finsecsiz) is proxied by the domestic credit to the private sector by banks as a percentage of GDP. Financial resources availed to the private sector by depository corporations is the domestic credit to private sector by banks through loans, excluding those from the central banks, and trade credits and other accounts receivable, as well as purchases of non-equity securities that establish a claim for repayment (World Bank 2021). For quite a number of nations, these claims include credit sums extended to public corporations. The coefficient of finsecsiz is expected to have a positive sign, because as the financial system expands, its ability to allocate credit to the private sector in an efficient manner increases; and it helps stimulate investment and aggregate economic activity. According to Coats and Khatkhate (1979), a majority of the less developed countries lead relatively small financial systems in terms of size and capitalisation, whereas this is equally true of the countries stated in section 3.4.
According to Mishkin and Butkiewicz (2004), financial development (findev) indicators include advancements in the production of information regarding potential investments and efficiency in capital allocation, monitoring borrowers and exercising corporate governance. Also, improvements in financial assets trading, risk diversification and management, pooling and mobilisation of savings, and facilitation of the easy exchange of goods and services encompass financial development. In this regard, financial development is a broad term that involves quite a number of financial system indicators. The net acquisition of financial assets, as a percentage of GDP, is used to measure the development of the financial sector, while findev is expected to have a negative and significant influence on monetary policy. According to Ma and Lin (2016), the effectiveness of monetary policy declines when the financial system develops as new technologies and financial products are developed to guard against unanticipated policy changes. Financial systems in SSA are relatively underdeveloped even by the standards of developing countries in other regions such as Asia. However, South Africa leads in terms of financial development in SSA.
A central bank is a financial entity in charge of monitoring a country’s or group of countries’ monetary system and policy, as well as controlling the supply of money and setting the rates of interest. The research made use of the ratio of bank liquid reserves to bank assets as a proxy for the size of the central bank (centrbanksiz). The ratio of domestic currency holdings and deposits with the central bank to claims on other governments, the private sector, non-financial public businesses, and other banking institutions is known as the bank liquid reserves to bank assets ratio (World Bank 2021). Size of the central bank is expected to have a positive influence on the monetary policy responses, since a higher increase in central bank’s liquid reserves relative to the central bank’s assets necessitates the central bank to pursue its objectives effectively and enhance monetary policy effectiveness (Carranza et al. 2010). Central Banks in SSA, except the South African Reserve Bank and the Bank of Nigeria, are relatively small with regards to the assets they hold, as well as the liquid reserves. This is a potential factor contributing to the monetary policy potency in the region.
Control variable(s)
Exchange rate regime (exchratereg): This is a binary indicator that captures the exchange rate system of a country in a specific period. The variable takes two values; 1 if managed floating, and 0 otherwise. An exchange rate regime refers to the way a monetary authority controls its own currency relative to other denominations in the foreign exchange market. Monetary policy is said to be ineffective under a fixed exchange rate regime and more effective under a flexible exchange rate regime (Obstfeld 2001). Therefore, the coefficient of exchratereg is expected to be positive and significant, since a managed floating exchange rate regime is considered to be ideal for effective monetary policy rather than the fixed exchange rate regime. The exchange rate regimes in SSA differ by region, country and circumstances concerning the respective currency of trade.
Data and estimation procedure
Data sources
The econometric analysis is based on annual data observations of a number of selected macro-economic variables: output growth, inflation, reserves, broad money, and FS indicators, including the number of bank branches per 100 000 inhabitants, net acquisition of financial assets, and the bank liquid reserves to bank assets ratio. Therefore, a sample of 21 SSA countries was analysed, for which data observations on the mentioned variables were collected during the period spanning from 1985 to 2018 for the VAR model and the period from 2011 to 2018 for the panel data model. The data for each individual country were collected from the World Development Indicators database. Also, some missing data were collected from the respective countries’ central banks. The sub-Saharan African countries included for investigation were: Angola, Botswana, Burkina Faso, Cameroon, Congo Republic, Cote d’Ivoire, Equatorial Guinea, Ghana, Kenya, Lesotho, Madagascar, Mauritius, Namibia, Nigeria, Rwanda, Seychelles, South Africa, Tanzania, Uganda, Zambia, and Zimbabwe. These countries, and the time periods under consideration, were selected on the basis of the availability of reliable and comprehensive macro-economic data. The STATA Software package was used to analyse the data.
Estimation procedure
The data analysis was carried out in stages following the methodology outlined in this section.
Vector autoregressive model
The VAR model is a strategy for modelling endogenous variables by expressing them in relation to their own realisations previously, as well as the past values of other endogenous factors in the system. For illustration, a three-dimensional country-specific VAR model, specified in Equation 3, was considered:
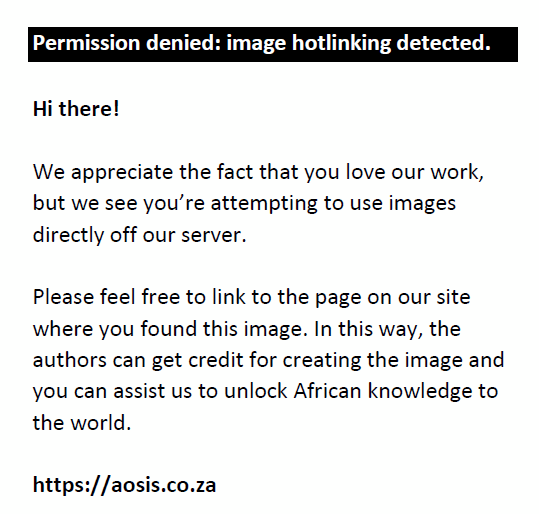
where Y1t is output, MPt is the monetary policy instrument and Y2t is the inflation rate. In line with Enders (2014), it was assumed that Y1t, MPt and Y2t are stationery, and the error terms (εit for i = 1,2,3) are uncorrelated white noise disturbances. Before approximation, VAR appropriateness was tested to avoid the problem of a spurious regression and the following situations were checked: stationarity, stability, lag length, and residual serial correlation: Lagrange Multiplier (LM) test.
Stationarity: When working with time-series data, this is one of the most significant concepts one encounters. Time-series analysis necessitates a lot of focus and investigation, as well as a stationary stochastic process. According to Gujarati (2009), if the mean of a time series is constant, the variance is finite, and the value of the covariance (or auto-covariance) between two time periods depends only on the distance or lag between these periods, not the actual time at which the covariance is computed, the time series is covariance (weakly) stationary. A stationary process is a ‘white noise’ process. Non-stationarity has been found to be very common in macro-economic and financial time series, hence the Dickey-Fuller test will be employed to check for non-stationarity among the variables included in the VAR model. The Dickey-Fuller test is carried out as follows:
H0: δ = 0 or ρ = 1 or the series are nonstationary
H1: δ ≠ 0 or the series are stationary
If the calculated , then we reject the null hypothesis, H0, and conclude that at the α level of significance test, we have enough support of statistical evidence that the series are stationary. Note that α is either at 1% or 5% or 10% level of significance test. The nonstationary variables will be differenced.
Stability: Are the polynomial’s roots contained within the unit circle? The roots of the polynomial defined in Equation 5 must be within the minus one and plus one range (-1; 1) for the VAR model to satisfy the stability criteria. As parameters are required to remain consistent over time, the stability condition is critical. The null hypothesis that the VAR model is stable, will be rejected when at least one of the roots of the polynomial lies outside the unit circle.
Lag length: In the first step, it will be assumed that all the series in the VAR model have equal lag lengths. If some of the VAR equations have regressors not included in others, seemingly unrelated regressions (SUR) provide efficient estimates of the VAR coefficients. Hence, when there is a good reason to let lag lengths differ across equations, the so-called near-VAR using SUR is estimated. To determine the number of lags that should be included, criterion functions can be utilised. The study will select the one with fewer lags between the Akaike Information Criterion (AIC) and the Schwarz Information Criterion (SC). The difference between these two approaches is that the SC will always select a more parsimonious model than the AIC.
Residual serial correlation: Lagrange Multiplier test: Autocorrelation refers to the correlation of a time series with its own previous and future values (Gujarati 2009). The LM test will be employed to check for correlation in the residuals. However, autocorrelation functions are also useful, as well as plotting residuals (Enders 2014). The existence of autocorrelation in the residuals of a model is a sign that the model may be unsound. The LM test is carried out as follows:
H0: There is no autocorrelation
H1: There is autocorrelation
The null hypothesis is rejected if the p-value is less than α Note that α is either at 1%, 5% and 10% level of significance. Autocorrelation will be corrected through the correct use of lags in the VAR model.
However, even if the VAR model fulfills the given situations, the model is problematic in that it is not established in relation to any economic theory. Therefore, the obtained coefficients of the VAR model are challenging to interpret, as they totally lack theoretical foundations. Since the individual coefficients in the estimated VAR models are often challenging to interpret, the specialists on this method often approximate the so-called IRFs. According to Enders (2014), the IRFs traces out the relation of the dependent variable in the VAR system to shocks in the disturbance terms, and traces out the impact of such tremors for a number of time periods in the future. Anticipating the impact of shocks for a number of periods ahead, gives the VAR methodology wide use in monetary policy analysis.
Impulse response functions
Considering a VAR(p) model similar to that defined in Equation 3 except for the dimension (see Equation 4):

According to Enders (2014), in the condition that the stationarity conditions hold, we have similar to the univariate case, the Vector Moving Average (VMA) representation of Zt as (see Equation 5):

The stochastic disturbances term, εt represent system shocks. The VMA representation permits one to follow the path of several shocks on the endogenous variables included in the VAR across time. It examines the interface between the {Yt} and {MPt} sequences in Equation 2. Suppose there is a shock in εt, then its impact in Z, s periods in the future is (see Equation 6):

Accordingly, the θ matrices symbolise the marginal effects, or the model’s sensitivity to an innovation in each of the included variables at some point in time t. The sensitivity of zi to a zj unit shock is sequential and this sequence is referred to as the IRF (see Equation 7):

where θij, k is the ij-th element of the matrix θk (i,j= 1,..m). θij coefficients can be used to obtain the marginal effects of εYt and εMPt shocks on the entire time paths of {Yt} and {MPt} sequences. The combined effects of unit impulses can be generated thorough the addition of the coefficients of the IRF. Thus, an IRF tracks the influence of a one standard deviation shock to one of the innovations on future values of the endogenous factors (Enders 2014). A tremor to the j-th variable straightly affects the j-th variable, and is also spread to all the endogenous variables through the VAR. In calculating the impulse responses, the order of the included variables is vital. This is so because impulse responses refer to a unit shock to the errors of one VAR equation alone.
Stationarity and co-integration tests in panel data
The study adopted the Levin, Lin and Chu’s (LLC) (2002) test which is built under the notion that the time series in the panel are cross-sectionally independently distributed. However, contradictory literature indicates proof of the co-movements between economic variables. Assuming that the time series {finsecsizi0,…,finsecsiziT}, on the cross-section units i = 1,2,…,N are generated for each i by a simple first-order autoregressive, AR(1), process (see Equation 8):

where finsecsiz represents financial sector size, the first values, finsecsizi0, are already given, and the disturbances terms, εit are i.i.d across i and t with E(εit) = 0, , and . Processes like these can also be easily expressed as Dickey-Fuller (DF) regressions as follows (see Equation 9):

The null hypothesis is that all time series among the panels contain unit roots and under the alternative hypothesis, the AR parameter is the same for all units cross-sectionally:
H0: ∅1 = … =∅N = 0 against two alternatives;
H1a: ∅1 = … =∅N ≡ ∅ and ∅ < 0; and
H1b: ∅1 < 0, …, ∅N0 < 0,N0 < N
H1a is the homogeneous alternative while H1b is referred to as the heterogeneous alternative (Im, Pesaran & Shin 2003). The LLC was used to check for non-stationarity in the panel data. In addition to stationarity tests, the Kao (1999) co-integration test was employed to check if there exists a long-run equilibrium relationship among the panels. In the Kao’s test for co-integration, the null hypothesis of no co-integration is rejected if the p-value from the calculated Dickey-Fuller statistic is less than α (1%, 5% and 10% level of significance) and the conclusion will be that there exists a long-run equilibrium relationship between the variables under consideration.
The Arellano and Bond general method of moments
The AB estimator is an extension of the Anderson and Hsiao (AH) (1982) estimator as Arellano and Bond (1991) argued that the AH estimator, while consistent, fails to take all of the possible orthogonality conditions into account. According to Baltagi (2008), a key feature of the AB strategy, resonating that of AH, is assuming that the essential instruments are ‘internal’ and constructed from lagged values of the instrumented variable(s). The estimators also permit the addition of outside instruments into the model (Baltagi 2008). Consider Equation 10 and Equation 11:
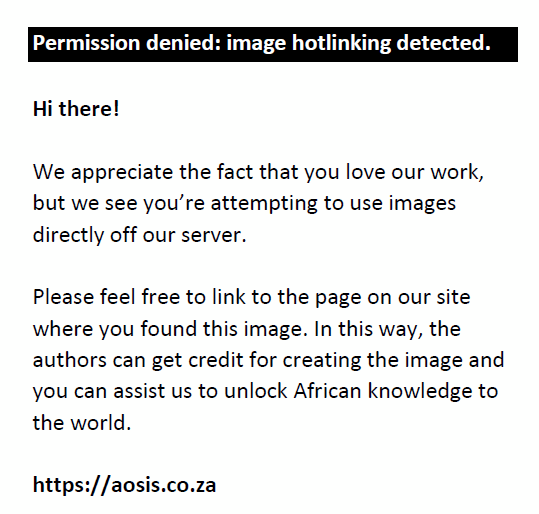

where: Xit includes strictly exogenous regressors such as the size of the central bank, Wit are predetermined regressors (which may include lags of mpi, the monetary policy impulses) and endogenous regressors (the degree of competition within the banking system, financial development and the size of the central bank), all of which may be correlated with μi, the unobserved individual effect. First-differencing the equation removes the μi and its associated omitted-variable bias. According to Baltagi (2008), the AB (1991) approach, and its extension to the ‘system GMM’ context, is an estimator designed for conditions with:
- A linear functional relationship.
- One left-hand variable that is dynamic, depending on its own past realisations.
- ‘Small T, large N’ panels: few time periods (8 years) and many individual units (21 SSA countries).
- Fixed individual effects, implying unobserved heterogeneity.
- Right-hand variables that are not strictly exogenous: correlated with past and possibly current realisations of the error.
- Heteroscedasticity and autocorrelation within individual units’ errors, but not across them.
Since the AB estimator is based on an instrumental variables method, it is necessary to carry out the Sargan’s diagnostic test for over-identifying restrictions (Baltagi 2008). The Sargan test’s null hypothesis supporting the validity of the over-identifying restrictions is rejected if the p-value is less than α (at 1%, 5% and 10% level of significance) and thus, the over-identifying restrictions will not be imposed.
The system general method of moments
A possible limitation of the AB dynamic panel data estimator was discovered years later by Arellano and Bover (1995). The lagged levels are weak instruments for the integrated variables of order one, given that the variables are nearer to a unit root. Their adjustment of the estimator includes lagged levels, as well as lagged differences (Baltagi 2008). The system GMM estimator encompasses a set of extra constraints on the preliminary conditions of the process producing y. Consider the dynamic panel data model in Equation 12:
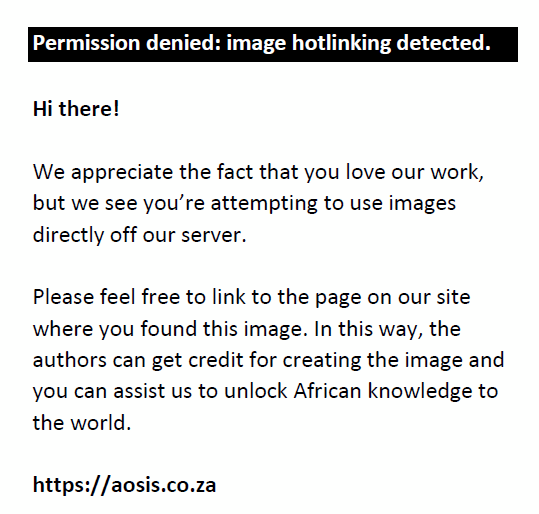
where: αi are the unobserved individual effects, xit is a vector of K1 time-varying explanatory variables, wi is a vector of K2 time-invariant variables. The model makes the following assumptions:
- E(εit) = 0, E(αi) = 0
- E(εit εjs) =
if j = i and t = s, 0 otherwise
- E(αi αj) =
if j = i, 0 otherwise
- E(αi xit) = 0, E(αi wi) = 0 (exogeneity assumption for wi)
The GMM estimation method is founded on a model with variables in first differences, so as to remove the individual effects αi and the variables wi. In this regard, both the AB and the Arellano and Bover method of moments are reliable. Thus, this study employed the Arellano and Bond GMM to estimate the coefficients on the dynamic panel data model in Equation 1 for 21 SSA countries.
Variables
The preliminary investigation steps involved employing country-specific VAR models to generate a reliable measure of monetary policy impulses. Table 2 shows the list of the 21 SSA countries and their averages over the period from 1985 to 2018 for each measure of inflation, reserve growth, output growth, and broad money as a percentage of GDP.
A cursory examination of the data exposes major disparities across countries among the variables under consideration. The rate of annual inflation ranged from an average of 2.696% for Burkina Faso and an average of 39.105% for Zambia. Also, the average annual growth in broad money as a percentage of GDP ranged from a record of 14.032% for Equatorial Guinea and a record of 84.151% for Mauritius among the cross-sectional units. The relatively large disparities in the observations suggest that monetary policy may have asymmetrical effects on prices and output across countries. As a result, the price and output reactions to an unexpected monetary policy shock vary significantly throughout SSA. Congo Republic has the lowest output growth rate average of 1.625%. However, we do not comment on the results from the VAR estimation for the separate countries. The VAR model was only used to estimate IRFs for each country in order to obtain a measure of monetary policy instrument. It should be highlighted that monetary policy in most of the countries under examination is often relatively short-term, and in some cases, even ineffective. The data to support this are presented in Appendix 1.
Descriptive statistics
The descriptive statistics were based on a panel consisting of 21 SSA countries and 8 time periods. Table 3 shows the mean, standard deviation, minimum and the maximum on each variable under consideration.
TABLE 3: Descriptive statistics for the overall, between and within. |
The ‘overall’ statistics are ordinary statistics that were based on 168 observations. ‘Between’ statistics are calculated on the basis of summary statistics of the 21 countries, regardless of the time period, while ‘within’ statistics by summary statistics of eight time periods regardless of the country. The variables mpi, finsecsiz, findev, and centrbanksiz have means of: -0.0275%, 25.4969%, 0.8732%, and 21.4764, respectively; exchratereg is a qualitative indicator taking two values: a minimum of zero and a maximum of one; whereas mpi has an overall minimum of 4.45%, while the average number of bank branches per 100 000 (marketcon) is 8.53 in SSA. The average number of bank branches indicates a low level of banking sector competition in SSA. Most countries in SSA have a fixed exchange-rate regime with a low variation in findev as compared to finsecsiz between groups. The average ratio of 21.48 for centrbanksiz indicates that central banks in SSA hold more reserves in relation to central bank assets.
Panel unit root and co-integration tests
The LLC’s (2002) test results are presented in Table 4. The STATA output is inserted in Appendix 1.
In all cases, the null hypothesis that the panels contain unit roots, is rejected and the conclusion is that all the panels are stationary. All the variables under consideration are stationary at the 1% level of significance, except centrbanksiz which is stationary at the 5% significance level. In addition to the stationarity tests, the Kao test for co-integration was conducted and results are presented in Appendix 1.
Findings
Empirical results
The results of the dynamic panel data model estimated using the AB estimator are presented in Table 5. The STATA output is also inserted in Appendix 2.
TABLE 5: Arellano-Bond estimation results. |
The Wald Chi-Square statistic is significant at the 1% level and this confirms that at least one of the coefficients in not equivalent to zero. The Sargan test’s null for the validity of over-identifying restrictions was rejected and the conclusion was that there was no need to impose over-identification restrictions. The results in Table 5 indicate that MPI in the previous two periods, as well as competition in the banking system in the previous period are significant at the 1% level (p-value < 0.001). Financial development is significant at the 5% level (p-value < 0.05), while the size of the central bank is economically and statistically significant at the 10% level (p-value < 0.10).
Interpretation of the results
Lagged monetary policy impulses
The negative coefficient of the monetary policy impulses in the previous two periods implies that the influence of monetary policy on economic activity vanishes as the economy moves from one period to another. A rise in the influence of monetary policy on economic activity in the previous two periods will result in a decline in the current period’s impulses by 0.62% in the leading period and by 0.27% in the subsequent period. Thus, it can be noted that monetary policy’s influence on economic activity is short-lasting in SSA. According to Brunner and Meltzer (1988), the form of transmission rests on the public’s opinion about the strength and resolution of various shocks to the macro-economy. The finding is in line with the priori information that monetary policy affects economic activity with a lag. According to Mankiw (2009), a change in the monetary policy stance is thought not to affect economic activity until about 6 months after it is made and the effects may persist up to 24 months. The results obtained, conform to this assertion.
Market concentration
The positive coefficient of markconc (L1) implies that an increase in the number of commercial bank branches per 100 000 people by one bank in the previous period will result in a 0.7% increase in the response of the economic activity to monetary policy in the current period. The result is in line with the expected results since a move towards a more competitive banking system, coupled with financial inclusion, is more likely to increase the responses of economic activity to monetary policy, as this will possibly accelerate credit expansion and reduce collusive tendencies by commercial banks when allocating financial resources. According to Mlachila et al. (2016), most countries in SSA have underdeveloped financial systems categorised by low competition and usually only a small number financial intermediaries. As competition within the banking system increases, commercial banks’ practices will respond better to market incentives and monetary policy becomes more effective. In line with Ncube (2008), lack of bank competition weakens the transmission mechanism resulting to the moderately sluggish correction of deposit and lending rates to alterations in the monetary policy stance.
Financial development
Monetary policy tends to have a significant cumulative impact on real economic activity when the financial system is less developed. The results indicate that a 1% rise in the net acquisition of financial assets (as a percentage of GDP) in the previous period will induce a 0.17% decline in the responsiveness of economic activity to monetary policy in the current period. As the financial system develops, private agents can devise new instruments such as crypto currency which allow them to safeguard against unpredicted monetary shocks and thus, reduce the volatility of their expenditure patterns (De Bondt 2000; Effiong et al. 2017). The finding confirms the expected results and the conclusion by Ma and Lin (2016) according to which the effects of monetary policy on output and inflation are negatively and significantly related to financial development.
Central bank size
The larger the central bank, the higher is the prospect that its actions will meaningfully affect economic agents’ actions and effectively influence economic activity in SSA. The coefficient of centrbanksiz indicates that an increase in the ratio of bank liquid reserves to bank assets, held by monetary authorities by 1%, will result in an increase in the cumulative impact of monetary policy on economic activity by 0.0448%. In conformity with the findings by Carranza et al. (2010), an increase in the size of the central bank results in an increase in the power of the central bank to conduct and implement effective monetary policy. The result is also in line with the expected results from the study.
Summary, conclusion and policy recommendations
In this article the nexus between financial structure and monetary policy impulses in SSA is analysed. It investigated a sample of 21 sub-Saharan African countries as certain characteristics work hand in hand to render SSA countries’ financial systems highly susceptible to both internal and external shocks. The way monetary policy is transmitted through the real economy is a debatable issue in academic and central bank research. Since the late 1990s, the net effect of financial market distortions has been to amplify monetary policy effectiveness in most of the typical emerging and advanced market economies. Empirical literature on the subject arrives at different conclusions, hence the rationale for conducting this study in SSA employing both the structural VAR model and the dynamic panel data model. The results show that competition in the banking system, financial development, and the size of the central bank have a significant impact on monetary policy impulses. Banking system competition and the size of the central bank both positively influence the operation of monetary policy while monetary policy impulses are inversely related to financial development.
Monetary policy has a short-lasting impact on economic activity in SSA. A move towards a more competitive banking system is more likely to increase the responses of economic activity to monetary policy, as this will accelerate credit expansion and lessen collusive behaviour among commercial banks when allocating financial resources. The article further concludes that monetary policy tends to have a significant cumulative impact on real economic activities when the financial system is less developed; and the larger the central bank, the higher is the possibility that its actions will significantly affect economic agents’ behaviour and effectively influence economic activity in SSA. Therefore, financial structure has a significant role to play in the monetary transmission mechanism.
Reforms targeted at enhancing the banking system competition should be implemented by the monetary authorities in SSA. A lack of stiff bank competition is an obstacle to a smooth transmission mechanism resulting in delayed response regarding lending and deposit rates to alterations in the monetary policy stance. Thus, as competition in the banking system increases, the behaviour of commercial banks will align itself increasingly to market incentives, and monetary policy may become more effective. The central banks in SSA must also closely monitor developments in the financial sector. Private economic agents could introduce new instruments such as cryptocurrency to safeguard them against unpredicted monetary policy shocks. In this regard, central bank policy on the acquisition of financial assets must keep in line with new developments in the financial market for monetary policy to remain effective. The size of the central bank is relevant to the conduct of monetary policy and, therefore, the central bank is advised to keep enough reserves and domestic currency holdings in order to effectively implement monetary policy.
Acknowledgements
Competing interests
The authors declare that they have no financial or personal relationships that may have inappropriately influenced them in writing this article.
Authors’ contributions
R.A-T. handled the theoretical portion of the article, while J.M. performed the regression analysis.
Ethical considerations
This article solely focuses on measuring the monetary policy impulses and the dynamic panel data model to examine the linear relationship, and it does not involve any activities that would necessitate ethical clearance related to human participants.
Funding information
The authors received no financial support for the research, authorship, and/or publication of this article.
Data availability
Data supporting the findings of this study are available from the corresponding author, J.M. on reasonable request.
Disclaimer
The views and opinions in this article are those of the authors and do not reflect the official policy of any agency of the authors, or the publisher.
References
Adelegan, O.J. & Radzewicz-Bak, B., 2009, What determines bond market development in sub-Saharan Africa? (No. 9-213), International Monetary Fund, Washington D.C.
Allen, F., Carletti, E., Cull, R., Qian, Q.J., Senbet, L.W. & Valenzuela, P., 2016, ‘Resolving the African financial development gap: Cross-country comparisons and a within-country study of Kenya’, in S. Edwards, S. Johnson & D. Weil (eds.), African successes: Modernization and development, NBER, pp. 1–10, University of Chicago Press, Chicago.
Allen, F., Otchere, I. & Senbet, L.W., 2011, ‘African financial systems: A review’, Review of Development Finance 1(2), 79–113. https://doi.org/10.1016/j.rdf.2011.03.003
Anderson, T.W. & Hsiao, C., 1982, ‘Formulation and estimation of dynamic models using panel data’, Journal of Econometrics 18(1), 47–82. https://doi.org/10.1016/0304-4076(82)90095-1
Arellano, M. & Bond, S., 1991, ‘Some tests of specification for panel data: Monte Carlo evidence and an application to employment equations’, The Review of Economic Studies 58(2), 277–297. https://doi.org/10.2307/2297968
Arellano, M. & Bover, O., 1995, ‘Another look at the instrumental variable estimation of error-components models’, Journal of Econometrics 68(1), 29–51. https://doi.org/10.1016/0304-4076(94)01642-D
Assenmacher-Wesche, K. & Gerlach, S., 2008, Financial structure and the impact of monetary policy on asset prices, Swiss National Bank, Zurich.
Baltagi, B., 2008, Econometric analysis of panel data, John Wiley & Sons, Wiley.
Bean, C.R., Larsen, J.D. & Nikolov, K., 2002, Financial frictions and the monetary transmission mechanism: Theory, evidence and policy implications, ECB Working Paper 113, European Central Bank, Frankfurt, viewed 28 November 2020, from https://econpapers.repec.org/paper/ecbecbwps/2002113.htm.
Bernanke, B. & Gertler, M., 1989, ‘Agency costs, net worth and business fluctuations’, in Business cycle theory, Edward Elgar Publishing Ltd., Cheltenham, viewed 26 January 2021, from https://econpapers.repec.org/scripts/redir.pf?u=https%3A%2F%2Fdoi.org%2F10.22004%252Fag.econ.292693;h=repec:ags:uwssri:292693.
Bernanke, B.S., 1993, ‘How important is the credit channel in the transmission of monetary policy?: A comment’, Carnegie-Rochester Conference Series on Public Policy 39(1), 47–52. https://doi.org/10.1016/0167-2231(93)90002-E
Bernanke, B.S. & Blinder, A.S., 1988, Credit, money, and aggregate demand (No. w2534), National Bureau of Economic Research, Massachusetts.
Bernanke, B.S. & Gertler, M. & Gilchrist, S., 1999, ‘The financial accelerator in a quantitative business cycle framework,’ in J.B. Taylor & M. Woodford (eds.), Handbook of Macroeconomics, 1st edn, vol. 1, pp. 1341–1393, Elsevier, National Bureau of Economic Research, Massachussettes.
Boivin, J. & Giannoni, M.P., 2006, ‘Has monetary policy become more effective?’, The Review of Economics and Statistics 88(3), 445–462. https://doi.org/10.1162/rest.88.3.445
Brunner, K. & Meltzer, A.H., 1988, ‘Money and credit in the monetary transmission process’, The American Economic Review 78(2), 446–451. http://www.jstor.org/stable/1818166
Carranza, L., Galdon-Sanchez, J.E. & Gomez-Biscarri, J., 2010, ‘Understanding the relationship between financial development and monetary policy’, Review of International Economics 18(5), 849–864. https://doi.org/10.1111/j.1467-9396.2010.00926.x
Christiano, L.J., Eichenbaum, M. & Evans, C.L., 1999, ‘Monetary policy shocks: What have we learned and to what end?’, in J.B. Taylor & M. Woodford (eds.), Handbook of Macroeconomics, 1st edn, vol. 1, pp. 65–148, Elsevier, National Bureau of Economic Research, Massachusetts.
Christiano, L.J., Eichenbaum, M. & Evans, C.L., 2005, ‘Nominal rigidities and the dynamic effects of a shock to monetary policy’, Journal of Political Economy 113(1), 1–45. https://doi.org/10.1086/426038
Coats, W.L., & Khatkhate, D.R., 1979, ‘Money and monetary policy in less developed countries: Survey of issues and evidence’, Economic and Political Weekly 14(46), 1881–1900.
Corker, R.J. & Tseng, W.S., 1991, Financial liberalization, money demand, and monetary policy in Asian Countries (No. 84), International Monetary Fund, Washington D.C.
Cotarelli, C. & Kourelis, A., 1994, ‘Financial structure, bank lending rates, and the transmission mechanism of monetary policy’, Staff Papers 41(4), 587–623. https://doi.org/10.2307/3867521
De Bondt, G.J., 2000, Financial structure and monetary transmission in Europe: A cross-country study, Edward Elgar Publishing, Cheltenham.
Effiong, E., Esu, G. & Chuku, C., 2017, Financial development and monetary policy effectiveness in Africa, MPRA Paper 79732., Cheltenham.
Enders, W., 2014, Applied econometric time series, 4th edn., John Wiley & Sons, Inc., New Jersey.
Fair, R.C., 2005, ‘Estimates of the effectiveness of monetary policy’, Journal of Money, Credit and Banking 37(4), 645–660. https://doi.org/10.1353/mcb.2005.0041
Ferreira, M.C., 2009, The credit channel transmission of monetary policy in the European Union, School of Economics and Management, Lisbon.
Gertler, M. & Gilchrist, S., 1993, ‘The role of credit market imperfections in the monetary transmission mechanism: Arguments and evidence’, The Scandinavian Journal of Economics 95(1), 43–64. https://doi.org/10.2307/3440134
Gertler, M. & Gilchrist, S., 1994, ‘Monetary policy, business cycles, and the behavior of small manufacturing firms’, The Quarterly Journal of Economics 109(2), 309–340. https://doi.org/10.2307/2118465
Green, C.J., 2013, Financial reform and financial development in Africa, Commissioned Proposal Prepared for AERC, African Economic Research Consortium, Nairobi.
Gujarati, D.N. & Porter, D., 2009, Basic econometrics, International edn., McGraw-Hill, Irvine.
Hildebrand, P.M., 2006, ‘Monetary policy and financial markets’, Financial Markets and Portfolio Management 20(1), 7–18. https://doi.org/10.1007/s11408-006-0004-8
Im, K.S., Pesaran, M.H. & Shin, Y., 2003, ‘Testing for unit roots in heterogeneous panels’, Journal of Econometrics 115(1), 53–74. https://doi.org/10.1016/S0304-4076(03)00092-7
Levin, A., Lin, C.F. & Chu, C.S.J., 2002, ‘Unit root tests in panel data: Asymptotic and finite-sample properties’, Journal of Econometrics 108(1), 1–24. https://doi.org/10.1016/S0304-4076(01)00098-7
Ma, Y. & Lin, X., 2016, ‘Financial development and the effectiveness of monetary policy’, Journal of Banking and Finance 68, 1–11. https://doi.org/10.1016/j.jbankfin.2016.03.002
Mankiw, N.G., 2009, Principles of macroeconomics, 7th edn., South-Western Cengage Learning, Mason.
Mishkin, F.S. & Butkiewicz, J.L., 2004, The economics of money, banking, and financial markets, Test Bank, Pearson.
Mishra, M.P. & Montiel, M.P., 2012, How effective is monetary transmission in low-income countries? A Survey of the Empirical Evidence, pp. 1–20, International Monetary Fund, Washington D.C.
Mlachila, M., Cui, L., Jidoud, A., Newiak, M., Radzewicz-Bak, B., Takebe, M. et al., 2016, Financial development in Sub-Saharan Africa: Promoting inclusive and sustainable growth (No. 16/11), International Monetary Fund, Washington, DC.
Ncube, M. (ed.), 2008, Financial systems and monetary policy in Africa, African Economic Research Consortium, Nairobi.
Obstfeld, M., 2001, International macroeconomics: Beyond the Mundell-Fleming model (No. w8369), National Bureau of Economic Research, Massachusetts.
Otchere, I., Senbet, L. & Simbanegavi, W., 2017, ‘Financial sector development in Africa – An overview’, Review of Development Finance 7(1), 1–5. https://doi.org/10.1016/j.rdf.2017.04.002
Rotberg, R., 2013, Africa emerges: Consummate challenges, abundant opportunities, Polity Press, Cambridge.
Senbet, L. & Otchere, I., 2010, African stock markets – Opportunities and issues in African finance in the 21st century, International Monetary Fund, Washington D.C.
World Bank, 2021, World development indicators, viewed 01 June 2021, from https://databank.worldbank.org/source/world-development-indicators#.
Zakaria, Z., 2007, ‘The performance of monetary policy: A comparison between civil-law and common-law countries’, Jurnal Kemanusiaan 5(1), 8–14.
Appendix 1
Stationarity and co-integration tests in panel data
Appendix 2
Empirical results
|